We prioritize the accuracy and reliability of storm tracking by employing advanced meteorological data validation methods. We use calibrated ground-based observations, cross-verify satellite data, and analyze radar accuracy to guarantee precise measurements. We rigorously control wind speed and direction data, monitor temperature and humidity, and validate precipitation measurements. We maintain long-term dataset consistency by detecting and correcting anomalies. By adapting to varying environmental conditions and leveraging statistical methods, we guarantee our data's integrity. Discover how these methods enhance storm tracking efficiency and reliability.
Key Points
- Cross-reference satellite and radar data with ground-based observations to ensure accuracy in storm tracking.
- Utilize statistical methods to detect and correct anomalies in meteorological datasets.
- Implement rigorous calibration schedules and real-time monitoring for sensor accuracy.
- Employ data fusion techniques to combine multiple data sources for robust analysis.
Ground-Based Observations
Ground-based observations form the cornerstone of meteorological data validation, providing high-resolution and accurate measurements directly from the earth's surface. By deploying weather stations for verification, we guarantee the integrity of our atmospheric data. These stations meticulously measure parameters such as temperature, humidity, and wind speed, which are vital for precise weather prediction. Soil moisture monitoring is another crucial aspect, offering insights into hydrological cycles and agricultural conditions.
Remote sensing applications, while invaluable, still rely on these ground-based observations for calibration and validation. As we grapple with the far-reaching impacts of climate change, the precision afforded by ground-based methods becomes even more important. These observations serve as a check against satellite data, anchoring our models in real-world measurements.
We can't overlook the role these observations play in understanding climate change impacts. By tracking long-term trends in soil moisture and atmospheric conditions, we gain actionable insights that drive policy and adaptation strategies. Ground-based observations provide the granularity needed to capture subtle changes, empowering us with the data necessary to make informed decisions.
In a world increasingly reliant on remote sensing, these earth-bound measurements remain indispensable for guaranteeing data accuracy and reliability.
Satellite Data Cross-Verification
To maintain the accuracy of satellite data, we utilize the following methods:
- Image consistency analysis
- Temporal data comparison
Image Consistency Analysis
When validating meteorological data, we employ image consistency analysis by cross-verifying satellite data to guarantee accuracy and reliability. Our first step involves conducting an image resolution analysis. By evaluating the resolution of satellite images, we confirm that the spatial details align with the expected data granularity. Higher resolution images provide finer details, which are essential for accurate storm tracking.
Next, we utilize data fusion techniques to enhance the robustness of our analysis. Data fusion allows us to integrate information from multiple satellite sources, creating a thorough picture of atmospheric conditions. This integration not only improves the quality of the data but also helps us identify any discrepancies between different datasets.
We systematically compare these fused images to detect inconsistencies. For instance, if one satellite shows a storm path deviating significantly from others, it raises a red flag, prompting further investigation. By cross-referencing various satellite data, we mitigate the risk of relying on potentially flawed information.
Through this thorough process, we uphold the integrity of our meteorological data, ensuring that our storm tracking models are both precise and dependable. This approach empowers us to provide accurate weather forecasts, aiding in timely and informed decision-making.
Temporal Data Comparison
Building on our image consistency analysis, we now focus on temporal data comparison to cross-verify satellite data over time, ensuring the continuity and accuracy of meteorological observations. By conducting historical data comparisons, we can analyze storm patterns, identifying consistencies and anomalies that inform our understanding of storm behavior. This method allows us to detect shifts in storm patterns and validate current satellite data against historical records, providing a solid foundation for storm analysis.
In addition to historical data comparison, real-time data correlation plays a critical role in our process. By correlating real-time satellite data with previous observations, we can predict storm movements with greater accuracy. Real-time data correlation helps us identify emerging storm patterns and make timely adjustments to our models, enhancing our storm movement prediction capabilities. This approach enables us to provide more accurate and reliable meteorological forecasts, essential for public safety and disaster preparedness.
