We need to start by understanding key meteorological instruments like anemometers and barometers, ensuring they are reliable and well-calibrated. Consistent data collection through standardized protocols and verification is essential. Utilizing advanced software tools enhances our ability to visualize and predict weather patterns accurately. Interpreting these patterns requires a solid grasp of atmospheric dynamics and real-time monitoring through satellites and radar systems. Analyzing historical data helps identify long-term trends, while precise prediction models improve storm trend forecasting. By mastering these techniques, we'll sharpen our meteorological analysis skills, allowing us to uncover more sophisticated insights.
Key Points
- Ensure data accuracy by regularly calibrating instruments and following standardized data collection protocols.
- Utilize advanced software tools for data visualization and predictive analysis to interpret meteorological data effectively.
- Validate collected data through robust verification processes, including automated algorithms and manual reviews.
- Analyze historical weather data to identify long-term patterns and enhance prediction accuracy.
Understand Meteorological Instruments
To accurately analyze meteorological data, we must first understand the different instruments used to measure atmospheric conditions. Precision in our data collection hinges on the reliability of meteorological technology and the accuracy of each instrument. Let's explore some key devices.
First, consider the anemometer, which measures wind speed. Its accuracy is essential for predicting weather patterns and evaluating wind energy potential.
Barometers, used to gauge atmospheric pressure, must be finely adjusted, as even slight deviations can lead to significant forecasting errors.
Thermometers, essential for temperature readings, come in various forms, including mercury, digital, and infrared. Their precision is necessary for everything from daily weather predictions to climate modeling.
Hygrometers measure humidity levels and must be kept in ideal condition to ensure accurate readings, impacting everything from agriculture to aviation.
Advanced meteorological technology, such as weather satellites and Doppler radar, offers detailed views of atmospheric phenomena. These instruments provide real-time data on cloud cover, precipitation, and storm development.
Instrument accuracy in these technologies is vital for early warning systems and disaster preparedness.
Master Data Collection Techniques
To effectively collect meteorological data, we must choose reliable instruments, guarantee data consistency, and validate the collected data.
We'll focus on selecting tools that provide accurate measurements, implementing protocols to maintain uniformity, and employing methods to verify data integrity.
These steps are essential for generating dependable and actionable insights from our meteorological analyses.
Choose Reliable Instruments
Selecting dependable instruments is essential for guaranteeing the accuracy and validity of our meteorological data collection. To achieve this, we must first focus on instrument calibration. Proper calibration ensures that our devices measure atmospheric parameters accurately over time. Without calibration, even the most advanced instruments can produce misleading data, which compromises our analysis and decision-making processes.
Next, we must consider weather station placement. A poorly positioned weather station can lead to data skewed by local environmental factors such as buildings, trees, or bodies of water. We need to position our stations in locations that represent the true atmospheric conditions of the area we're studying. Ideal placements are typically in open fields away from obstructions, at standard heights above ground, and in locations that meet World Meteorological Organization (WMO) guidelines.
Additionally, the choice of instruments themselves is vital. We should opt for devices with proven reliability and accuracy, considering factors like sensor longevity and maintenance requirements. Investing in high-quality instruments from reputable manufacturers will save us from future inaccuracies and costly replacements.
Ensure Data Consistency
Ensuring data consistency hinges on mastering precise data collection techniques that minimize variability and error. To achieve this, we must implement standardized protocols, ensuring accuracy across our data sets. By calibrating our instruments regularly, we maintain quality in our measurements, reducing discrepancies caused by equipment wear or environmental factors.
We should establish a rigorous schedule for data collection, adhering to the same times and conditions whenever possible. This consistency helps to eliminate biases introduced by varying external conditions. For example, taking temperature measurements at dawn and dusk daily provides comparable data points that are less influenced by transient weather phenomena.
Additionally, using redundant systems or parallel measurements allows us to cross-verify data, further ensuring accuracy. When discrepancies arise, we can investigate and correct anomalies promptly, maintaining the integrity of our data.
Moreover, meticulous documentation of our methods and any deviations from the standard protocol is essential. This transparency enables us to trace errors back to their source and refine our techniques continually.
Validate Collected Data
We must rigorously validate collected data to guarantee its dependability and accuracy. First, we need to implement robust data verification processes. This involves cross-checking our data against multiple sources and ensuring consistency across datasets. By doing so, we enhance data accuracy and mitigate potential disparities.
Quality control is another critical component. We should employ automated algorithms to detect anomalies and flag outliers. These tools not only streamline our workflow but also uphold data integrity by identifying errors that might otherwise go unnoticed.
