To improve Doppler radar accuracy in storm forecasting, we implement advanced calibration techniques, reduce signal noise, and enhance data resolution. Calibrating our systems and using dual-polarization radar refines measurement precision. Adaptive filtering and real-time signal processing mitigate interference. Utilizing AI and machine learning models improves data quality control and predictive accuracy, ensuring better storm cell identification and path predictions. Enhanced spectral analysis and computational resource optimization further boost resolution. By integrating these technologies, we achieve more reliable forecasts, offering timely and precise storm warnings. With these strategies, we gain deeper insights into advancing our weather forecasting capabilities.
Key Points
- Utilize advanced signal processing algorithms to enhance data resolution and reduce noise.
- Implement real-time system adaptation for continuous model updates and precise forecasting.
- Integrate AI and machine learning to identify patterns and anomalies accurately.
- Perform regular calibration and quality control to maintain radar performance.
Understanding Doppler Radar
Doppler radar functions by transmitting radio waves and analyzing the frequency shifts caused by the motion of targets to measure their velocity and location. By employing Doppler principles, we can accurately ascertain the speed and direction of moving objects, such as precipitation particles within a storm system. This capability is vital for precise weather forecasting and timely storm warnings, which ultimately provide us with the freedom to prepare and respond effectively.
In Doppler radar technology, the radio waves emitted by the radar system encounter moving targets, like raindrops or snowflakes. These targets reflect the waves back to the radar receiver, where the frequency shift—referred to as the Doppler shift—is measured. The magnitude of this shift directly correlates to the velocity of the target. Fundamentally, a higher frequency shift indicates faster movement towards or away from the radar, enabling us to map out the storm's dynamics in real-time.
Common Accuracy Issues
Despite its advanced capabilities, Doppler radar often faces accuracy issues due to factors like signal weakening, ground clutter, and velocity aliasing. These challenges impact our ability to provide precise storm forecasts. Let's explore the common accuracy issues we encounter:
- Signal Weakening: As radar signals travel through the atmosphere, they can diminish, particularly in heavy precipitation, leading to reduced accuracy.
- Ground Clutter: Objects such as buildings, trees, and terrain can reflect radar signals, creating false echoes that complicate data interpretation.
- Velocity Aliasing: The radar's inability to accurately determine the speed of fast-moving weather phenomena can result in misleading velocity data.
- Data Interpretation Challenges: Differentiating between various weather patterns can be challenging, especially when faced with complex meteorological scenarios.
Error correction techniques are essential to mitigate these issues, but they come with their own set of data interpretation challenges. By understanding these limitations, we can better appreciate the complexity of storm forecasting and work towards more accurate predictions. Addressing these common accuracy issues will enable us to refine our forecasting methodologies, offering greater freedom and reliability in weather prediction.
Calibration Techniques
Let's focus on our calibration techniques by enhancing equipment precision, controlling data quality, and reducing signal noise.
We should analyze the calibration data to identify deviations and implement corrective measures.
Equipment Precision Enhancement
Calibrating our radar systems with cutting-edge techniques significantly enhances measurement precision and reliability. By utilizing rigorous performance evaluation and capitalizing on the latest technology advancements, we can achieve unparalleled accuracy in our weather prediction strategies. Precision enhancement is crucial in refining the data we collect, guaranteeing our storm forecasting models are as dependable as possible.
To enhance our calibration process, we incorporate a variety of advanced methods:
- Signal-to-Noise Ratio Analysis: By examining the signal-to-noise ratio, we can filter out unnecessary data, ensuring clearer and more precise readings.
- Reflectivity Adjustment: Tweaking the reflectivity settings enables us to better assess the intensity of precipitation, directly influencing the precision of our forecasts.
- Velocity De-aliasing: This method involves rectifying velocity data to eliminate uncertainties and enhance the reliability of wind speed measurements.
- Dual-Polarization Tweaks: Using dual-polarization radar offers more intricate details on precipitation types, improving our capacity to predict severe weather events.
Data Quality Control
To secure the highest level of data fidelity, our calibration techniques utilize real-time adjustments and sophisticated algorithms to systematically eliminate errors and biases. We employ advanced error detection mechanisms that continuously monitor incoming data, allowing us to identify and correct discrepancies immediately. By integrating machine learning models, we enhance our ability to predict potential sources of error and adjust our systems dynamically.
