When we analyze historical data for predicting storm formations, we uncover patterns and trends that enhance our forecasting accuracy. Using advanced algorithms, we pinpoint critical indicators like atmospheric pressure, humidity, and sea surface temperatures linked to storm activity. These insights, combined with current conditions, refine our predictive models and reduce errors. Historical data outperforms real-time data alone, empowering us to make informed decisions for disaster preparedness and minimizing damage. This method has proven effective in past events, such as Hurricane Katrina and Typhoon Haiyan. Exploring this further will reveal how these techniques continue to evolve and improve.
Key Points
- Identifying recurring patterns and trends in historical data aids in predicting storm formations.
- Historical data visualizations simplify understanding complex meteorological information.
- Cross-referencing past data with current conditions validates predictive models.
- Historical data integration reduces forecasting errors and enhances accuracy.
Importance of Historical Data
By analyzing historical data, we can identify patterns and trends that are important for accurately predicting storm formations. Leveraging vast datasets collected over decades, we're able to create detailed data visualizations that make complex information more accessible. These visualizations allow us to discern recurring patterns, providing vital insights into storm behaviors and trajectories.
Our approach involves employing advanced pattern recognition algorithms to sift through historical data meticulously. By doing so, we can highlight correlations between various meteorological variables—such as sea surface temperatures, atmospheric pressure anomalies, and wind shear levels—that often precede storm formations. This method isn't just about spotting obvious trends; it's about uncovering subtle shifts and anomalies that may indicate future storm activity.
Data-driven analysis empowers us to make informed predictions, granting us a higher degree of freedom in decision-making. Whether we're preparing for potential evacuations or optimizing resource allocation, the precision and accuracy derived from historical data guarantees that our actions are grounded in solid evidence.
Essentially, historical data isn't just a record of the past; it's a powerful tool that shapes our preparedness and resilience against future storms.
Identifying Storm Patterns
Using advanced algorithms, we meticulously analyze meteorological variables to identify recurring storm patterns with precision and accuracy. By processing extensive datasets, we pinpoint critical indicators such as atmospheric pressure, humidity levels, and sea surface temperatures that consistently precede storm formations. This data-driven approach allows us to decode intricate weather patterns and improve storm tracking capabilities.
Our analysis isn't just theoretical; it's firmly grounded in empirical evidence. We cross-reference historical storm data with current meteorological conditions to validate our findings. For example, we observe that specific shifts in jet stream positions often correspond with the onset of severe weather events. By recognizing these patterns, we can more precisely predict future storm activity and provide timely warnings.
Moreover, our storm tracking methodologies involve continuously updating our models with real-time data. This dynamic approach guarantees that we adapt to evolving weather patterns and refine our predictive accuracy. The freedom to access and interpret this data empowers us to make informed decisions and enhance public safety.
Enhancing Predictive Models
We consistently refine our predictive models by integrating machine learning techniques and leveraging vast amounts of historical and real-time meteorological data. By doing so, we can identify data trends that greatly enhance model accuracy. Understanding these trends allows us to anticipate storm formations more precisely, offering better preparation and minimizing potential damage.
To visualize the process, consider:
- Data Collection: We gather extensive datasets from satellites, weather stations, and ocean buoys, ensuring a thorough repository of historical and current weather conditions.
- Model Training: Using machine learning algorithms, we train our models on these datasets, allowing them to recognize patterns and predict future storm formations with enhanced accuracy.
- Validation and Testing: We rigorously test our models against historical data to validate their predictive power, continually refining them to improve their performance.
These steps are essential in enhancing our predictive capabilities. The detailed analysis of data trends not only boosts model accuracy but also empowers us to make informed decisions.
Reducing Forecasting Errors
As we refine our predictive models, it's important to address and reduce forecasting errors to ensure the highest possible accuracy in storm predictions. Minimizing uncertainties in our forecasts demands a thorough analysis of historical data. By examining past storm formations, we identify patterns and anomalies that can impact future predictions. This retrospective approach allows us to adjust our models, improving accuracy and reducing the margin of error.
One effective method is the assimilation of historical weather data into our models. This involves integrating past observations with current conditions to create a more detailed dataset. By doing so, we can better simulate potential storm scenarios and refine our predictive algorithms. Studies have shown that models incorporating historical data can greatly outperform those relying solely on real-time data, often reducing forecasting errors by up to 20%.
