We need actionable, data-driven strategies to advance our storm forecast atmospheric modeling techniques. First, we analyze meteorological data, including temperature, humidity, and wind patterns. High-resolution models are leveraged for capturing small-scale phenomena and improving accuracy through data assimilation. Satellite imagery offers real-time monitoring. By scrutinizing atmospheric dynamics, we gain insights into storm intensities and trajectories. Machine learning helps process vast datasets, revealing complex relationships. Real-time simulations with high-performance computing enhance precision. Finally, we validate forecast accuracy by comparing model predictions with observed data to fine-tune our models. By mastering these techniques, we'll greatly enhance our forecast capabilities.
Key Points
- Integrate high-resolution models to capture small-scale atmospheric phenomena and reduce prediction uncertainty.
- Employ data assimilation techniques to incorporate real-time observational data for enhanced forecast accuracy.
- Utilize satellite imagery for comprehensive monitoring and timely updates of storm systems.
- Leverage machine learning algorithms for pattern identification and adaptive learning to improve forecast precision.
Understand Meteorological Data
To accurately model storms, we need to thoroughly understand and analyze various types of meteorological data, including temperature, humidity, wind patterns, and atmospheric pressure. By interpreting trends in these data points, we can identify key indicators that precede storm development. For instance, rapid temperature changes and shifts in atmospheric pressure often signal the onset of severe weather conditions.
We must employ data visualization tools to make sense of this complex information. Graphs and heat maps allow us to spot anomalies and patterns that might otherwise go unnoticed. For example, a sudden spike in humidity levels, when visualized on a time-series graph, can alert us to potential storm formation.
Moreover, analyzing wind patterns through vector field plots helps us predict storm trajectories with higher accuracy. By combining these visualizations, we can create a comprehensive picture of the atmospheric conditions. This holistic approach enables us to make more informed and timely decisions, ultimately granting us the freedom to prepare and respond more effectively to impending storms.
In essence, mastering the interpretation of meteorological data through effective visualization techniques is essential for accurate storm forecasting. Let's harness this knowledge to enhance our predictive capabilities and safeguard our communities.
Utilize High-Resolution Models
High-definition models offer unmatched detail in storm prediction, allowing us to capture small-scale atmospheric phenomena that coarser models might overlook. These models offer crucial insights into the dynamics of severe weather systems, enabling us to forecast events like tornadoes, microbursts, and localized heavy rainfall with greater precision.
By integrating high-definition data, our forecasting becomes more accurate and responsive to real-time changes. Data assimilation techniques play a crucial role here. They allow us to incorporate observational data, such as temperature, humidity, and wind speed, directly into our models. This process enhances the initial conditions, leading to enhanced forecast accuracy.
Model validation is equally crucial. After running a high-definition model, we compare its outputs with observed data to evaluate its performance. This step is essential for identifying potential discrepancies and refining the model parameters accordingly. Validation ensures our models aren't just theoretically sound but also practically reliable.
Incorporating high-definition models and robust data assimilation processes grants us the freedom to make more informed decisions, reducing the uncertainty inherent in storm prediction. By validating these models continuously, we can maintain a high level of confidence in our predictive capabilities, ultimately protecting lives and property.
Leverage Satellite Imagery
Leveraging satellite imagery often provides us with real-time, high-resolution visual data essential for monitoring storm development and progression. By using satellite tracking, we can pinpoint the exact location and movement of storm systems. This enables us to anticipate changes in weather patterns more accurately, ensuring timely and effective response measures.
Our cloud analysis techniques allow us to examine cloud formations, their structures, and their potential for severe weather development.
Key benefits of leveraging satellite imagery include:
- Enhanced Precision: With high-resolution data, we can differentiate between various cloud types and precipitation intensities, leading to more accurate storm forecasting.
- Timely Updates: Satellite tracking offers near-instantaneous updates, allowing us to keep pace with rapidly evolving storm conditions and provide real-time alerts.
Analyze Atmospheric Dynamics
Analyzing atmospheric dynamics involves scrutinizing the complex interactions between various atmospheric layers to predict storm behavior accurately. We start with atmospheric pressure analysis, examining pressure variations from the surface to the upper troposphere. Precise pressure readings help us identify potential storm systems and their intensities.
Next, we focus on temperature gradient assessment. By monitoring temperature changes across different altitudes, we can understand the energy distribution within the atmosphere. Sharp temperature gradients often indicate unstable conditions, which are precursors to storm formation.
Wind pattern evaluation is essential as well. By studying wind speeds and directions at varying altitudes, we can pinpoint jet streams and wind shear zones. These wind patterns play a key role in the development and trajectory of storms.
Additionally, humidity level interpretation is crucial. High humidity levels, especially in the lower atmosphere, provide the moisture necessary for storm development. By analyzing humidity profiles, we can assess the likelihood of storm intensification.