We employ sophisticated algorithms to analyze temporal data, ensuring that our findings are data-driven and precise. By integrating historical data with real-time observations, we enhance our ability to track and predict storms, offering a thorough view that empowers informed decision-making.
Sensor Accuracy Checks
Frequently, we conduct sensor accuracy checks to cross-verify satellite data and guarantee the reliability of our meteorological observations. This process involves rigorous calibration verification and systematic sensor maintenance, ensuring our equipment functions efficiently. By regularly comparing satellite readings with ground-based measurements, we can detect and rectify any discrepancies.
We employ advanced validation software to streamline our data interpretation. This software cross-references multiple data sources, highlighting inconsistencies and potential errors. With these tools, we can rapidly identify any drift in sensor calibration and implement corrective measures promptly. Such diligence is essential for maintaining the precision of our storm tracking models and forecasts.
Moreover, calibration verification isn't a one-time event but an ongoing commitment. We schedule routine sensor maintenance to prevent degradation over time, which could otherwise compromise data integrity. By staying proactive, we uphold the accuracy of our meteorological datasets, thereby enhancing our ability to predict severe weather events.
Ultimately, our meticulous approach to sensor accuracy checks empowers us to provide reliable, high-quality meteorological data. This data-driven methodology supports the freedom of informed decision-making, enabling communities to better prepare for and respond to storms.
Radar Data Accuracy
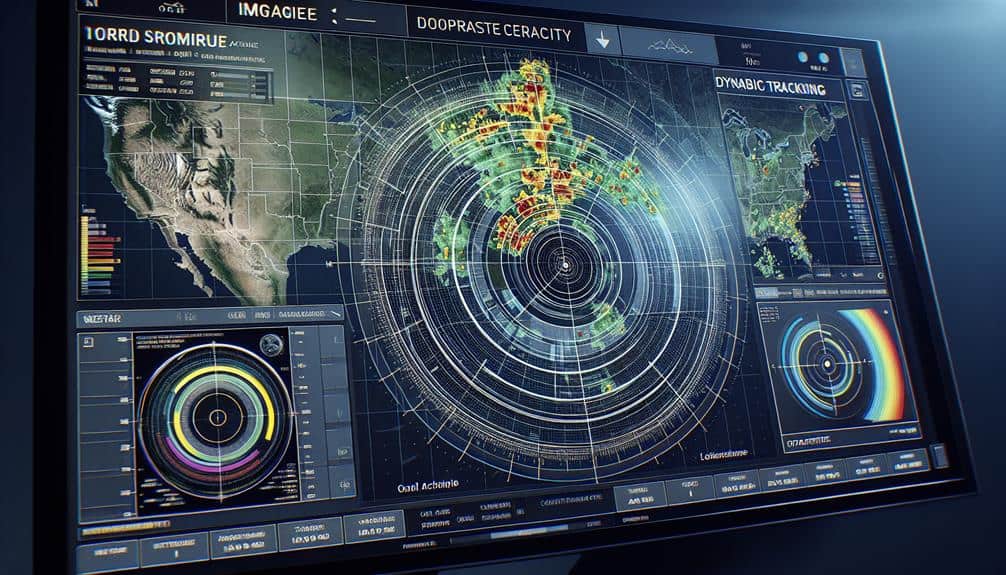
To guarantee radar data accuracy, we thoroughly analyze signal quality, calibration processes, and error sources. By conducting a detailed Doppler radar evaluation, we assess storm intensity with high precision. Our approach involves careful calibration of radar systems to ensure the reliability of the data captured. We scrutinize signal quality to detect and rectify any discrepancies that could skew our interpretation of storm movement.
We don't just stop at calibration; we also investigate error sources, identifying potential interferences that could impair data accuracy. By cross-referencing radar data with ground-truth observations, we validate the integrity of our readings. This data-driven approach allows us to make informed decisions about storm tracking and prediction.
