We leverage advanced methods like satellite remote sensing and Doppler radar technology to predict storms with remarkable precision. Satellites help us monitor atmospheric conditions and storm evolution in real-time, while Doppler radar measures wind speed and storm structure. High-resolution weather models simulate small-scale atmospheric processes, and data assimilation techniques integrate observational data for enhanced forecasts. Additionally, analyzing social media data provides on-the-ground observations. These cutting-edge techniques guarantee precise, timely storm predictions, so if you're interested in a deeper understanding, there's much more to explore.
Key Points
- Satellite remote sensing enables accurate real-time monitoring and prediction of storm behavior.
- Doppler radar technology provides detailed storm structures and velocity data for predictive models.
- High-resolution models simulate atmospheric processes with finer grid spacing for improved storm forecasts.
- Improved data assimilation techniques integrate diverse real-time data into predictive models.
Satellite Remote Sensing
Satellite remote sensing is a vital tool that enables us to monitor and predict storm behavior with unparalleled accuracy. By leveraging advanced satellite technology, we can conduct detailed cloud cover analysis and atmospheric monitoring. This allows us to track the formation and evolution of storm systems in real-time. The precision of satellite imagery helps us understand the dynamics of cloud structures and temperature variations within the atmosphere, essential for anticipating storm development.
In addition, satellite remote sensing excels in precipitation detection. High-resolution sensors measure the intensity and distribution of rainfall, providing necessary data for storm tracking. This data helps meteorologists pinpoint areas of heavy precipitation and potential flooding, enhancing our ability to issue timely warnings. We can analyze changes in precipitation patterns, aiding in the prediction of a storm's path and intensity.
The integration of satellite data with other meteorological tools enables a thorough approach to storm prediction. We can correlate cloud cover analysis with atmospheric pressure systems and moisture content, offering a holistic view of storm dynamics. This multi-faceted analysis empowers us to make informed decisions, ultimately ensuring our safety and freedom from the unpredictable forces of nature.
Doppler Radar Technology
Let's explore how Doppler radar technology enhances storm prediction.
We use the Doppler Effect to measure velocity data, allowing us to determine wind speeds and direction within a storm.
Advances in radar imaging provide us with more detailed and accurate storm structures, improving our predictive models.
Doppler Effect Principles
Doppler radar technology leverages the principles of the Doppler effect to measure the velocity and direction of storm systems with high accuracy. By analyzing frequency shifts, we can predict atmospheric disturbances with remarkable precision. This method hinges on wave propagation: as radar waves encounter moving storm particles, their frequency changes. These shifts reveal critical data about storm speed and trajectory, enhancing our storm tracking accuracy.
Key components of Doppler radar technology include:
- Frequency Shift Analysis: By detecting changes in radar wave frequency, we can determine the velocity of rain droplets and other storm elements.
- Wave Propagation: Radar waves travel through the atmosphere, and their interaction with storm systems provides data on storm structure and movement.
- Atmospheric Disturbance Prediction: Analyzing the collected data allows us to forecast weather disturbances, aiding in early storm warnings.
- Storm Tracking Accuracy: The precise measurement of storm movement helps in creating accurate weather models, ensuring better preparedness and response.
Velocity Data Analysis
Building on our understanding of the Doppler effect principles, we now focus on how velocity data analysis in Doppler radar technology enables us to quantify storm dynamics with unparalleled accuracy.
By measuring the frequency shift of radar signals reflected from precipitation particles, we can determine the velocity of these particles relative to the radar. This allows us to track storm movement and identify vital wind patterns within the storm system.
In real-time storm tracking, velocity data analysis lets us discern rotational patterns indicative of severe weather events like tornadoes.
By analyzing the inbound and outbound velocities of raindrops, we can detect the presence of mesocyclones, which are precursors to tornadoes. This capability provides essential lead time for issuing warnings and taking protective measures.
Moreover, velocity data helps us understand the vertical and horizontal wind shear within a storm.
