We utilize high-resolution satellite imagery and remote sensing to monitor cloud formations and wind speeds, leveraging advanced algorithms for early storm detection. Doppler radar provides velocity and intensity data of precipitation, enhancing our ability to forecast storm developments. Machine learning models analyze complex patterns, optimizing predictive accuracy through hyperparameter tuning. GIS integrates real-time data, creating dynamic, multi-dimensional models to spatially analyze storm movements. Collaborating with meteorological networks enhances data integration and enhances effective information exchange. By utilizing these techniques, we achieve precise storm tracking, greatly enhancing our ability to anticipate and respond to severe weather events. Learn more about each strategy's profound impact.
Key Points
- Utilize high-resolution satellite imagery to monitor and predict storm paths with advanced algorithms.
- Analyze Doppler radar data to track precipitation intensity and wind speed within storms.
- Implement machine learning models to identify complex storm patterns and enhance predictive accuracy.
- Leverage Geographic Information Systems (GIS) for spatial analysis and real-time visualization of storm movement.
Utilizing Satellite Imagery
By leveraging high-resolution satellite imagery, we can track storm paths with unprecedented accuracy and detail. Satellite technology allows us to observe atmospheric conditions from space, providing critical data for weather forecasting. With remote sensing capabilities, we can monitor cloud formations, wind speeds, and precipitation patterns in real-time, enabling more precise storm prediction.
Incorporating satellite imagery into our analytical models, we employ advanced algorithms to interpret vast amounts of data. These algorithms help us detect early signs of storm development, track their movements, and predict potential landfall locations. For instance, geostationary satellites offer continuous coverage of specific regions, while polar-orbiting satellites provide global snapshots. This dual approach enhances our ability to predict storms over both short and long-term periods.
Utilizing these satellite resources, we gain a detailed understanding of storm dynamics. The high-resolution imagery reveals the intricate details of storm structures, including eye formation in hurricanes and the delineation of storm fronts.
Analyzing Doppler Radar Data
Analyzing Doppler radar data enables us to measure the velocity and intensity of precipitation within storm systems, providing critical insights into their behavior and potential impact. By capturing real-time data, we can dissect storm patterns and undertake precise data analysis to predict the storm's trajectory and severity. This capability is essential for enhancing our weather forecasting methods and refining predictive models.
We begin by examining the radar reflectivity values, which indicate precipitation intensity. Higher reflectivity values typically suggest heavier rainfall, while velocity data reveals wind speed and direction within the storm. By integrating these metrics, we identify rotational patterns indicative of severe weather phenomena, such as tornadoes or hurricanes.
Furthermore, Doppler radar data allows us to monitor the storm's development over time, offering a dynamic view of storm patterns. We can track the evolution of storm cells, detect potential flooding events, and issue timely warnings, thereby safeguarding communities. By utilizing sophisticated data analysis techniques, we improve the accuracy of our weather forecasting and predictive models, empowering us to make informed decisions.
In essence, Doppler radar data is a cornerstone in our arsenal for tracking and analyzing storm systems, equipping us with the knowledge needed to anticipate and mitigate their impacts.
Implementing Machine Learning Models
To enhance our predictive capabilities, we leverage machine learning models to analyze vast datasets and identify complex patterns within storm systems. Our approach starts with rigorous data preprocessing, ensuring that our input data is clean, normalized, and free from inconsistencies. By addressing missing values and outliers, we prepare our data for more accurate modeling.
Once the data is preprocessed, we focus on model evaluation to choose the most effective algorithms. We typically compare a range of models like Random Forest, Gradient Boosting, and Neural Networks. Each model is assessed based on metrics such as Mean Absolute Error (MAE) and Root Mean Square Error (RMSE) to determine its predictive accuracy.
Hyperparameter tuning is another critical step in our process. By optimizing parameters like learning rate, depth of trees, and the number of layers, we can greatly enhance the performance of our models. Techniques such as Grid Search and Random Search allow us to systematically explore a wide range of hyperparameters.
Ultimately, our goal is to achieve the highest predictive accuracy possible. This data-driven and analytical approach empowers us to provide more reliable storm tracking, enhancing public safety and giving individuals the freedom to make informed decisions.
Leveraging Geographic Information Systems
Incorporating Geographic Information Systems (GIS) into our storm tracking methodology enables us to spatially analyze and visualize the intricate movement patterns of storm systems. By utilizing GIS applications, we can overlay various data layers, such as topography, land use, and historical storm paths, to enhance our understanding of how weather patterns evolve and interact with the environment.
Geospatial technology provides us with the tools to integrate real-time data from satellites, radar, and ground-based sensors. This integration allows us to create dynamic, multi-dimensional models of storm systems, offering a thorough view of their trajectories. By mapping these models, we can identify potential impact zones and generate predictive analytics that inform emergency response strategies and public safety measures.