Our quality assurance protocols are designed to maintain the integrity of the data throughout the entire collection process. We implement robust statistical methods to validate the accuracy of our measurements, guaranteeing that each data point meets strict standards. This involves cross-referencing radar data with ground truth observations and other meteorological data sources.
We also conduct periodic calibration checks using known reference targets. These checks help us assess the radar's performance and recalibrate as necessary to maintain peak accuracy. By employing these meticulous calibration techniques, we ensure that our Doppler radar systems provide the most reliable data possible for storm forecasting.
Signal Noise Reduction
In our pursuit of minimizing signal noise, we utilize a combination of adaptive filtering techniques and real-time signal processing algorithms to enhance the clarity of our Doppler radar data. By integrating these advanced methods, we can more effectively perform noise filtering and algorithm optimization to achieve higher accuracy in storm forecasting.
To further refine our approach, we focus on several key strategies:
- Adaptive Filtering: We continuously adjust our filters based on the changing characteristics of the incoming radar signals.
- Real-time Signal Processing: Our systems process data in real-time, enabling immediate interference mitigation and more accurate readings.
- Algorithm Optimization: We regularly update and refine our algorithms to ensure they're operating at peak efficiency, minimizing error margins.
- Interference Mitigation: We implement techniques to identify and eliminate sources of interference, such as atmospheric anomalies or man-made obstacles.
Enhancing Data Resolution
By integrating advanced signal processing algorithms, we can greatly enhance the resolution of Doppler radar data, leading to more precise atmospheric measurements. This resolution improvement is vital for accurate data interpretation, allowing us to detect even minute changes in storm dynamics. Higher resolution data enables us to discern finer details within storm structures, which is essential for timely and accurate storm tracking.
Our approach involves leveraging sophisticated techniques such as spectral analysis and adaptive filtering. Spectral analysis helps us to isolate and amplify relevant signal components, reducing the impact of noise and enhancing the clarity of the radar images. Adaptive filtering, on the other hand, dynamically adjusts to the signal variations, ensuring consistent precision enhancement across different atmospheric conditions.
The benefits of these advancements are twofold. First, improved resolution directly translates to more detailed radar imagery, which facilitates better identification of storm cells and their potential paths.
Second, precision enhancement leads to more reliable predictions, reducing false alarms and ensuring that warnings are issued only when necessary. This not only increases the trust in our forecasting systems but also empowers individuals and communities to make informed, timely decisions, thereby maximizing their freedom to act proactively.
Integrating AI and Machine Learning
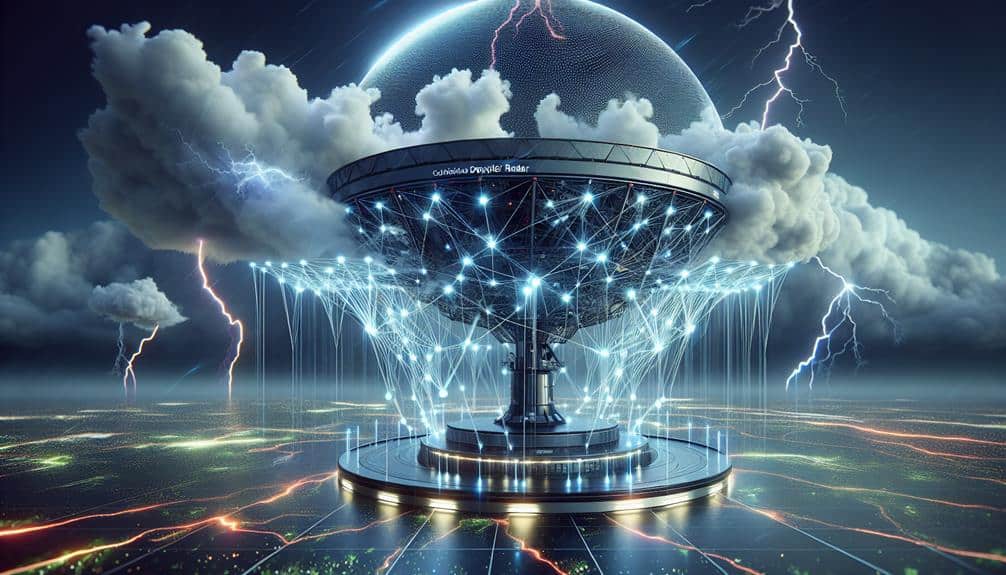
As we integrate AI and machine learning into Doppler radar systems, we enhance data processing capabilities, enabling more accurate and timely weather predictions.