Manual reviews are equally important; they offer a nuanced understanding that automated systems may lack, allowing us to correct subtler inaccuracies.
Additionally, we must validate our data collection instruments. Calibration of sensors and regular maintenance schedules are essential to ensure the equipment's best performance. By maintaining high-quality instruments, we strengthen the foundation of our data integrity.
Utilize Advanced Software Tools
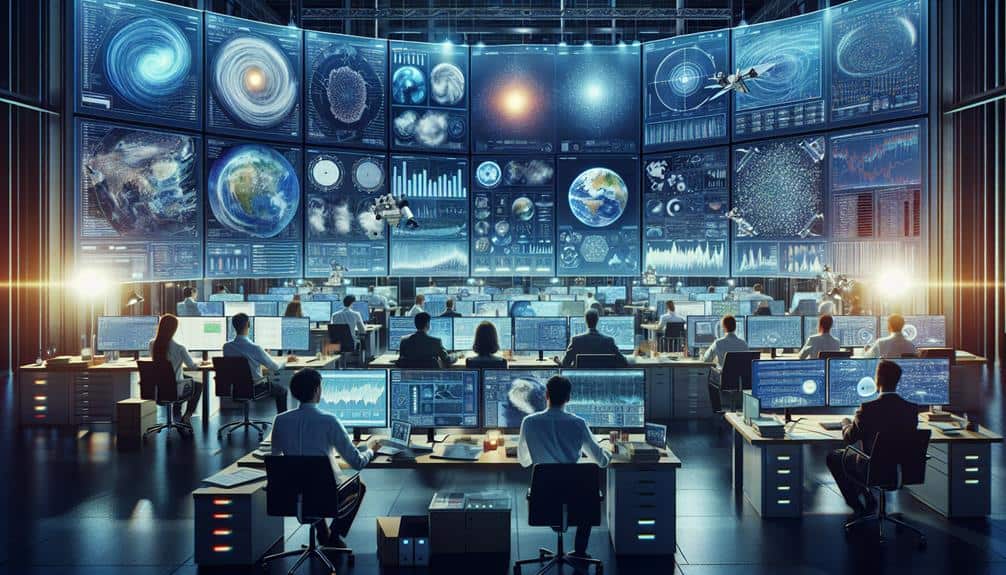
We must leverage advanced software tools to enhance our analysis of meteorological data.
By employing data visualization techniques and predictive analysis tools, we can gain deeper insights and make more accurate forecasts.
These tools enable us to interpret complex datasets efficiently and identify patterns that mightn't be immediately apparent.
Data Visualization Techniques
Using advanced software tools, we can transform raw meteorological data into detailed visualizations that reveal key patterns and trends. By employing color coding techniques, we enhance the clarity and interpretability of our graphs. Customizing these graphs enables us to highlight specific data points or anomalies, providing a deeper understanding of weather phenomena. For example, distinguishing temperature ranges with distinct colors makes it easier to identify heat waves or cold spells.
Interactive dashboards are another potent tool in our arsenal. They allow us to manipulate and explore data dynamically, offering real-time insights and facilitating better decision-making. By integrating spatial analysis, we can overlay meteorological data on geographic maps, pinpointing regions affected by specific weather events. This spatial context is essential for identifying patterns that might be overlooked in tabular formats.
Moreover, advanced software tools support a variety of visualizations, from time-series graphs to heat maps and scatter plots. Each type of visualization serves a unique purpose, helping us to analyze different aspects of the data comprehensively. By combining these techniques, we create a robust framework for understanding and interpreting meteorological data, empowering us to anticipate and respond to weather-related challenges effectively.
Predictive Analysis Tools
Predictive analysis tools, employing advanced software, enable us to forecast weather patterns with remarkable precision. By leveraging machine learning algorithms, we can process vast datasets to identify intricate patterns and trends. These algorithms learn from historical data, continually refining their predictive capabilities. This iterative process guarantees our predictions remain strong and adaptable to changing climatic conditions.
Statistical modeling plays a vital role in our predictive analysis arsenal. Techniques such as regression analysis and time-series forecasting allow us to quantify relationships between different meteorological variables. By understanding these relationships, we can create models that predict future weather events with a high level of confidence. These models are especially valuable for anticipating extreme weather events and mitigating their potential impact.
We utilize advanced software tools like Python and R, which offer extensive libraries for machine learning and statistical modeling. These tools enable us to automate data processing and model building, thereby increasing efficiency and accuracy. Moreover, cloud computing platforms give us the computational power needed to handle large-scale meteorological data.