Radar Imaging Advancements
With recent advancements in radar imaging, Doppler radar technology now offers unprecedented resolution and accuracy in storm detection and analysis. This cutting-edge technology enhances our storm tracking and weather forecasting capabilities, giving us greater freedom to prepare for severe weather events.
By measuring the velocity and movement of precipitation particles, Doppler radar provides real-time data that's vital for meteorologists.
Here are four key benefits of Doppler radar technology:
- Enhanced Clarity: Doppler radar can distinguish between different types of precipitation, such as rain, snow, and hail, with high precision. This allows for more precise storm tracking.
- Speed Detection: By detecting the speed and direction of precipitation particles, Doppler radar can identify wind patterns and potential tornado formations, which is essential for timely weather forecasting.
- Immediate Data: The technology delivers instant updates, allowing meteorologists to track storm development and movement as they happen, enhancing situational awareness.
- Broad Range: Doppler radar systems cover a larger geographic area, providing detailed data that helps in forecasting weather over extensive regions.
Machine Learning Models
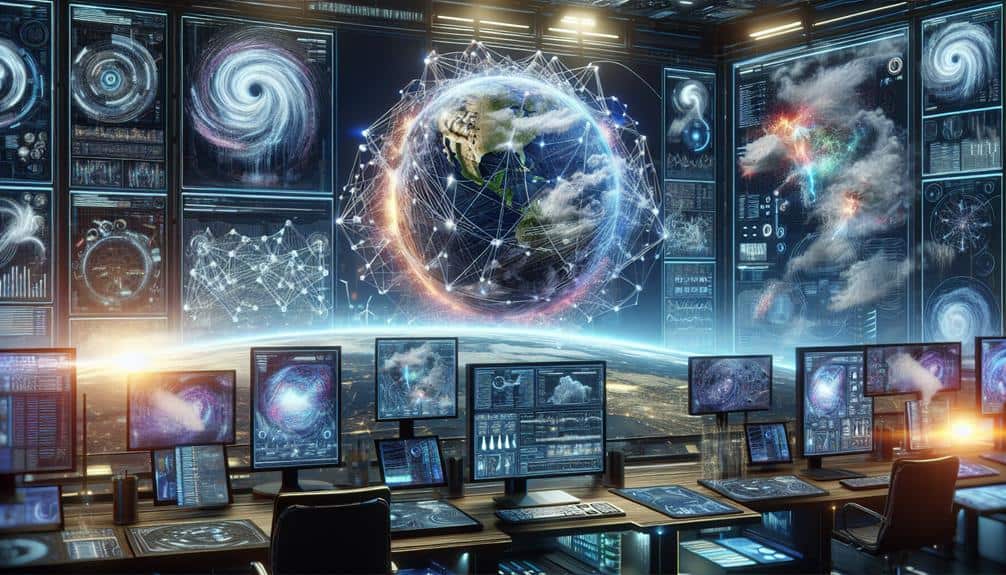
Leveraging advanced algorithms, machine learning models have revolutionized the way we predict storm patterns. By utilizing deep learning algorithms, we can analyze vast datasets that were previously unmanageable. These algorithms excel at identifying complex patterns in historical weather data, enabling us to make more accurate predictions.
Neural network predictions have become particularly invaluable. These networks, inspired by the human brain, process input data through interconnected layers to produce forecasts. By training these networks on extensive historical weather data, we can predict storm paths, intensity, and arrival times with remarkable precision. The adaptability of neural networks allows them to improve with each new data set, continuously refining their predictive capabilities.
Moreover, machine learning models offer the flexibility to incorporate various data sources, including satellite imagery and sensor readings. This integration enhances the model's accuracy and reliability. The real-time processing capability of these models empowers meteorologists and emergency planners, providing them with timely and actionable insights.
High-Resolution Weather Models
Let's explore how high-resolution weather models enhance our storm prediction capabilities.
