We depend on interdisciplinary approaches for hailstorm research because they integrate critical insights from meteorology, climatology, and advanced data analytics. By combining vertical wind profiles, temperature gradients, and humidity levels from radar systems and satellites, we enhance our understanding of convective processes that form hailstones. Analyzing historical weather patterns and incorporating machine learning algorithms into predictive models allows us to simulate future hailstorm scenarios with greater accuracy. Additionally, integrating social science perspectives improves community resilience by refining early warning systems. Through these methods, we can better predict and mitigate the impacts of severe hailstorms, leading to more effective strategies.
Key Points
- Interdisciplinary approaches integrate meteorology, oceanography, and geoscience for comprehensive understanding of hailstorm dynamics.
- Machine learning and advanced algorithms from computer science enhance predictive accuracy and operational efficiency.
- Social sciences provide community insights, identifying vulnerabilities and improving warning systems.
- Combining diverse data sources, including sensors and satellites, refines real-time hailstorm tracking and forecasting.
Understanding Meteorological Dynamics
To fully comprehend the intricacies of hailstorm formation, we must analyze the meteorological dynamics driving convective processes and atmospheric instability. By diving into meteorological research, we can better understand how temperature gradients, humidity levels, and wind shear contribute to these phenomena.
Convective updrafts, fueled by these dynamics, lift water droplets to altitudes where they freeze and coalesce into hailstones.
In our quest for insight, we examine data from weather balloons, radar systems, and satellite observations. These tools reveal vertical wind profiles and temperature layers essential for identifying atmospheric instability.
For instance, when warm, moist air at the surface meets cooler, dry air aloft, it creates an environment ripe for vigorous convection. This convection is intensified by wind shear, which tilts the updraft, allowing the hailstones to grow larger before gravity pulls them back down.
Leveraging Climatology Insights
We can enhance our understanding of hailstorm patterns by analyzing historical weather data and leveraging predictive climate models.
By examining regional climate variability, we identify trends that inform both short-term forecasts and long-term climate predictions.
These insights enable us to develop more accurate risk assessments and mitigation strategies for hail-prone areas.
Historical Weather Patterns
Drawing from extensive climatology databases, we can identify recurring patterns and anomalies in historical hailstorm events that provide critical insights for predictive modeling.
Analyzing historical data allows us to detect trends in hailstorm frequency, intensity, and geographical distribution. These trends, when contextualized within the broader scope of climate change, reveal how shifts in atmospheric conditions are influencing hailstorm behavior.
By examining data spanning several decades, we've observed that hailstorm occurrences aren't random but exhibit periodicity. For instance, certain regions experience heightened activity during specific seasons, correlating with variations in temperature and humidity.
Additionally, climate change has introduced new variables, altering traditional patterns. Warmer global temperatures have intensified atmospheric instability, thereby increasing the frequency and severity of hailstorms in previously unaffected areas.
Our analysis highlights the importance of integrating historical weather patterns with contemporary climatological changes. This integration enables us to refine our understanding of how hailstorms evolve and adapt to changing environmental conditions.
Predictive Climate Models
Leveraging climatology insights, predictive climate models allow us to simulate future hailstorm scenarios with improved accuracy and granularity. By integrating vast datasets from historical weather patterns, atmospheric conditions, and current climate trends, we can refine these models to pinpoint hailstorm events with unprecedented precision. This isn't just about forecasting; it's about enhancing our decision-making efficiency.
We utilize advanced algorithms and machine learning techniques to analyze and process climatological data, leading to models that adapt and learn over time. As we input more data, the models' predictive capabilities improve, resulting in higher accuracy rates. This iterative process helps us anticipate the likelihood, timing, and intensity of hailstorms, reducing economic impacts and potential harm.
Moreover, these models offer us the freedom to explore various what-if scenarios, adjusting for different variables such as temperature shifts and atmospheric pressure changes. This flexibility is essential for developing robust disaster preparedness strategies and optimizing resource allocation.
Incorporating interdisciplinary methodologies, we can further enhance our predictive models. By continuously updating our algorithms and integrating new climatological insights, we're not just improving accuracy but also enhancing operational efficiency, ultimately empowering communities to better withstand the impacts of severe weather events.
