We're revolutionizing storm interception using advanced data analysis techniques, ensuring precise data accuracy crucial for robust predictive models. By integrating Doppler radar, weather satellites, and unmanned aerial systems, we gather thorough real-time data. Machine learning algorithms and remote sensing enhance our ability to decode intricate atmospheric patterns and update models instantaneously. Tools like ensemble methods and neural networks for storm trajectory predictions are fine-tuned with continuous real-time data integration. This facilitates optimized resource deployment and efficient storm path visualization, greatly enhancing intercept strategies. Stay with us to explore the cutting-edge advancements driving these capabilities.
Key Points
- Utilize Doppler radar and weather satellites for accurate real-time atmospheric data collection.
- Implement machine learning algorithms for processing and recognizing complex storm patterns.
- Employ ensemble methods and neural networks to enhance storm trajectory predictions.
- Integrate real-time data analytics for rapid and efficient resource deployment during storm interception.
Importance of Accurate Data
Accurate data is crucial for developing predictive models that can effectively forecast and intercept storms. When we're discussing storm tracking, precise data accuracy is non-negotiable. Without reliable data, our predictive models become mere approximations, lacking the robustness needed to accurately predict storm paths. This, in turn, compromises our ability to provide early warnings and implement timely interventions.
Let's think about the implications: real-time storm tracking hinges on the precision of meteorological data. We gather data points on wind speeds, atmospheric pressure, humidity levels, and temperature gradients, among others. These variables must be accurate to the smallest degree because even minor errors can lead to significant deviations in storm trajectory predictions.
By ensuring data accuracy, we empower our predictive algorithms to generate highly reliable forecasts. These forecasts are critical for decision-makers who value their freedom to act swiftly and accurately. For instance, communities can evacuate in advance, aviation routes can be adjusted, and emergency services can be pre-positioned to mitigate the storm's impact.
In essence, our commitment to data accuracy directly translates to enhanced storm tracking capabilities, giving us the freedom to proactively manage and intercept storms, thereby reducing potential damage and saving lives.
Tools for Data Collection
To capture the precise meteorological data essential for storm interception, we deploy a variety of sophisticated instruments such as Doppler radar, weather satellites, and unmanned aerial systems. These tools enable us to gather high-resolution data essential for accurate storm prediction and tracking.
Doppler radar provides real-time data on precipitation, wind speed, and direction, allowing us to detect storm formation and intensity changes with remarkable accuracy. Weather satellites offer extensive coverage, capturing cloud patterns, temperature variations, and atmospheric moisture levels from space. Unmanned aerial systems (UAS) or drones, equipped with sensors, fly into areas too dangerous for manned aircraft, collecting detailed in-situ measurements.
Remote sensing technologies are integral to our data collection strategy. They enable us to monitor vast areas with minimal human intervention, ensuring continuous data flow. We also employ machine learning techniques to process and interpret the massive volumes of data collected. These algorithms help identify patterns and anomalies that might be missed by traditional analysis methods.
Key tools we use include:
- Doppler radar: For real-time precipitation and wind data.
- Weather satellites: For wide-area atmospheric monitoring.
- Unmanned aerial systems: For in-situ data collection in hazardous zones.
Analyzing Atmospheric Patterns
By leveraging advanced computational models, we can decode complex atmospheric patterns that drive storm behavior. Our focus is on harnessing machine learning algorithms to analyze massive datasets, including temperature gradients, humidity levels, and wind velocities. These algorithms sift through terabytes of data to identify subtle correlations and anomalies that traditional methods might overlook.
Using machine learning, we enhance weather forecasting by training models on historical storm data and real-time atmospheric conditions. The models learn to recognize patterns associated with storm genesis, growth, and dissipation. This process involves supervised learning, where algorithms are fed labeled data, and unsupervised learning, where the system identifies patterns without predefined labels.
To make sure accuracy, we utilize cross-validation techniques, splitting datasets into training and testing subsets. This helps in fine-tuning the models, reducing overfitting, and improving generalizability. Additionally, feature selection methods allow us to pinpoint the most significant variables influencing storm activity, optimizing computational efficiency.
Our data-driven approach empowers us to forecast storms with greater precision, offering communities the freedom to prepare and respond proactively. By continuously updating our models with new data, we keep our weather forecasting capabilities at the cutting edge, ensuring robust and reliable storm interception.
Predictive Modeling Techniques
Predictive modeling techniques, particularly ensemble methods and neural networks, enable us to simulate storm trajectories with unprecedented accuracy. These sophisticated algorithms harness vast datasets, allowing us to predict storm paths with a degree of precision that was previously unattainable. By leveraging machine learning, we can integrate a multitude of variables, from atmospheric pressure to wind speed, and generate highly reliable forecasts.
One of the key advantages of these techniques lies in their ability to adapt and improve over time. As new data becomes available, models are continuously refined, enhancing their predictive power.
