We enhance storm prediction algorithms by combining historical storm data, real-time weather updates, and cutting-edge machine learning techniques. We analyze historical data to identify migratory shifts and storm patterns, then validate these models using metrics like precision and recall. By integrating real-time weather updates and leveraging mobile applications, our dynamic system adjusts predictions instantly. High-resolution radar and satellite imaging further refine our predictive accuracy. Machine learning also plays a critical role, continuously fine-tuning models to identify key storm indicators. To uncover more about how these improvements benefit storm chasers, explore the following details.
Key Points
- Incorporate real-time weather updates to dynamically adjust storm predictions for enhanced accuracy.
- Utilize machine learning to analyze historical data and identify key storm formation indicators.
- Enhance radar and satellite imaging for detailed and accurate storm tracking.
- Validate algorithm accuracy using performance metrics like precision and recall with real-time and historical data.
Analyzing Historical Storm Data
By leveraging extensive historical storm data, we can identify patterns and trends that are essential for enhancing our predictive models. Historical data serves as a foundational element in understanding recurring climate patterns. When we systematically analyze this wealth of information, we can pinpoint variables that greatly influence storm behavior.
Our approach involves rigorous data visualization techniques to decode complex datasets. Utilizing advanced algorithms, we transform raw data into comprehensible visual formats. These visualizations allow us to observe anomalies and regularities within climate patterns, providing a clearer picture of storm genesis and progression.
For instance, plotting storm tracks over decades can reveal migratory shifts influenced by changing climatic conditions.
Analyzing historical storm data also helps us quantify the frequency and intensity of past storms. By categorizing these events, we identify thresholds that might indicate the onset of severe weather. This analytical process is pivotal for improving our predictive accuracy.
Integrating Real-Time Weather Updates
Building on our insights from historical storm data, we now turn our attention to integrating real-time weather updates to enhance the immediacy and accuracy of our storm prediction models. By incorporating continuous weather monitoring through advanced mobile applications, we can achieve a dynamic system that adjusts predictions based on live data. These applications utilize GPS tracking to pinpoint storm chasers' locations, safeguarding that our emergency alerts are both timely and context-specific.
The integration of real-time weather updates allows us to refine our models more effectively. Real-time data feeds from weather stations, satellites, and radar systems provide an up-to-the-minute snapshot of atmospheric conditions. This data-driven approach guarantees that our models aren't only reactive but also predictive, allowing for more precise storm path forecasts.
Moreover, emergency alerts can be customized to the user's immediate environment, enhancing personal safety and decision-making autonomy. By leveraging mobile applications, we empower storm chasers with tools that offer real-time situational awareness.
The synergy between historical data and live updates creates a robust framework for storm prediction, ultimately granting storm chasers the freedom to navigate safely and effectively in the field.
Leveraging Machine Learning Techniques
Leveraging machine learning techniques, we can analyze vast datasets to uncover patterns and improve the predictive accuracy of our storm models. By employing advanced predictive modeling, we're able to sift through historical weather data, real-time sensor inputs, and environmental variables to identify key indicators of storm formation and intensity. Machine learning algorithms excel at recognizing complex, non-linear relationships within this data, allowing us to forecast storm paths and behaviors with exceptional precision.
Algorithm optimization plays a pivotal role in this process. By fine-tuning our models through iterative training and validation, we can enhance their performance and reliability. Techniques such as gradient boosting and deep learning enable us to refine our predictive models continually, adapting to new data and evolving weather patterns. This dynamic approach guarantees our storm prediction algorithms remain cutting-edge and highly responsive.
Our aim is to empower storm chasers with actionable insights, allowing them to make informed decisions and maximize their freedom in the field. By leveraging machine learning, we not only improve the accuracy of our forecasts but also enhance the overall safety and effectiveness of storm chasing operations. This fusion of data-driven analysis and algorithmic precision represents the future of meteorological science.
Enhancing Radar and Satellite Imaging
Frequently, improving radar and satellite imaging involves integrating high-definition data to provide more detailed and accurate storm visualizations. We need to focus on enhancing radar resolution to detect finer-scale meteorological phenomena. By refining radar resolution, we can capture smaller storm cells and intricate precipitation patterns, enabling more timely and precise predictions.
Simultaneously, expanding satellite coverage ensures we don't miss important storm developments. Enhanced satellite coverage allows us to monitor wide geographical areas, essential for tracking storm systems that evolve over large regions. High-definition satellite imagery, combined with extensive coverage, offers a robust observational framework.