Furthermore, GIS applications in storm tracking facilitate the analysis of spatial-temporal patterns. This capability is essential for identifying recurring phenomena and anomalies in weather patterns, which can improve the accuracy of our forecasts. Leveraging GIS, we can empower communities to prepare and respond more effectively to storm threats, ensuring both their safety and autonomy in decision-making.
In essence, the synergy between GIS and storm tracking equips us with advanced analytical tools, fostering a proactive approach to managing and mitigating the impacts of severe weather events.
Collaborating With Meteorological Networks
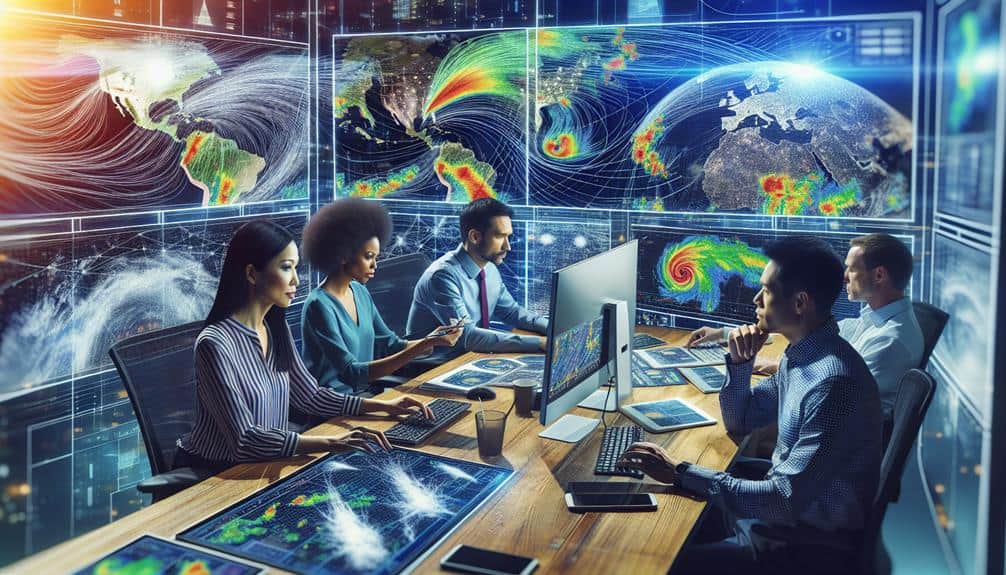
Frequently, we collaborate with meteorological networks to pool data and expertise, enhancing our storm tracking accuracy and predictive capabilities. By integrating data from various sources, we can achieve a more thorough understanding of storm dynamics. This data integration involves real-time monitoring from satellite feeds, radar systems, and ground-based weather stations, allowing us to refine our models continuously.
Effective communication protocols are essential in this collaborative environment. We establish standardized data formats and transmission methods to guarantee seamless information exchange between different meteorological networks. This not only optimizes our analysis but also speeds up the dissemination of vital information to relevant stakeholders.
In emergency response scenarios, timely and accurate data is paramount. By working closely with meteorological networks, we can provide real-time updates on storm progression, enabling emergency services to make informed decisions and deploy resources efficiently. This collaborative approach empowers communities by providing them with the information they need to prepare and respond effectively, ultimately safeguarding lives and property.
Our collective efforts in data integration and real-time monitoring, combined with robust communication protocols, notably enhance our ability to track storm paths and support emergency response initiatives. This synergy fosters a proactive stance in weather-related disaster management, offering freedom through preparedness.
Frequently Asked Questions
How Do Historical Weather Patterns Affect Storm Path Predictions?
When we analyze historical weather patterns, we better understand climate change impact on storm forecasting. Geographical influences also play a pivotal role, enhancing storm tracking accuracy. This data-driven approach empowers us to improve predictive models and guarantee preparedness.
What Role Do Ocean Temperatures Play in Storm Development?
Ocean temperatures are essential in storm development. Warmer waters, influenced by climate change and ocean currents, can increase storm intensity and frequency, making predictive models crucial for understanding and mitigating future impacts.
How Can Smartphone Apps Help Track Storms?
We can't live without smartphone apps! They provide real-time updates and GPS accuracy, letting us track storms like never before. We're empowered with precise data, ensuring safety and freedom in even the most unpredictable weather conditions.
What Are the Limitations of Current Storm Tracking Technologies?
The limitations of current storm tracking technologies include data accuracy and tracking consistency. We often encounter discrepancies in real-time data, making it challenging to predict storm paths accurately and maintain consistent tracking for timely alerts.
How Do Altitude Variations Impact Storm Movement?
Altitude variations impact storm movement by altering wind patterns and air pressure. Higher altitudes often mean stronger winds and lower pressure, which can accelerate or redirect storms, making tracking more complex but offering opportunities for precise predictions.