Outliers in meteorological data are essential for us storm chasers because they often indicate severe weather events that standard forecasting models might miss. These anomalies can arise from unusual weather phenomena and require sophisticated algorithms and statistical models to detect. By identifying these outliers, we can enhance our predictive capabilities and provide timely warnings. Real-time data analysis and advanced visualization techniques help us pinpoint potential storm activity, ensuring our safety and accuracy in forecasting. This process not only improves our response to hazardous conditions but also refines our storm tracking methodologies further.
Key Points
- Outliers can signal the onset of severe weather events, crucial for storm chasers' timely interventions.
- Identifying outliers helps in refining predictive models for more accurate storm forecasting.
- Outliers provide early warnings, enhancing safety measures for storm chasers in hazardous conditions.
- Analyzing outliers aids in understanding extraordinary weather phenomena, improving storm tracking techniques.
Understanding Meteorological Outliers
Meteorological outliers are data points that deviate significantly from the expected range of weather patterns, often signaling anomalies or errors in data collection. These outliers can arise from measurement errors, sensor malfunctions, or extraordinary weather phenomena. As storm chasers, we must distinguish between genuine anomalies and erroneous data to make informed decisions. This is where data visualization and anomaly detection techniques become invaluable.
By employing data visualization, we can graphically represent complex meteorological datasets, making outliers more conspicuous. For instance, scatter plots and heat maps allow us to quickly identify deviations from the norm. When outliers are visualized, it becomes easier to spot trends and patterns that numerical data alone might obscure.
Anomaly detection, on the other hand, uses statistical methods and machine learning algorithms to automatically identify data points that diverge from established patterns. Techniques like Z-score analysis or clustering algorithms can flag potential outliers for further scrutiny. These methods enable us to sift through vast datasets efficiently, ensuring we're focused on the most pertinent data.
Together, data visualization and anomaly detection equip us with the tools needed to interpret meteorological outliers accurately, paving the way for more precise forecasting and safer storm chasing.
The Role of Data Anomalies
While analyzing weather patterns, data anomalies play a vital role in highlighting unexpected meteorological events that standard models might overlook. These anomalies, often representing deviations from typical weather behavior, can be vital for storm tracking. By identifying data anomalies, we enhance our ability to detect and comprehend sudden atmospheric shifts that could indicate the early stages of storm formation.
Anomaly detection is a sophisticated process involving advanced algorithms and statistical models. When we apply these techniques to weather forecasting, we can isolate irregular data points that suggest potential storm activity. It's not just about identifying outliers; it's about understanding their implications on weather systems. This is particularly valuable for storm chasers who rely on accurate and timely information to navigate extreme conditions safely.
Storm tracking becomes significantly more effective when we integrate data anomalies into our analysis. These outliers often indicate rapid changes in variables such as temperature, humidity, or wind speed, which are precursors to severe weather. By closely monitoring these anomalies, we can refine our predictions and provide more reliable forecasts.
Essentially, leveraging data anomalies empowers us to stay ahead of the storm, ensuring we remain informed and prepared.
Predicting Severe Weather Events
Predicting severe weather events requires the integration of real-time data, advanced modeling techniques, and continuous monitoring of atmospheric conditions. By leveraging storm tracking technology and sophisticated weather prediction models, we identify potential severe weather patterns early. This process involves analyzing outliers in meteorological data, which can signify the inception of intense weather phenomena such as tornadoes or hurricanes.
We utilize high-resolution satellite imagery, Doppler radar data, and automated weather stations to gather extensive atmospheric information. These data sources allow us to detect anomalies that traditional methods might overlook. For instance, sudden shifts in barometric pressure or unexpected wind shear can indicate the formation of a severe storm.
Our predictive models integrate these outliers to refine weather prediction accuracy. By continuously updating our models with real-time data, we can forecast storms with greater precision, thereby providing timely warnings. This proactive approach not only enhances our storm tracking capabilities but also empowers communities to take necessary precautions.
In essence, the meticulous analysis of meteorological data, particularly outliers, is essential in our quest to predict severe weather events. It allows us to stay ahead of the storm, ensuring both safety and freedom for those in its path.
Enhancing Forecast Accuracy
To enhance forecast accuracy, we must systematically identify extreme weather patterns that skew predictive models.
By integrating advanced algorithms and high-resolution datasets, we can refine our predictive capabilities to a great extent.
Our approach prioritizes real-time data assimilation and machine learning techniques to improve model precision and reliability.
Identifying Extreme Weather Patterns
Accurately identifying extreme weather patterns requires advanced algorithms and high-resolution meteorological data to enhance forecast accuracy. When we utilize advanced storm tracking systems, we can better understand and predict extreme conditions.
Pinpointing weather anomalies involves parsing vast datasets with precision, allowing us to refine our forecasting techniques. By leveraging high-resolution satellite imagery, Doppler radar, and ground-based sensors, we create a detailed picture of atmospheric dynamics.