In radar data interpretation, understanding storm movement is essential. We employ sophisticated algorithms to decode radar returns, translating them into actionable insights about storm trajectories. This process involves analyzing through vast amounts of data to highlight patterns indicative of storm developments. By doing so, we ensure our storm tracking methods aren't only precise but also responsive to real-time changes.
Our commitment to accuracy and flexibility in data analysis empowers us to provide reliable storm tracking, necessary for mitigating risks and enhancing preparedness.
Wind Speed and Direction Analysis
When analyzing wind speed and direction, we must focus on sensor calibration techniques to guarantee accurate data collection.
We also implement rigorous data quality control measures to identify and correct errors.
Additionally, anomaly detection methods help us pinpoint and address inconsistencies in the dataset.
Sensor Calibration Techniques
How do we guarantee the accuracy of wind speed and direction measurements? To safeguard our data integrity, we must employ rigorous sensor calibration techniques. Calibration error analysis and sensor drift detection are critical processes in preserving the accuracy of our meteorological readings. By systematically identifying and correcting discrepancies, we enhance our storm tracking capabilities.
Let's break down our approach:
- Regular Calibration Schedules: Adhering to a strict calibration timetable ensures that sensors maintain their precision over time.
- Real-Time Monitoring: Implementing continuous monitoring systems to detect any deviations in sensor performance promptly.
- Reference Comparisons: Comparing sensor data with trusted reference instruments to identify any calibration errors.
- Environmental Adjustments: Adjusting for environmental factors such as temperature and humidity that can affect sensor accuracy.
Data Quality Control
By implementing strict data quality control measures, we guarantee the reliability and accuracy of our wind speed and direction readings. We employ robust data validation techniques to scrutinize every data point collected. This process includes real-time error detection algorithms that identify anomalies and inconsistencies in the data. By systematically analyzing wind speed and direction, we confirm that each reading meets our stringent criteria for accuracy.
Our data quality assessment involves multiple verification methods. First, we cross-reference readings from different sensors to detect any discrepancies. This comparative analysis helps in identifying potential sensor malfunctions or calibration drifts. Additionally, we use statistical methods to evaluate the data distribution and identify outliers that could indicate measurement errors.
Verification methods don't stop at data collection. We also perform historical data comparisons to detect trends and validate current readings against established baselines. This longitudinal analysis provides an additional layer of error detection, ensuring that our data remains consistent over time.
Anomaly Detection Methods
To improve our data quality control measures, we utilize advanced anomaly detection methods to carefully analyze wind speed and direction data. By leveraging statistical analysis and machine learning algorithms, we can identify outliers and inconsistencies that may jeopardize the integrity of our meteorological datasets.
Our approach includes:
- Data visualization: Visual tools help us map wind patterns, making it easier to identify anomalies.
- Pattern recognition: We use sophisticated algorithms to detect irregularities in wind speed and direction data.
- Statistical thresholds: These define acceptable data ranges, flagging values that deviate significantly.
- Machine learning models: These models learn from historical data, enhancing their ability to detect anomalies over time.
Each of these methods offers unique advantages, allowing us to uphold high standards of data quality.
By integrating statistical analysis with machine learning algorithms, we improve our ability to track and respond to storm-related events with precision. Moreover, our data visualization techniques and pattern recognition tools provide clear, actionable insights, empowering us to make informed decisions swiftly.
This thorough approach ensures that our storm tracking is both accurate and dependable, ultimately supporting our dedication to delivering high-quality meteorological data.