Integrate Machine Learning
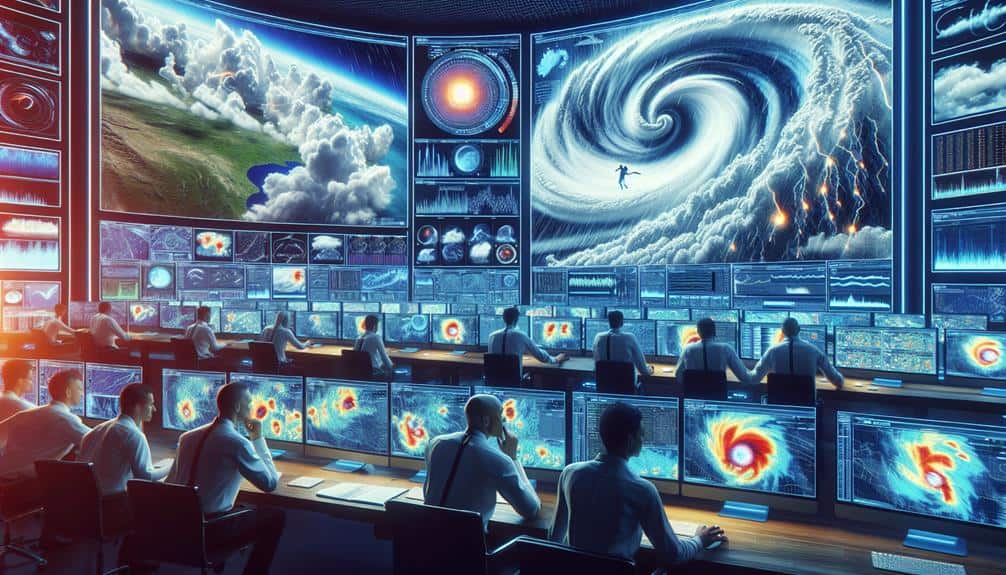
To enhance storm forecasting accuracy, we can integrate machine learning algorithms that process vast datasets to identify patterns and correlations undetectable by traditional methods. This approach enables us to leverage predictive analytics, transforming raw data into actionable insights.
Machine learning applications in atmospheric modeling offer several advantages:
- Data Fusion: Combining diverse data sources, such as satellite imagery, sensor readings, and historical weather patterns, to create an all-inclusive dataset.
- Pattern Recognition: Detecting complex, non-linear relationships within the data that are often missed by conventional statistical methods.
Conduct Real-Time Simulations
Let's focus on conducting real-time simulations by integrating high-resolution data, which enhances accuracy.
Utilizing advanced computational techniques allows us to process vast amounts of data swiftly.
It's pivotal to calibrate our models meticulously to guarantee reliability and predictive power.
High-resolution Data Integration
In real-time simulations, integrating high-detail data is necessary for improving the precision and dependability of storm forecast atmospheric models. To accomplish this, we focus extensively on data assimilation and model validation, making sure that our inputs are both accurate and timely.
By incorporating high-detail datasets, we can capture finer-scale atmospheric phenomena that coarser data would overlook. This leads to more reliable forecasts, which are vital for making informed decisions during storm events.
We undertake sensitivity analysis to identify which variables most significantly impact our models. This process helps us prioritize data sources and adjust our models to enhance performance. Model optimization then follows, where we tweak parameters and algorithms based on our findings to improve the overall accuracy and efficiency of our simulations.
Here are three crucial components of high-detail data integration:
- Data Assimilation: Ingesting real-time data from multiple sources to refine the initial conditions of our models.
- Model Validation: Continuously comparing our model outputs with observed data to ensure accuracy.
Advanced Computational Techniques
We leverage advanced computational methods to conduct real-time simulations that enhance the accuracy and responsiveness of our storm forecast models. By employing high-performance computing (HPC) systems, we can process vast amounts of atmospheric data at unprecedented speeds. This capability allows us to generate dynamic and precise storm forecasts, which are essential for timely decision-making.
Our approach focuses on integrating numerical weather prediction (NWP) models with machine learning algorithms. This hybrid methodology notably improves model accuracy by continuously updating the simulations based on the latest observational data. The real-time aspect ensures that our models aren't only accurate but also highly responsive to evolving weather conditions.
To achieve significant forecast improvements, we incorporate data assimilation techniques. These techniques refine the initial conditions of our models, making sure they closely match real-world observations. As a result, our simulations can predict storm behavior with higher fidelity, offering actionable insights for mitigation strategies.
In essence, advanced computational methods empower us to push the boundaries of storm forecasting. By optimizing both computational resources and data integration methods, we provide forecasts that aren't just accurate but also adaptive, meeting the demands of an ever-changing atmospheric landscape.
Model Calibration Essentials
Accurate storm forecasting hinges not just on advanced computational techniques but also on meticulous model tuning to guarantee real-time simulations align with observed atmospheric conditions. We need to employ robust tuning techniques that fine-tune our models, ensuring their outputs are both reliable and precise. This is essential for enhancing model precision and achieving consistent forecast validation.