Regional Climate Variability
Regional climate variability, driven by complex interactions between atmospheric, oceanic, and terrestrial systems, greatly influences the frequency and intensity of hailstorm events. By leveraging climatology insights, we can better understand how these factors interplay, particularly in the context of climate change.
For instance, warmer sea surface temperatures can alter atmospheric circulation patterns, leading to more extreme events, including severe hailstorms.
Analyzing historical climate data allows us to identify regional trends and anomalies. By correlating these patterns with hailstorm occurrences, we can pinpoint regions at higher risk.
For example, we've observed that areas experiencing increased temperature variability also report higher incidences of large hail. This correlation underscores the need for localized climate models to predict and mitigate hailstorm impacts effectively.
Our interdisciplinary approach incorporates data from meteorology, oceanography, and geoscience, providing a comprehensive understanding of how regional climate variability shapes extreme weather events. By doing so, we enable communities to make informed decisions, enhancing resilience against future hailstorms.
As climate change continues to evolve, understanding these regional dynamics becomes paramount, empowering us to develop adaptive strategies and safeguard our freedom to thrive in increasingly unpredictable environments.
Engineering Robust Data Collection
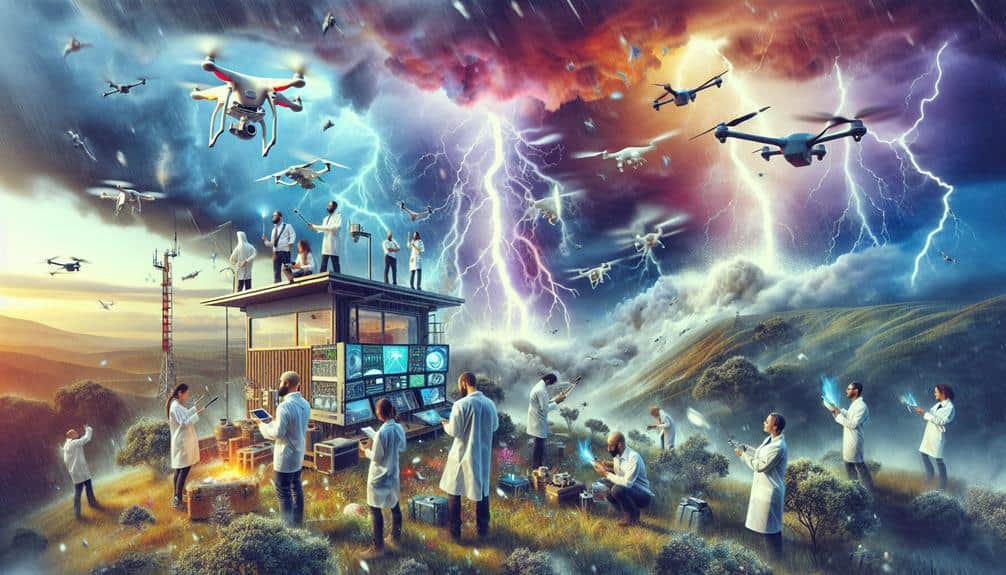
To engineer robust data collection systems for hailstorm research, we must integrate advanced sensor technologies with real-time data analytics. Remote sensing provides a pivotal tool for capturing large-scale atmospheric data, enabling us to track storm development and hail formation from space. Using high-resolution satellites, we can monitor cloud structures, temperature gradients, and precipitation patterns with unprecedented precision.
Field observations complement these remote sensing capabilities by offering ground-truth data critical for validating and refining satellite-based measurements. Deploying an array of ground-based sensors, such as disdrometers and radar systems, allows us to measure hail size, density, and distribution directly. These instruments provide granular, real-time data that remote sensors might miss due to their broader focus.
Utilizing Geographic Information Systems
Let's integrate Geographic Information Systems (GIS) to enhance our hailstorm research.
By leveraging spatial data analysis and mapping hailstorm patterns, we can identify high-risk areas with greater accuracy.
Additionally, predictive modeling techniques in GIS will enable us to forecast future hailstorm events more effectively.
Spatial Data Analysis
Harnessing the power of Geographic Information Systems (GIS), we can effectively analyze spatial patterns and distributions of hailstorm occurrences to enhance predictive models and risk assessments. Geospatial analytics and remote sensing technologies enable us to gather, process, and interpret vast amounts of spatial data. By integrating these tools, we uncover critical insights that drive more accurate forecasting models and informed decision-making.