Interpret Weather Patterns Accurately
Interpreting weather patterns accurately requires a deep understanding of atmospheric dynamics and the ability to analyze diverse meteorological data sources. To achieve precision in weather forecasting, we must integrate knowledge of climate change impacts with real-time and historical data. By doing this, we can discern subtle shifts in weather patterns that often go unnoticed.
First, we need to grasp the fundamental principles governing atmospheric behavior. Understanding how variables like temperature, humidity, and wind interact allows us to predict potential weather developments. We should also be adept at using advanced meteorological tools and models that simulate these interactions under varying conditions, including those influenced by climate change.
Furthermore, analyzing satellite imagery, radar data, and surface observations provides a multi-dimensional view of the atmosphere. This holistic perspective helps us pinpoint anomalies and emerging trends. We should also be vigilant about the accuracy of our data sources, ensuring they're both reliable and up-to-date.
Monitor Real-Time Data Effectively
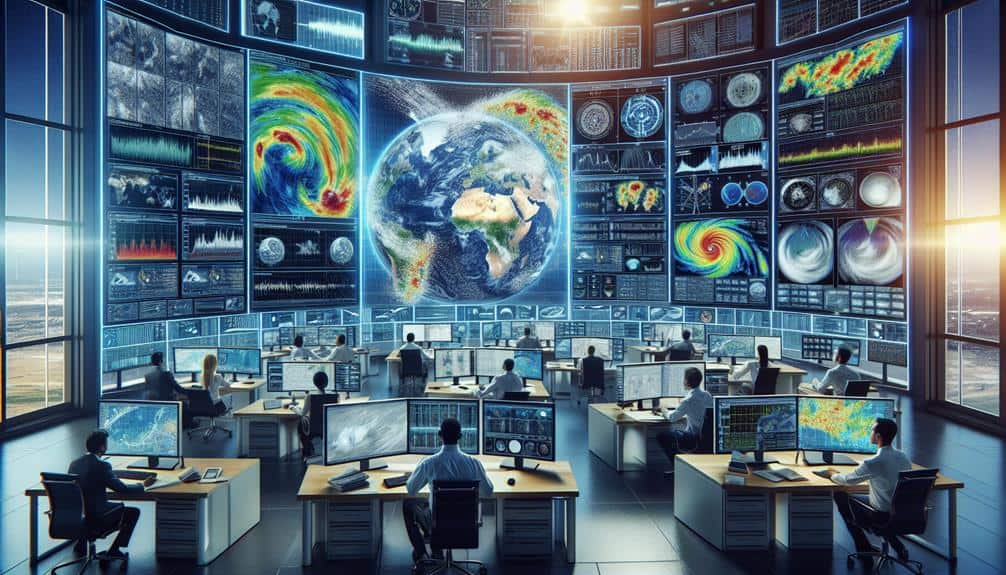
To monitor live data effectively, we must use cutting-edge technology and robust data integration techniques to capture and analyze atmospheric conditions continuously. By leveraging high-resolution satellites, advanced radar systems, and ground-based sensors, we can obtain thorough data streams essential for accurate weather forecasting. Integrating these diverse data sources ensures we've a detailed picture of meteorological trends as they evolve.
Live monitoring is critical for timely data interpretation. Automated systems can process vast amounts of data, identifying patterns and anomalies that could indicate significant weather events. The faster we can interpret these signals, the more responsive and adaptive our forecasting models become. This precision is crucial for issuing timely alerts and minimizing the impact of severe weather on communities.
Furthermore, using machine learning algorithms enhances our ability to predict weather patterns. These algorithms can analyze live data and adjust forecasts dynamically as new information becomes available. By continually refining our models, we stay ahead of meteorological trends, providing accurate and actionable insights.
Analyze Historical Weather Data
Analyzing historical weather data allows us to identify long-term patterns and trends that inform more accurate forecasting models. By examining weather trends over decades, we can enhance our prediction accuracy, making forecasts more reliable and useful.
Historical data reveals essential insights into recurring climatic conditions, such as seasonal temperature shifts and precipitation variations. This information is crucial for creating models that predict future weather scenarios with greater precision.
In our analysis, we should focus on identifying historical patterns that indicate changes in climate over time. These patterns help us understand the broader implications of climate change, allowing us to anticipate shifts in weather behavior.
By comparing historical data with current observations, we can detect anomalies and deviations that could signify significant climatic transformations.