By employing predictive analysis models, we can identify patterns and anomalies with greater precision.
Additionally, real-time system adaptation allows our radar systems to continuously improve their accuracy and reliability.
Enhanced Data Processing
Leveraging AI and machine learning algorithms, we can greatly enhance the accuracy and efficiency of Doppler radar data processing. By integrating advanced algorithms, we're able to analyze vast datasets more effectively, leading to improved precision in storm forecasting. Real-time processing of radar data not only accelerates our response times but also enhances the accuracy of our predictions.
To achieve this, we focus on several key areas:
- Data Cleansing: Removing noise and irrelevant data to ensure only high-quality information is processed.
- Pattern Recognition: Identifying weather patterns and anomalies faster than traditional methods.
- Adaptive Learning: Continuously updating models based on new data to enhance precision over time.
- Resource Allocation: Efficiently utilizing computational resources to handle large volumes of data.
Predictive Analysis Models
Building on the foundation of improved data processing, our focus now shifts to developing advanced predictive analysis models using AI and machine learning, aiming to forecast weather events with unparalleled accuracy. Leveraging these technologies, we can analyze vast datasets from Doppler radar systems, extracting patterns and trends that traditional methods might overlook.
Machine learning algorithms provide the flexibility to adapt to new data inputs, enhancing storm prediction accuracy. By training these models on historical weather data, we enable them to recognize precursors to severe weather phenomena, such as tornadoes or hurricanes, with greater reliability. Our approach involves using convolutional neural networks (CNNs) and recurrent neural networks (RNNs) to process spatial and temporal data, respectively, ensuring thorough analysis.
Advanced algorithms like gradient boosting and ensemble methods further refine our predictions by combining outputs from multiple models, thereby reducing errors and increasing confidence in forecasts. This iterative process of training, validation, and testing ensures our models aren't only accurate but also robust against outliers and anomalies.
As we integrate these predictive analysis models, we empower meteorologists and emergency management teams to make more informed decisions, ultimately enhancing public safety and granting society greater freedom from the unpredictable impacts of severe weather.
Real-time System Adaptation
We integrate real-time system adaptation by utilizing AI and machine learning to continuously refine Doppler radar data interpretation, ensuring immediate responsiveness to evolving weather conditions. Adaptive algorithms enhance the accuracy of storm forecasts by dynamically monitoring incoming data and adjusting models instantly. These advances provide us with a more precise understanding of atmospheric changes, essential for anticipating severe weather events.
Our adaptive system operates on several key principles:
- Data Assimilation: Incorporating real-time data from various sensors to update models continuously.
- Predictive Analytics: Leveraging machine learning to anticipate weather patterns and anomalies.
- Anomaly Detection: Identifying irregularities in radar data to refine forecasting accuracy.
- Model Calibration: Adjusting parameters in real-time to improve model precision.
Real-time Data Processing
How can we improve Doppler radar accuracy through real-time data processing techniques that integrate advanced algorithms and high-speed computational resources?
To start, we need to focus on algorithm optimization. By refining our algorithms, we can ensure that our data processing is both swift and precise. This involves leveraging machine learning techniques to adaptively fine-tune parameters, improving the radar's ability to distinguish between noise and genuine meteorological signals.
Next, we must emphasize data visualization. When we can visualize data in real-time, it allows meteorologists to make swift, informed decisions. High-resolution, dynamic visualizations highlight vital storm features and trends that static images might miss. This capability is essential for anticipating sudden changes in storm intensity or direction, potentially saving lives and property.
Combining these elements, we utilize high-speed computational resources to handle the immense data throughput required for real-time processing. Modern GPUs and parallel computing architectures enable us to process vast amounts of radar data with minimal latency. This rapid processing is important for updating forecasts in real-time, providing the public with up-to-the-minute information.