With improved spatial resolution, we can identify finer details in storm structures, leading to more accurate forecasts.
Additionally, better data assimilation techniques allow us to integrate real-time observational data, further refining our models.
Enhanced Spatial Resolution
High-resolution weather models frequently enhance our ability to predict storms with greater spatial accuracy. By leveraging advanced computational techniques, we can achieve more precise storm tracking and weather forecasting. These models provide a deeper understanding of storm dynamics, which is essential for accurate predictions and timely warnings.
In terms of their benefits, high-resolution models allow us to:
- Identify localized weather patterns: These models enable the detection of small-scale weather phenomena that broader models might miss.
- Improve storm tracking: Enhanced spatial resolution allows for more accurate monitoring of storm paths, aiding in the prediction of potential impact zones.
- Optimize resource allocation: With precise weather forecasting, emergency services can better allocate resources to areas most likely to be affected.
- Enhance public safety: Accurate predictions lead to timely warnings, giving people the freedom to make informed decisions about their safety.
High-resolution models work by simulating the atmosphere with finer grid spacing, which captures smaller-scale processes more effectively. This precision is critical for regions with complex terrain or coastal areas where weather patterns can change rapidly.
Improved Data Assimilation
While high-resolution models enhance our spatial understanding of storms, the accuracy of these predictions hinges on improved data assimilation techniques. By integrating diverse data sources, we can refine atmospheric modeling to a great extent.
Advanced sensors, such as Doppler radar and satellite imaging, collect vast amounts of data in real time. These sensors provide granular details on temperature, humidity, wind speed, and pressure—crucial variables for storm prediction.
Machine learning algorithms play an essential role in processing this data. They analyze patterns and correlations that might go unnoticed in traditional models. By feeding these algorithms with real-time data, we improve our forecasting capabilities significantly. These models learn and adapt, continuously refining their predictions based on new incoming data.
Data assimilation isn't just about volume; it's also about accuracy and timeliness. High-resolution weather models benefit from the precise and immediate input that advanced sensors provide. The faster we can integrate this data into our models, the more accurate our forecasts become.
In essence, the combination of advanced sensors and machine learning algorithms revolutionizes real-time forecasting, offering us unprecedented precision in storm prediction. This empowers us to make informed decisions, ensuring greater preparedness and safety.
Data Assimilation Techniques
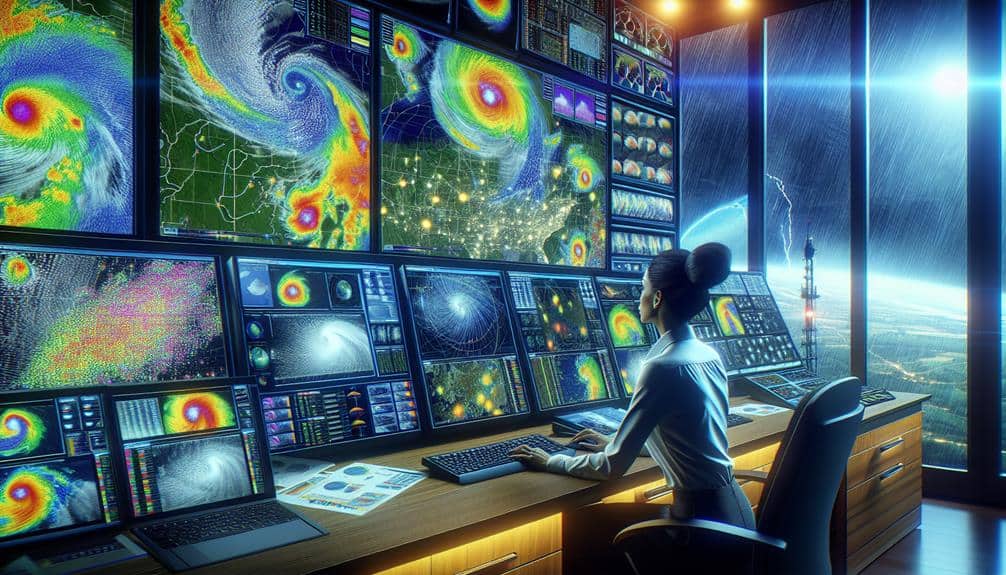
Data assimilation techniques integrate real-time observational data into predictive models to enhance the accuracy of storm forecasts. By incorporating atmospheric measurements and numerical simulations, we refine our meteorological forecasting capabilities, making weather prediction more reliable and timely. These techniques bridge the gap between raw data and actionable insights, ensuring that our models reflect current atmospheric conditions.