Additionally, leveraging machine learning techniques enables us to detect subtle correlations within vast datasets that might be missed by conventional methods. These advanced algorithms learn from historical trends and continuously update themselves, enhancing their predictive capabilities over time.
Ultimately, our goal is to provide timely, accurate storm forecasts that empower individuals and communities to make informed decisions, safeguarding lives and property.
Case Studies and Examples
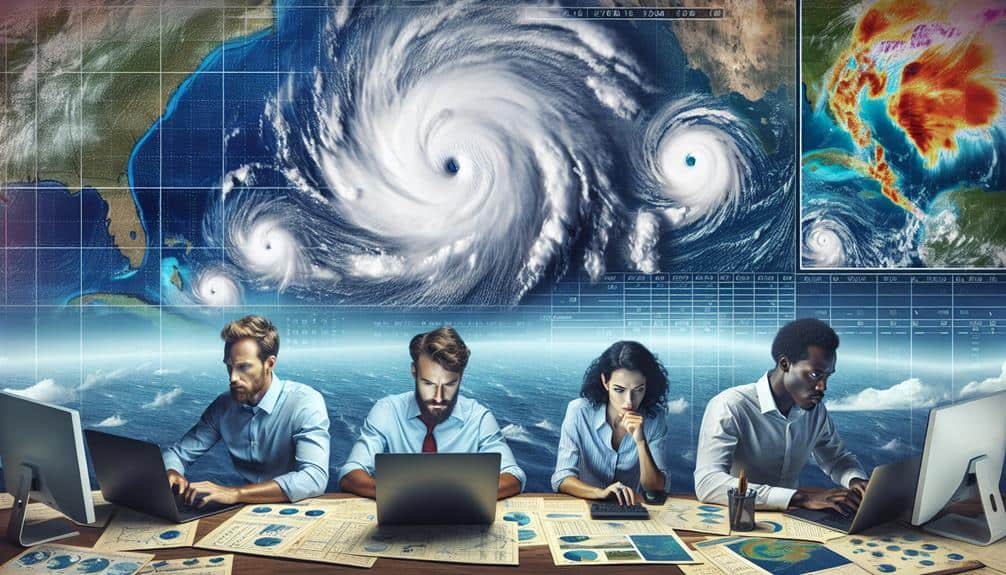
Several landmark case studies highlight the substantial enhancements in storm prediction accuracy achieved through the integration of historical data and advanced machine learning techniques. Let's immerse ourselves in some remarkable examples to illustrate this.
- Hurricane Katrina (2005): By thoroughly analyzing historical storm data, we've vastly improved our understanding of hurricane tracks and intensities. Pre-Katrina, prediction models lacked precision, leading to inadequate preparedness. Post-Katrina, incorporating historical patterns into predictive models has resulted in more precise forecasts, reducing loss of life and property.
- Typhoon Haiyan (2013): Historical data proved invaluable in understanding the rapid intensification of Typhoon Haiyan. Machine learning algorithms trained on past typhoon data provided early warning signs, allowing for timely evacuation and resource allocation. This case underscores the importance of historical data in improving the predictability of extreme weather events.
- 2017 Atlantic Hurricane Season: Leveraging decades of historical hurricane data, we've refined predictive models to forecast multiple storms with heightened accuracy. The result was a season where advanced warnings minimized the human and economic toll, showcasing the practical benefits of data-driven predictions.
These examples clearly demonstrate how historical data integration, combined with cutting-edge machine learning, enhances our ability to predict and respond to devastating storms, empowering us with the freedom to prepare and protect ourselves more effectively.
Tools for Data Analysis
Cutting-edge tools for data analysis, such as machine learning algorithms and statistical software, are essential for accurately predicting storm formations. By leveraging these technologies, we can process vast datasets, identifying patterns that might be imperceptible to the human eye. Machine learning models, trained on historical storm data, enable us to predict future storm behavior with increasing precision.
Data visualization tools play a pivotal role in this process. They transform complex datasets into intuitive visual formats, allowing meteorologists to quickly interpret trends and anomalies. Tools like Tableau and D3.js facilitate the creation of interactive maps and graphs that make storm trajectory and intensity predictions more accessible and understandable.
Statistical analysis is another cornerstone of storm prediction. Techniques such as regression analysis and time-series forecasting allow us to quantify relationships between various atmospheric variables. By applying these methods, we can establish correlations and causations that inform predictive models. For example, analyzing sea surface temperatures alongside storm formation data can reveal crucial insights into how oceanic conditions influence storm genesis.