Temperature and Humidity Checks
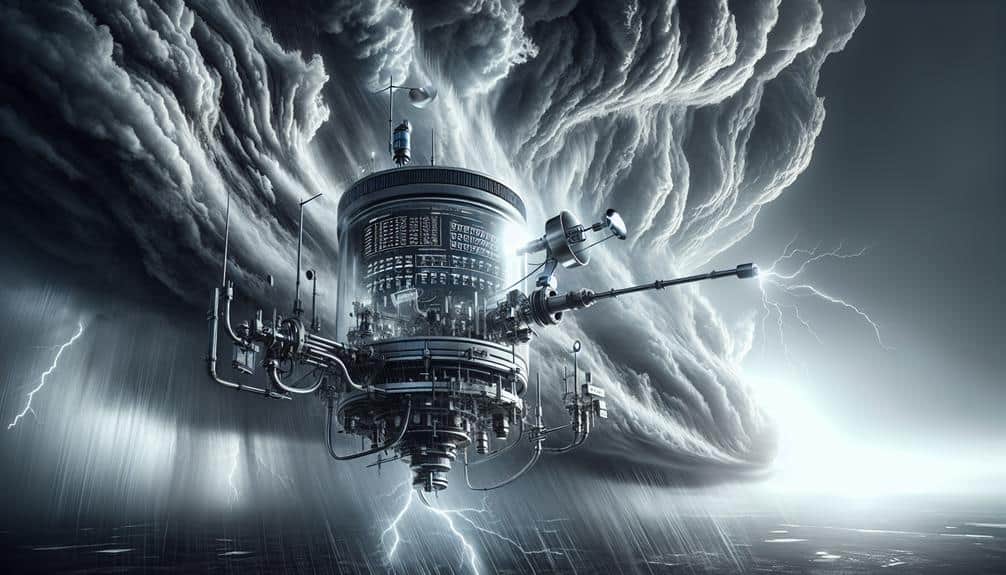
We frequently rely on automated and manual methods to verify the accuracy of temperature and humidity data collected from meteorological stations. Maintaining data integrity is essential for reliable storm tracking, so we employ rigorous data integrity checks and sensor validation protocols. Automated systems continuously perform real-time monitoring to detect anomalies and confirm each sensor is operating within specified parameters.
Manual methods supplement these automated processes, wherein field technicians periodically inspect and calibrate the sensors. This dual approach guarantees that any discrepancies are promptly addressed, thereby preserving the reliability of our temperature and humidity readings. By cross-referencing data from multiple sensors and using redundancy, we can identify and correct potential errors.
Real-time monitoring is vital for enhancing weather forecasting accuracy. Continuous data streams allow us to make timely adjustments to our models, which directly impacts the precision of storm predictions. High-quality temperature and humidity data, validated through these rigorous checks, provide the foundation for accurate weather forecasting. In turn, this empowers us to deliver timely warnings and actionable insights, catering to an audience that values the freedom to make informed decisions based on accurate, real-time weather data.
Precipitation Measurement Validation
Accurate precipitation measurement validation is another critical aspect of maintaining the integrity of meteorological data. When we assess data accuracy for storm tracking, precise precipitation analysis is paramount. We utilize several methods to make sure that our measurements are both valid and reliable.
To enhance our measurement validation, we focus on the following key techniques:
- Cross-referencing multiple data sources: We compare precipitation data gathered from different sensors, such as rain gauges, radar systems, and satellite observations, to identify discrepancies.
- Calibration of instruments: Regular calibration of rain gauges and other precipitation measurement tools helps us maintain high data accuracy.
- Data quality control algorithms: Implementing sophisticated algorithms can detect and correct anomalies in precipitation data, ensuring consistency.
- Field validation studies: Conducting on-site verification under various weather conditions helps validate the accuracy of remote sensing data.
Our approach to measurement validation is data-driven, combining multiple methods to guarantee thorough storm tracking and precise precipitation analysis. By focusing on these techniques, we can maintain the integrity of our meteorological data, providing accurate information that empowers users to make informed decisions.