Leveraging advanced statistical tools and software, we can dissect vast datasets to uncover subtle trends that might otherwise go unnoticed. This rigorous approach enables us to build robust models that factor in historical weather trends, thereby enhancing our overall prediction accuracy.
Ultimately, mastering the analysis of historical weather data empowers us to make informed decisions, ensuring we respond proactively to both everyday weather variations and long-term climate shifts.
Predict Storm Trends Precisely
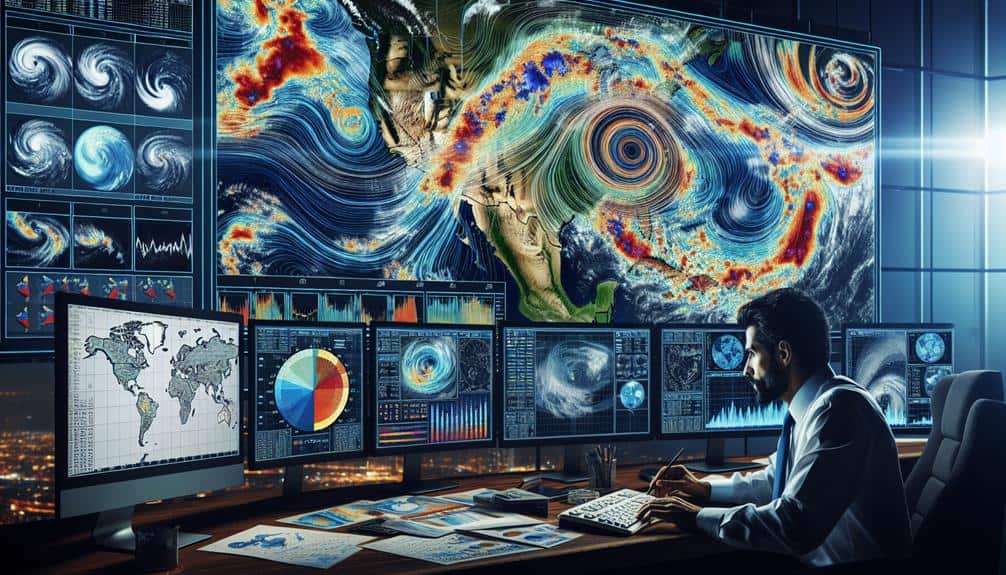
By leveraging high-resolution meteorological data and advanced predictive algorithms, we can precisely forecast storm trends with remarkable accuracy. The key to successful storm tracking and prediction lies in our ability to synthesize vast datasets from satellite imagery, radar systems, and ground-based observations. High-resolution data allows us to identify minute changes in atmospheric conditions, which are crucial for early and accurate storm forecasting.
When conducting weather pattern analysis, we need to take into account variables such as humidity, temperature gradients, wind speeds, and pressure systems. These factors interact in complex ways, and our predictive algorithms must accommodate this intricacy. Machine learning models, trained on historical storm data, help us recognize patterns and anomalies that precede storm formation and intensification. The result is a more refined and reliable storm prediction.
Additionally, real-time data assimilation enhances our forecasting capabilities. Continuous updates from observational networks guarantee that our models reflect the current state of the atmosphere, leading to more precise storm trend forecasts.
Frequently Asked Questions
How Can I Ensure Data Accuracy in Adverse Weather Conditions?
We guarantee data accuracy in adverse weather conditions by handling outliers meticulously and conducting rigorous statistical validation. Let's apply sophisticated algorithms to filter anomalies and consistently cross-verify with trusted data sources to maintain precision and reliability.
What Are the Ethical Considerations in Meteorological Data Analysis?
70% of data breaches involve sensitive information. We must prioritize data privacy while analyzing meteorological data. We should also detect and mitigate bias to secure accurate, fair outcomes. Let's respect ethical standards for better, unbiased insights.
How Do I Handle Data Gaps or Missing Values in My Dataset?
When handling data gaps or missing values, we should address outliers and use imputation techniques. Identifying outliers guarantees accurate imputation, promoting data integrity and analytical freedom. Techniques like mean substitution or regression imputation can be effective.
What Role Does Machine Learning Play in Modern Meteorology?
Machine learning's pivotal in modern meteorology. We leverage it for data interpretation, model training, and algorithm optimization. This enhances forecast accuracy, empowering us to understand weather patterns better and make informed, decisive actions.
How Can I Visualize Complex Meteorological Data Effectively for Presentations?
We can visualize complex meteorological data effectively for presentations by leveraging advanced data visualization tools like heat maps, 3D models, and interactive graphs. These techniques guarantee clarity and engagement, making our data-driven insights compelling and accessible.