Image processing techniques play a vital role in this scenario. Advanced algorithms help us extract meaningful information from raw data, enhancing the clarity and usability of radar and satellite images. Integrating these processed images through data fusion techniques allows us to produce a coherent and thorough storm visualization. Data fusion combines multiple data sources, leading to more reliable and holistic storm tracking.
Validating Algorithm Accuracy
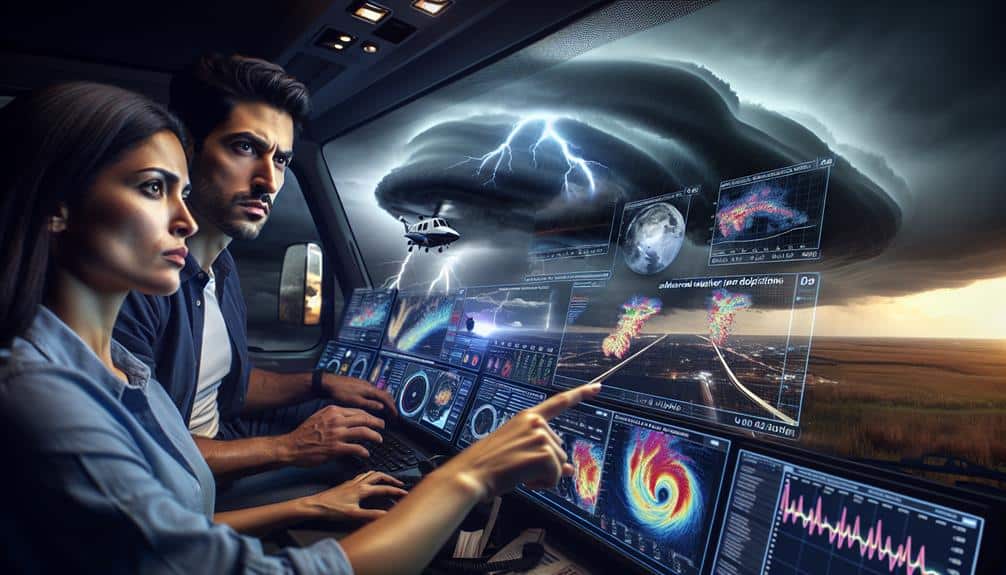
Ensuring the precision of storm prediction algorithms requires rigorous validation against historical weather data and real-time observations. We need to establish a robust framework for data validation and accuracy assessment to secure our algorithms deliver reliable forecasts. This process involves comparing predicted storm events with actual outcomes and adjusting the models accordingly to minimize discrepancies.
Key components of our validation process include:
- Historical Data Analysis: We analyze decades of archived weather data to identify patterns and trends, using this information to benchmark our algorithm's performance.
- Real-Time Testing: By integrating real-time observations, we can immediately assess the algorithm's accuracy and make necessary adjustments.
Algorithm optimization is essential for improving performance. By continuously fine-tuning our models, we can enhance their predictive capabilities. Performance testing helps us identify areas for improvement, securing that our algorithms remain adaptive and responsive to new data inputs.
Freedom to chase storms relies on our confidence in these algorithms. With rigorous validation and ongoing optimization, we can trust our tools to provide accurate, real-time predictions, empowering us to make informed decisions in the field.
Frequently Asked Questions
How Can Storm Prediction Algorithms Benefit Local Communities and Emergency Services?
How can we enhance community safety and emergency response? By refining storm prediction algorithms, we provide timely, accurate data that empowers local communities and emergency services to act swiftly, minimizing risks and safeguarding lives and properties.
What Are the Ethical Considerations in Storm Prediction Technology?
In addressing the ethical considerations in storm prediction technology, we must analyze privacy concerns and data ownership. Ensuring transparent data practices and protecting individuals' privacy rights are paramount to maintaining trust and freedom in our technological advancements.
How Do Storm Chasers Contribute to Improving Prediction Algorithms?
We gather critical data collection during storms, enabling real-time analysis. Our contributions provide invaluable insights, refining prediction algorithms. This empowers us with accurate, timely information, enhancing our freedom to navigate and understand extreme weather phenomena effectively.
What Funding Sources Support Research in Storm Prediction Technologies?
We rely on government grants and private sponsorships to fund research in storm prediction technologies. These funding sources enable us to leverage cutting-edge scientific methodologies and data analysis, ensuring accurate, timely weather forecasting while maintaining our operational independence.
How Do International Collaborations Enhance Storm Prediction Capabilities?
International collaborations enhance storm prediction capabilities by facilitating data sharing and model validation. These partnerships expand our datasets, refine predictive models, and improve accuracy, ultimately providing us with greater freedom to anticipate and respond to severe weather events.