Data Consistency Over Time
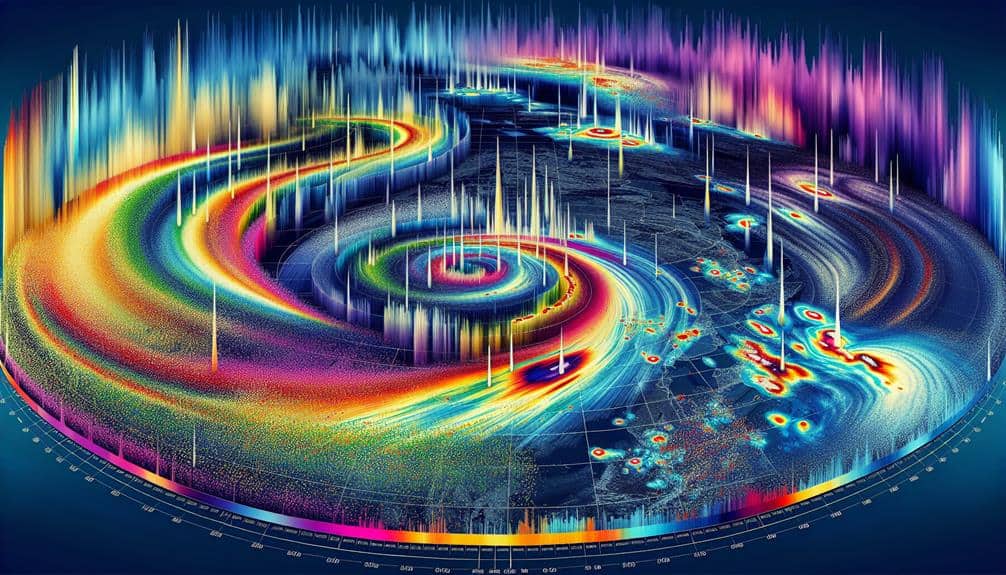
Maintaining data consistency over time is essential for safeguarding the reliability and validity of long-term meteorological studies. We must focus on data integrity and measurement accuracy to achieve this.
Securing data integrity involves cross-referencing multiple data sources and employing automated systems for real-time error detection. This helps us identify discrepancies swiftly and uphold the quality of our data.
Measurement accuracy is another critical aspect. We need to calibrate our instruments regularly and use high-precision equipment to capture meteorological variables like temperature, humidity, and wind speed. When instruments drift out of calibration, it can result in data that's misleading, impacting our storm tracking efforts. By routinely auditing our equipment and updating our calibration protocols, we can mitigate these risks.
Additionally, data normalization techniques allow us to account for seasonal variations and other temporal changes. This safeguards that our long-term datasets remain consistent, even when environmental conditions vary. We also employ statistical methods to detect and correct anomalies, safeguarding that our data remains robust over long periods.
Frequently Asked Questions
How Can Machine Learning Enhance Meteorological Data Validation for Storm Tracking?
We can leverage machine learning applications to enhance data accuracy improvements in storm tracking. By analyzing vast datasets, ML algorithms identify patterns, correct errors, and provide precise predictions, empowering us to make informed, timely decisions.
What Role Does Historical Data Play in Validating Current Storm Tracking Methods?
We leverage historical data to validate current storm tracking methods, ensuring data accuracy and identifying trends. This process provides insights that drive forecast improvements, helping us enhance our predictive models and deliver reliable, timely warnings.
Are There Specific Software Tools Recommended for Real-Time Data Validation?
When addressing software recommendations for real-time validation techniques, we'd suggest tools like AWIPS, WRF, and Python-based libraries. They offer precise, data-driven capabilities, empowering us to make informed decisions without compromising our analytical freedom.
How Do Human Observations Complement Automated Data Validation Systems?
In examining human vs automated observations, we see that human insights add context and real-time adaptability to data validation techniques. They catch anomalies and refine automated processes, ensuring more accurate and reliable meteorological data.
What Are the Ethical Considerations in Using Meteorological Data for Public Safety?
We acknowledge concerns about data privacy, but ensuring public safety is paramount. By maintaining transparency and safeguarding personal information, we can build public trust, effectively using meteorological data to protect communities while respecting individual freedoms.