Our ability to identify outliers in meteorological data enhances our storm tracking capabilities. Outliers often signify significant deviations from typical weather patterns, which can indicate the onset of severe storms or other extreme conditions. By closely monitoring these anomalies, we gain valuable insights into the development and progression of storms.
This data-driven approach enables us to issue timely warnings and take preemptive actions to mitigate potential impacts. Advanced algorithms are essential for analyzing the complexity of meteorological data. They help us filter noise from valuable signals, ensuring our forecasts are both accurate and actionable.
Improving Predictive Models
We improve forecast accuracy by integrating machine learning algorithms that analyze high-resolution meteorological data. By doing so, we can capture outliers more effectively, which are essential in identifying extreme weather events. Leveraging these algorithms allows us to enhance model accuracy, helping us predict storm paths and intensities with greater precision. Machine learning models excel at recognizing patterns and anomalies in vast datasets, making them indispensable for storm chasers.
Furthermore, data visualization plays a significant role in this process. We utilize advanced visualization techniques to interpret complex meteorological data, transforming it into understandable formats. These visual tools enable us to quickly identify outliers that might indicate severe weather conditions. By visualizing data, we not only improve our predictive models but also facilitate real-time decision-making during storm chasing expeditions.
Incorporating outliers into our predictive models ensures that we don't overlook crucial data points that could impact model accuracy. This thorough approach empowers us to make informed decisions, granting us the freedom to anticipate and respond to extreme weather with confidence. As storm chasers, our ability to accurately predict and visualize storm behavior is paramount, and leveraging outliers in meteorological data is essential to achieving this.
Real-Time Data Analysis
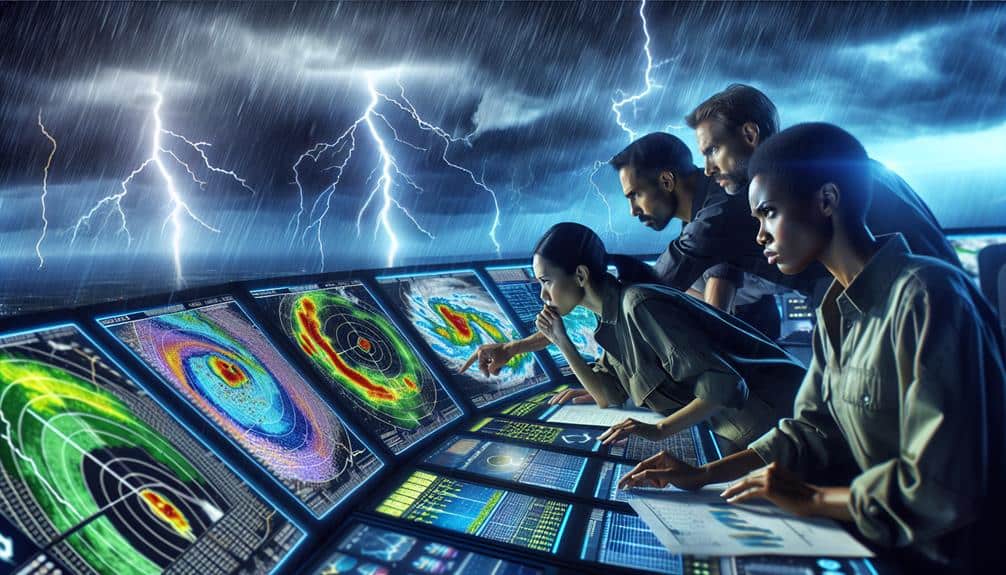
Real-time data analysis in meteorology necessitates robust algorithms capable of swiftly identifying and addressing outliers to ensure the accuracy and reliability of forecasts. We leverage advanced data visualization techniques and anomaly detection systems to scrutinize incoming meteorological data streams. By pinpointing outliers, we can better comprehend and forecast storm behavior, providing us with a pivotal edge in the field.
Utilizing machine learning models, we engage in rigorous outlier analysis to detect deviations from expected weather patterns. These models, trained on vast datasets, can discern subtle anomalies that might elude traditional methods. Our algorithms operate with high precision, ensuring that each data point is meticulously evaluated in real-time.
This real-time capability empowers us with the freedom to make informed decisions on the fly, enhancing our ability to track and respond to volatile weather conditions. By integrating machine learning and data visualization, we can't only detect outliers but also interpret their significance within the broader meteorological context.
Harnessing these technologies allows us to stay agile and adaptive, essential for storm chasers who need to navigate rapidly changing environments. Real-time data analysis thereby becomes an indispensable tool in our quest to understand and anticipate the unpredictable nature of storms.
Improving Safety Measures
Integrating advanced outlier detection techniques into meteorological systems can greatly enhance safety measures by providing timely warnings and actionable insights. When we identify outliers in weather data, we can improve our risk assessment processes to a large extent. These outliers often indicate severe weather anomalies that traditional forecasting models might overlook. By recognizing these anomalies early, we can better prepare for extreme events, reducing the risk to life and property.