Consider the following key aspects of data assimilation:
- Observation Quality: High-quality atmospheric measurements from satellites, radars, and weather stations are essential. They provide the necessary data points for accurate numerical simulations.
- Model Integration: Combining observational data with numerical models involves intricate algorithms that adjust model variables to match real-world observations. This helps in minimizing errors in storm predictions.
- Real-Time Processing: Speed is vital. Real-time data assimilation allows for continuous updates to forecasting models, making them more responsive to rapidly changing weather conditions.
- Uncertainty Reduction: By integrating diverse data sources, we reduce uncertainty in weather predictions. More inclusive data leads to more precise forecasts, empowering individuals and communities to make informed decisions.
Social Media Data Analysis
Recognizing the importance of diverse data sources, we now explore how social media data analysis can enhance storm prediction models by providing real-time, ground-level observations from the public. Social media platforms are replete with posts, images, and videos that can offer valuable, immediate insights into weather conditions. By applying sentiment analysis, we can gauge public perception and emotions related to an impending storm, identifying areas of heightened concern or distress. This real-time data, when integrated with traditional meteorological inputs, can markedly improve the accuracy and timeliness of storm predictions.
Trend prediction algorithms allow us to detect emerging patterns in social media activity related to weather events. For instance, a sudden surge in posts mentioning heavy rainfall or strong winds can serve as an early indicator of developing storm conditions. By leveraging these trends, we can enhance our storm tracking and forecasting capabilities, ensuring that warnings are disseminated more swiftly and accurately.
Utilizing social media data analysis isn't just about adding another layer of information; it's about harnessing the collective, real-time experiences of individuals on the ground. This approach empowers us to build more responsive and adaptive storm prediction systems, ultimately providing greater independence from the uncertainties of severe weather.
Frequently Asked Questions
How Do Emergency Services Prepare for Predicted Storms?
We coordinate emergency response efforts by designing thorough evacuation plans, ensuring resources and personnel are strategically positioned. We prioritize maintaining communication channels and updating the public to facilitate informed and timely decisions, safeguarding their freedom and safety.
What Role Do Ocean Buoys Play in Storm Prediction?
Imagine ocean buoys as watchful sentinels. They gather essential data, enabling us to analyze and predict storms with precision. Their real-time monitoring offers invaluable insights, enhancing our storm prediction efforts and ensuring our freedom from unexpected disasters.
Can Storm Prediction Methods Be Used to Forecast Tornadoes?
Yes, we can adapt storm prediction methods for tornado warning and severe weather detection. Enhanced radar systems, atmospheric models, and real-time data analysis improve our ability to forecast tornadoes, providing critical lead time for safety measures.
How Accurate Are Long-Term Storm Predictions Compared to Short-Term Forecasts?
When we conduct an accuracy assessment of forecasting comparison, short-term forecasts generally outperform long-term predictions. Weather models and prediction techniques are more precise for immediate events, while long-term projections often suffer from higher uncertainty.
What Impact Does Climate Change Have on Storm Prediction Accuracy?
Climate change complicates storm prediction accuracy due to shifting weather patterns and increased storm intensity. We must refine our models and incorporate more data to address these variables, ensuring more reliable forecasts despite the evolving climate landscape.