- Ensemble Methods: By combining multiple models, we reduce the likelihood of single-model errors and increase overall forecast reliability.
- Neural Networks: These algorithms excel in recognizing complex patterns within large datasets, making them particularly effective for storm trajectory predictions.
- Real-time Data Integration: Continuous data feeds allow us to update our predictions instantaneously, ensuring that our models remain current and accurate.
Enhancing Field Strategies
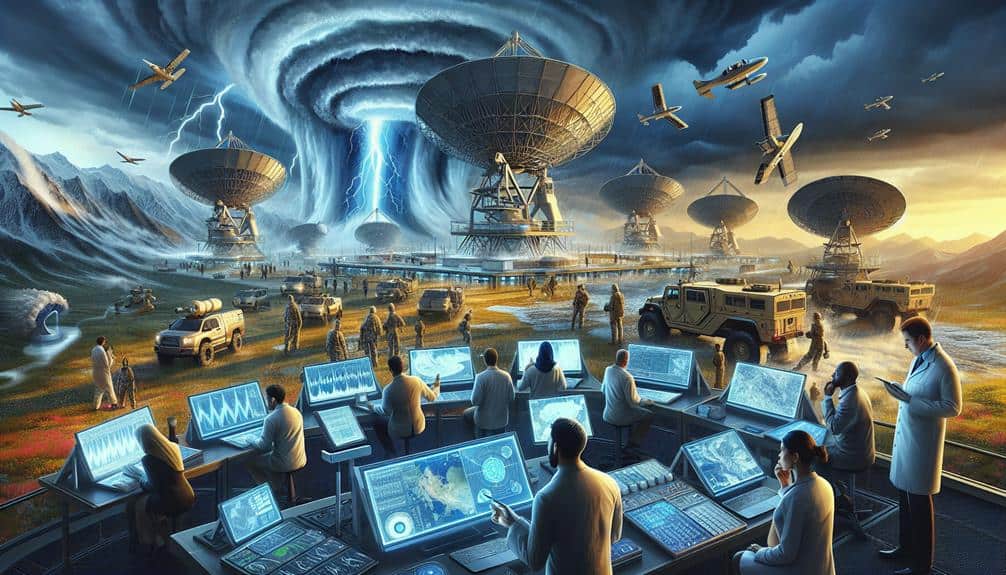
We can optimize field strategies by leveraging real-time data analytics to deploy resources more effectively during storm interception. By integrating high-frequency data streams from weather sensors and satellites, we can enhance intercept efficiency significantly. This enables us to make rapid, informed decisions on resource allocation and positioning.
Our primary focus is on target localization, which is crucial for pinpointing the most volatile segments of a storm. Utilizing machine learning algorithms, we can analyze vast datasets to predict storm paths with greater accuracy. These insights allow us to deploy storm chasers and emergency responders to precise locations, minimizing response times and maximizing safety.
Moreover, real-time communication between field units and central command centers ensures seamless coordination. We use advanced geospatial mapping tools to visualize storm progression and potential impact zones. By overlaying real-time data on these maps, we can dynamically adjust our strategies as the storm evolves.
Incorporating these advanced data analysis techniques, we not only improve our intercept efficiency but also empower our teams to act with greater autonomy and precision. This approach guarantees that our field strategies are both proactive and adaptive, aligning with our commitment to freedom and safety.
Frequently Asked Questions
How Do Advanced Data Analysis Techniques Compare to Traditional Methods in Storm Prediction Accuracy?
Advanced data analysis techniques, including statistical modeling and machine learning, outperform traditional methods in storm prediction accuracy. Predictive analytics provide real-time insights, offering us greater freedom to prepare and respond effectively to severe weather events.
What Is the Role of Machine Learning in Enhancing Storm Interception Strategies?
Isn't it ironic how machine learning applications, often considered futuristic, are now pivotal in storm interception? We leverage predictive modeling techniques to refine our strategies, ensuring accurate forecasts and timely interventions, granting us the freedom to act decisively.
How Can We Ensure Data Privacy and Security During Storm Data Collection and Analysis?
We can guarantee data privacy and security during storm data collection and analysis by implementing robust data encryption and employing secure data storage solutions. These measures protect sensitive information and uphold users' freedom and trust in our systems.
What Are the Challenges in Integrating Real-Time Data From Various Sources for Storm Interception?
Integrating real-time data presents challenges like data integration complexities, latency issues, and inconsistent data formats. We must guarantee seamless, timely data flow from various sources to maximize the effectiveness of our storm interception efforts.
How Can Community Involvement Improve the Effectiveness of Storm Interception Initiatives?
Community engagement and citizen science enhance storm interception by boosting public awareness and providing volunteer training. By leveraging local knowledge and real-time data, we can improve predictive models and facilitate faster, more effective responses to storm threats.