Incorporating these advanced tools into our predictive arsenal empowers us to make data-driven decisions, enhancing our ability to forecast storms accurately. This technological synergy ultimately provides individuals and communities the freedom to prepare more effectively for impending weather events.
Future of Storm Prediction
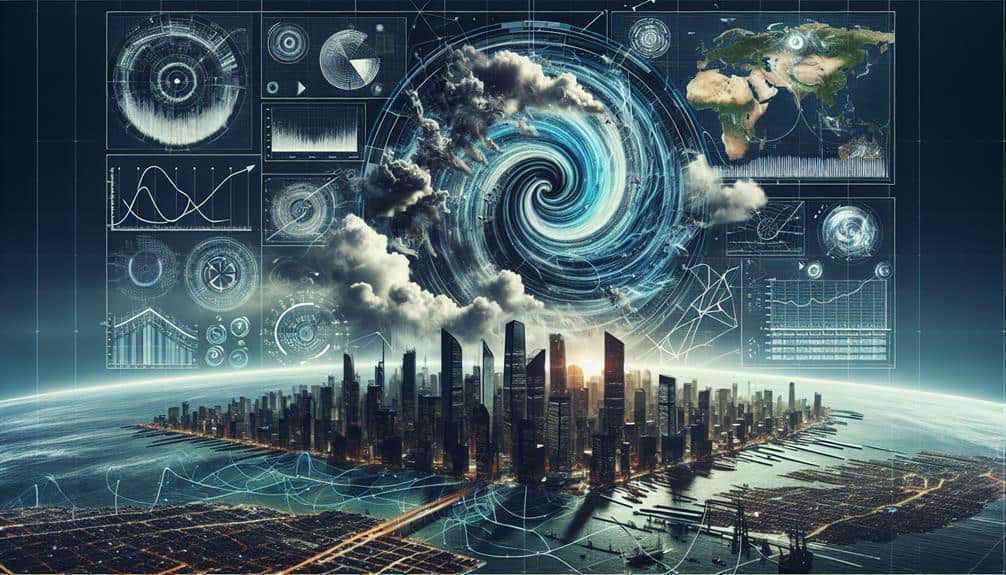
Looking ahead, the future of storm prediction hinges on integrating emerging technologies and refining existing models to improve accuracy and lead times. By leveraging data trends and technology advancements, we can revolutionize how we comprehend and respond to storm formations.
Here are three key components driving this evolution:
- Artificial Intelligence and Machine Learning: These technologies allow us to analyze vast amounts of historical and real-time data more efficiently. Machine learning algorithms can identify patterns that human analysts might miss, enhancing our predictive capabilities.
- Satellite and Remote Sensing Technologies: Advances in satellite imagery and remote sensing provide high-resolution data that enhance our understanding of atmospheric conditions. This technology offers a more detailed view of storm development, enabling quicker and more accurate forecasting.
- High-Performance Computing (HPC): HPC systems can process complex models and simulations at unprecedented speeds. This computational power allows us to run multiple scenarios and refine predictive models, resulting in better accuracy and extended lead times.
Frequently Asked Questions
How Does Climate Change Impact Storm Formation Predictions?
Oh sure, let's predict storms with a crystal ball! Seriously, climate change increases climate variability, messing with prediction accuracy. We need high-quality data and continuous algorithm refinement to keep our forecasts reliable and evidence-based.
What Are the Challenges in Collecting Historical Storm Data?
When collecting historical storm data, we face challenges like ensuring data accuracy and overcoming technological limitations. Older records often lack precision, and outdated technology hampers the reliability of data, affecting our predictive capabilities and freedom of action.
How Can Individuals Contribute to Storm Data Collection?
Citizen scientists are the lifeblood of storm data accuracy. By reporting local weather conditions through apps and networks, we can weave a rich tapestry of information that enhances predictive models, ensuring greater freedom and safety for all.
Are There Ethical Concerns in the Use of Historical Data for Predictions?
Addressing the Current Question, we must consider data privacy and algorithm bias. Ensuring ethical use involves safeguarding individuals' data and mitigating biases in predictive models to promote fairness and reliability in storm prediction efforts.
What Training Is Required for Meteorologists to Analyze Historical Storm Data?
"Knowledge is power." We need extensive meteorology training and mastery of data analysis techniques. Our education covers atmospheric science, computer modeling, and statistical methods, ensuring we can accurately interpret historical storm data and make precise predictions.