To explore deeper, consider these three pivotal aspects:
- Geospatial Analytics: By employing geospatial analytics, we can identify hotspots and trends in hailstorm activity. These insights help us understand the underlying factors contributing to hailstorm development and propagation.
- Remote Sensing: Utilizing remote sensing technologies, we can capture high-resolution imagery and data from satellites and airborne sensors. This allows us to monitor atmospheric conditions and surface changes in near real-time, providing a dynamic view of hailstorm evolution.
- Spatial Data Integration: Integrating various spatial datasets, such as topography, land use, and meteorological data, enhances our predictive capabilities. This holistic approach ensures that our models account for the complex interplay of factors influencing hailstorm behavior.
Mapping Hailstorm Patterns
By employing Geographic Information Systems (GIS), we can map hailstorm patterns with precision, revealing spatial distributions and temporal trends that are critical for enhancing predictive accuracy. GIS technology allows us to integrate diverse datasets, including satellite imagery and ground-based observations, to construct detailed maps that display the frequency, intensity, and duration of hailstorms over specified regions.
Utilizing satellite imagery, we capture high-resolution data that provides a bird's-eye view of affected areas. This data is then processed using machine learning algorithms to identify and classify hailstorm events accurately. Machine learning enhances our ability to analyze vast amounts of data quickly, detecting patterns and anomalies that traditional methods might overlook.
By layering temporal data, we can observe how hailstorm activity evolves over time, offering insights into seasonal variations and long-term trends. This historical data is invaluable for identifying regions with high hailstorm recurrence, thereby informing risk assessments and mitigation strategies.
Incorporating GIS into our hailstorm research empowers us to make data-driven decisions. Enhanced mapping capabilities not only improve our understanding of hailstorm dynamics but also provide the freedom to develop more effective and targeted responses to these natural phenomena.
Predictive Modeling Techniques
Predictive modeling techniques utilizing Geographic Information Systems (GIS) enable us to forecast hailstorm occurrences with increased accuracy by integrating multiple data sources and sophisticated algorithms. By leveraging GIS, we can synthesize spatial and temporal data, which provides a thorough framework for analyzing and predicting hailstorm patterns.
To enhance our predictive capabilities, we employ:
- Machine Learning Algorithms: These algorithms analyze historical weather data, identifying patterns and trends that traditional statistical methods might miss. By training these models on vast datasets, we can forecast future hailstorm events with higher precision.
- Statistical Analysis: Complementing machine learning, statistical analysis helps validate our models. Techniques such as regression analysis and time-series forecasting enable us to quantify the relationship between various climatic variables and hailstorm occurrences.
- Multi-Source Data Integration: Combining satellite imagery, ground-based weather stations, and radar data allows us to create a more detailed and accurate model. This integration helps us pinpoint potential hailstorm zones and predict their development in real-time.
Enhancing Predictive Models
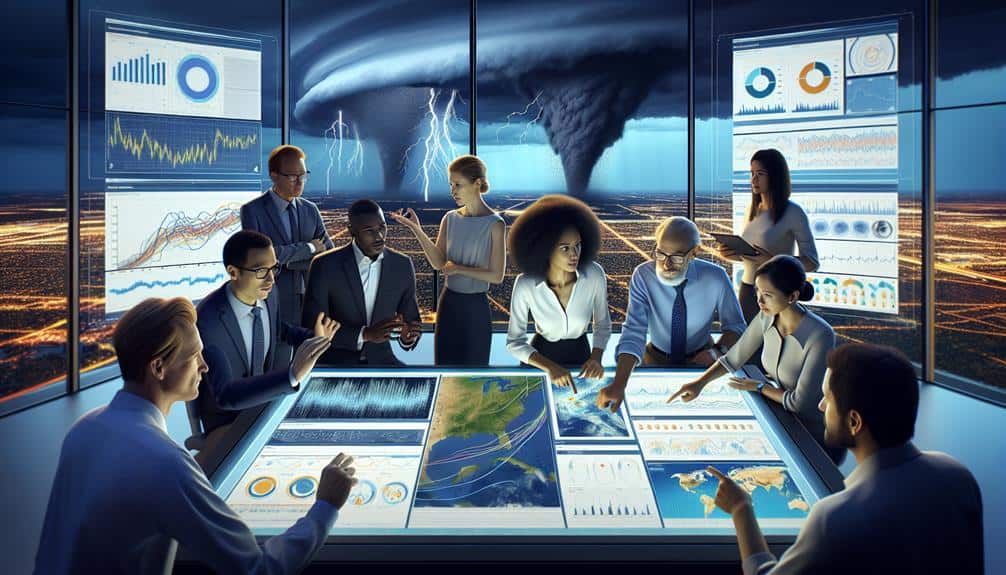
To enhance predictive models for hailstorms, we must integrate high-resolution atmospheric data with advanced machine learning algorithms. By leveraging machine learning, we can process vast amounts of meteorological data, identify patterns, and improve the accuracy of our predictions.