To optimize our model tuning process, we should:
- Leverage historical information: By comparing real-time simulations with historical storm data, we can fine-tune our models, making necessary adjustments to improve accuracy.
- Utilize statistical approaches: Advanced statistical tools can help identify discrepancies between model outputs and observed data, facilitating targeted adjustments.
Through thorough data analysis, we can identify specific areas where our models deviate from observed conditions and implement corrective measures. This iterative process of tuning and validation ensures our forecasts remain credible and actionable.
Validate Forecast Accuracy
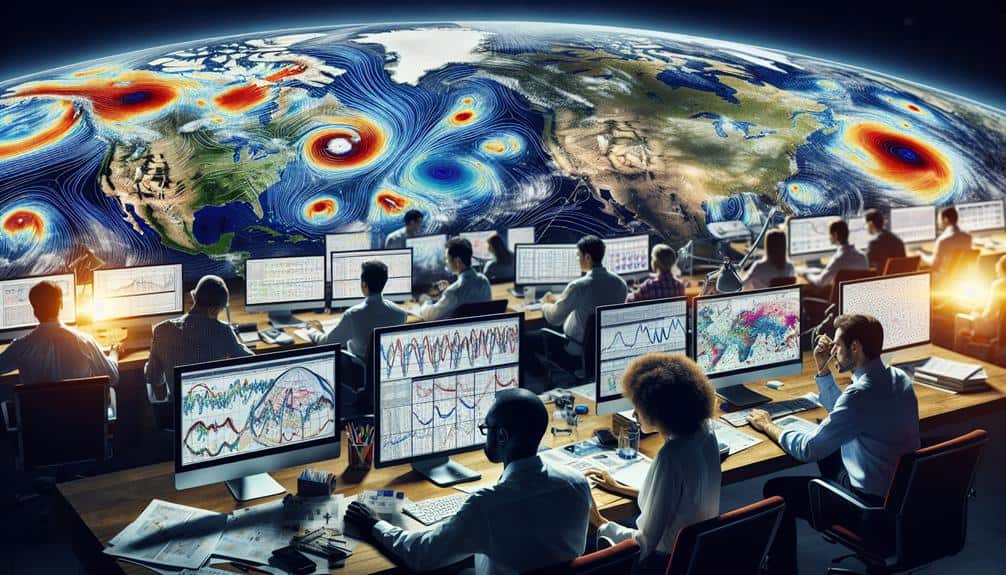
Consistently evaluating forecast accuracy involves comparing model predictions with observed atmospheric data to quantify disparities and refine predictive algorithms. This process, known as forecast verification and model validation, is essential for enhancing the dependability of storm forecast models. We need to utilize robust storm tracking and data analysis techniques to guarantee our models can predict atmospheric changes with high precision.
By systematically comparing predicted storm paths and intensities against actual observed data, we can identify specific areas where our models excel and where they fall short. This involves detailed statistical analysis, such as computing bias, root mean square error, and skill scores. These metrics allow us to measure the accuracy and dependability of our models.
Incorporating real-time data from radar, satellites, and weather stations is imperative for our analysis. We must verify the data is clean, high-quality, and representative of the atmospheric conditions. Once disparities are identified, we can make iterative improvements to the models, adjusting parameters, and incorporating new algorithms or data inputs.
Ultimately, our goal is to minimize forecast errors and provide more accurate and timely storm warnings. This empowers communities to make informed decisions and take proactive measures, ensuring safety and resilience against severe weather events.
Frequently Asked Questions
How Do Historical Weather Patterns Influence Storm Forecast Accuracy?
When we analyze Hurricane Katrina, historical weather patterns greatly enhance forecast accuracy. By leveraging data analysis and studying meteorological trends, we can predict storm paths more precisely, giving us the freedom to prepare and respond effectively.
What Role Do Ocean Temperatures Play in Storm Development?
We can't overlook the impact of ocean temperatures on storm development. Temperature anomalies and ocean circulation directly affect storm formation and intensity, providing critical data for accurate modeling and predictions. Freedom lies in understanding these dynamics.
How Can Public Safety Organizations Use Forecast Data Effectively?
We can improve emergency response by leveraging forecast data through advanced data visualization techniques, enabling us to quickly interpret storm patterns and allocate resources efficiently. This approach guarantees timely actions, maximizing public safety and operational freedom.
What Are the Common Challenges in Storm Surge Prediction?
Coincidentally, we often face challenges with data accuracy and predictive modeling. Storm surge prediction requires precise input, but data gaps and model limitations can lead to inaccuracies, complicating our efforts to guarantee timely and reliable warnings.
How Does Climate Change Impact the Frequency and Intensity of Storms?
Climate change drives extreme weather, increasing storm intensity and frequency. Global warming elevates sea surface temperatures, fueling more potent storms. Data shows a clear correlation between rising global temperatures and heightened storm activity, impacting communities worldwide.