Future Innovations in Radar Technology
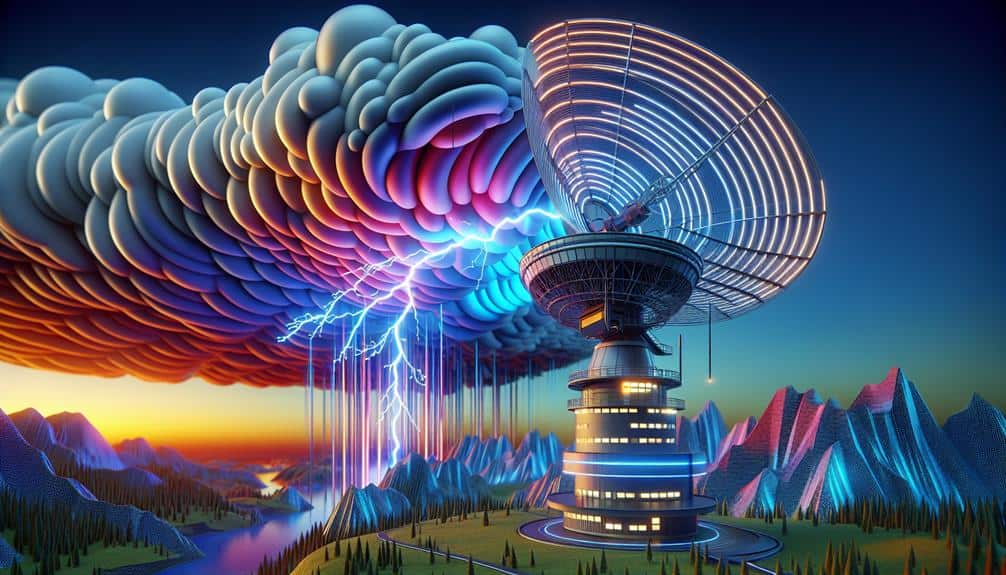
Advancements in artificial intelligence and quantum computing promise to revolutionize the capabilities of future radar systems, pushing the boundaries of detection accuracy and forecast reliability. By leveraging these cutting-edge technologies, we can enhance radar technology advancements and integrate sophisticated data visualization techniques to provide clearer, more actionable insights.
AI-driven weather prediction algorithms can process vast amounts of atmospheric data at unprecedented speeds, refining storm tracking innovations. Quantum computing, with its ability to handle complex calculations, can further optimize these algorithms, leading to more precise and timely forecasts.
Our focus areas include:
- Enhanced Signal Processing: Utilizing AI to filter out noise and improve signal clarity.
- Predictive Analytics: Implementing machine learning models to predict weather patterns with higher accuracy.
- Quantum Enhancement: Applying quantum computing for faster data processing and simulation.
- Advanced Visualization: Developing new data visualization techniques to present radar data intuitively.
These innovations don't just promise improved accuracy—they empower us with the freedom to make better-informed decisions.
As we continue to push the envelope in radar technology advancements, the future of storm forecasting will be marked by unprecedented precision and reliability.
Frequently Asked Questions
How Does Doppler Radar Differ From Traditional Radar Systems?
A bird in the hand is worth two in the bush; Doppler radar technology offers real-time data, enhancing weather prediction and data analysis. Unlike traditional radar systems, it measures velocity, overcoming limitations in applications like tracking storm movement.
What Are the Primary Benefits of Using Doppler Radar in Meteorology?
We benefit from Doppler radar's enhanced detection and improved warning accuracy. By accurately measuring storm velocity and precipitation, it provides timely, precise data, empowering us to make informed decisions and safeguard our communities' safety and freedom.
Can Doppler Radar Be Used to Track Tornadoes Effectively?
Yes, we can use Doppler radar to track tornadoes effectively. The radar technology enhances tornado detection by analyzing wind velocity patterns, providing real-time data that helps us predict and respond to these severe weather events swiftly.
How Do Weather Balloons Complement Doppler Radar Data?
Did you know weather balloons reach 100,000 feet? Balloon data gives us upper air wind profiles that, when integrated with radar data, enhance accuracy. This radar integration offers a clearer picture of storm dynamics, aiding in precise forecasts.
What Is the Cost Implication of Upgrading Existing Radar Systems?
When we conduct a cost analysis of upgrading radar systems, we must consider various funding sources. By securing public and private investments, we can efficiently manage expenses and guarantee the upgrades provide significant benefits without financial burdens.