For storm chasers, timely detection of outliers is essential. It allows us to anticipate hazardous conditions and adjust our plans accordingly. Advanced algorithms can flag unexpected shifts in wind patterns, temperature drops, or pressure changes, which are often signs of severe storms. This data-driven approach enhances our ability to predict and respond to emergencies more effectively.
Furthermore, integrating these techniques into our emergency response protocols increases our operational efficiency. When we're aware of the precise location and potential impact of a weather anomaly, we can deploy resources more strategically and provide accurate, real-time updates to the public. This guarantees that both storm chasers and the communities we serve are better protected against unforeseen severe weather events.
Tools for Identifying Outliers
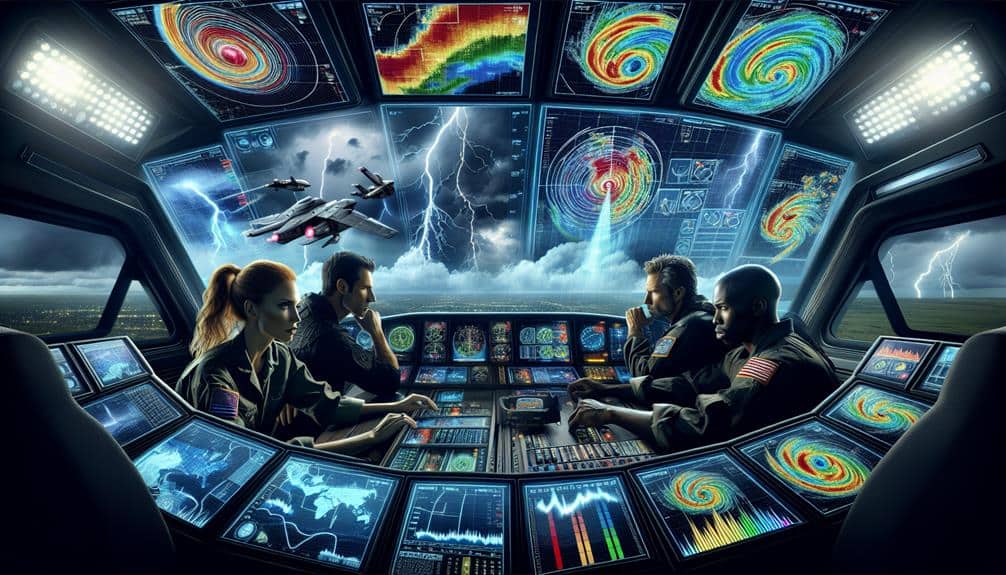
Harnessing advanced algorithms and statistical methods, we can pinpoint outliers in meteorological data with remarkable accuracy, thereby enhancing our predictive capabilities. By utilizing tools designed for statistical analysis and data visualization, we enable storm chasers to make informed decisions. Identifying outliers is vital for comprehending extreme weather events and anticipating storm behavior.
Here are some powerful tools we rely on:
- R and Python: These programming languages offer robust libraries like Pandas and NumPy for statistical analysis and Matplotlib and Seaborn for data visualization.
- Machine Learning Models: Algorithms such as Isolation Forest, DBSCAN, and K-means clustering help detect anomalies in large datasets.
- GIS Software: Geographical Information Systems (GIS) like ArcGIS provide spatial analysis capabilities essential for visualizing meteorological data.
- Meteorological Workstations: Advanced systems like AWIPS (Advanced Weather Interactive Processing System) integrate various data sources, offering real-time visualization and analysis capabilities.
Frequently Asked Questions
How Do Storm Chasers Select Their Target Locations?
We select our target locations through meticulous target analysis, focusing on weather patterns. By interpreting data and utilizing storm prediction models, we identify ideal chase zones, ensuring we maximize our freedom to pursue and document severe weather events.
What Qualifications Are Needed to Become a Professional Storm Chaser?
To become professional storm chasers, we need meteorology expertise, experience, and proficiency in tracking technology. We must follow strict safety protocols to navigate severe weather conditions effectively while ensuring our freedom to chase storms doesn't compromise our safety.
What Equipment Do Storm Chasers Typically Use in the Field?
We use advanced technology, like Doppler radar and anemometers, to monitor weather patterns. GPS systems and laptops aid in data collection and forecasting. This equipment helps us accurately predict storm behavior and stay safe in the field.
How Do Storm Chasers Stay Safe During Severe Weather Events?
How do we stay safe during severe weather events? We practice emergency preparedness and safety precautions rigorously, use advanced tracking devices, and implement robust communication strategies to guarantee real-time data sharing and coordinated responses.
Are There Any Famous Storm Chasing Teams or Individuals?
We've all heard of famous storm chasing teams like the Tornado Intercept Vehicle crew, featured in storm chasing documentaries. They utilize cutting-edge storm chaser technology, including Doppler radar and drones, to capture invaluable meteorological data safely.