High-resolution data, such as satellite imagery and radar observations, provide the granularity needed to capture the nuances of hailstorm formation and evolution. This data serves as the foundation for training machine learning models, allowing them to learn from past events and make informed predictions about future occurrences.
In addition to machine learning, robust statistical analysis is essential for validating our models and ensuring their reliability. Statistical methods enable us to assess the performance of predictive algorithms, identify biases, and quantify uncertainties. By combining statistical rigor with the computational power of machine learning, we can develop models that aren't only accurate but also adaptable to changing atmospheric conditions.
Our goal is to create predictive models that empower individuals and communities to make proactive decisions, minimizing the impact of hailstorms. By adopting an interdisciplinary approach, we can harness the strengths of various scientific domains and push the boundaries of what's possible in meteorological forecasting.
Integrating Social Sciences
While enhancing predictive models improves our technical capacity to forecast hailstorms, integrating social sciences provides a thorough understanding of how communities perceive, prepare for, and respond to these severe weather events. We must consider community engagement and cultural perspectives to create effective mitigation strategies.
To attain a detailed analysis, we should focus on:
- Community Engagement: Engaging with communities directly helps us gather qualitative data on local beliefs and practices regarding hailstorms. This data informs tailored communication strategies.
- Cultural Perspectives: Understanding cultural nuances allows us to identify specific vulnerabilities and resilience factors unique to different communities. These insights are essential for designing interventions that respect cultural contexts.
- Behavioral Analysis: Examining how individuals and communities react to hailstorm warnings and events can illuminate gaps between predicted behavior and actual response. This helps refine our warning systems and educational programs.
Data-driven insights from social sciences can guide policymakers in crafting public policies that empower communities. By incorporating local knowledge and cultural perspectives, we guarantee that our approaches aren't only scientifically robust but also socially relevant. This interdisciplinary approach enhances our ability to protect lives and property, offering communities the freedom to thrive despite the threat of hailstorms.
Frequently Asked Questions
How Do Hailstorms Impact Agriculture and Crop Yields?
Hailstorms disrupt weather patterns, causing significant damage to agriculture. They can devastate crop yields, leading to economic losses. Data shows that regions frequently hit by hailstorms experience a 20-30% reduction in crop yields annually.
What Are the Economic Costs Associated With Hailstorm Damage?
Did you know hailstorms cause over $10 billion in damages annually? We face substantial economic costs, primarily from insurance coverage claims and repair costs. Effective planning and response strategies can mitigate these financial impacts.
Can Hailstorm Research Inform Building Codes and Construction Practices?
We can enhance structural resilience and risk mitigation by integrating hailstorm research into building codes and construction practices. Data-driven insights on hail impact patterns help engineers design structures that reduce potential damage and guarantee safety.
How Do Insurance Companies Assess and Manage Hailstorm-Related Risks?
In evaluating hailstorm-related risks, we prioritize accurate risk evaluation and proactive mitigation strategies. We verify insurance coverage aligns accurately, streamlining the claims process. Data-driven decisions empower clients, offering freedom through informed, resilient choices.
What Role Do Public Awareness and Education Play in Hailstorm Preparedness?
We enhance disaster resilience through community engagement and integrating weather education into the school curriculum. Data shows informed communities better prepare for hailstorms, reducing damage and recovery costs, thereby promoting individual and collective freedom.