We rely on several advanced models for predicting storm formations, each leveraging different techniques to enhance accuracy. The Global Forecast System (GFS) and the European Centre Model (ECMWF) integrate high-resolution data and ensemble forecasting for reliable medium-range predictions. The Weather Research and Forecasting (WRF) model excels in capturing fine atmospheric details through detailed simulations. For real-time, hourly updates, the High-Resolution Rapid Refresh (HRRR) model is highly effective. Machine learning algorithms further boost prediction accuracy by identifying complex patterns and updating simulations dynamically. Each of these models plays a critical role in refining our storm forecasting capabilities. Let's explore how they complement each other.
Key Points
- The Global Forecast System (GFS) excels with high-resolution and ensemble forecasting for detailed storm predictions.
- The Weather Research and Forecasting (WRF) Model offers high-resolution simulations capturing fine-scale atmospheric details.
- The High-Resolution Rapid Refresh (HRRR) Model provides hourly updated, high-fidelity forecasts with a 3 km spatial resolution.
- The European Centre Model (ECMWF) delivers accurate medium-range forecasts and outstanding storm formation predictions.
Numerical Weather Prediction
Numerical Weather Prediction (NWP) utilizes mathematical models based on physical laws to forecast atmospheric conditions with a high degree of accuracy. As we track storms, the precision of these models becomes essential. Advanced modeling techniques, such as data assimilation and ensemble forecasting, have greatly enhanced storm tracking accuracy. These methods integrate vast amounts of observational data into models, refining predictions and reducing uncertainties.
Climate change has introduced new variables into storm predictions. Rising sea temperatures and shifting weather patterns complicate our models, but emerging technologies in weather forecasting are helping us adapt. Machine learning algorithms and high-resolution satellite data are now being incorporated into NWP, enhancing our ability to predict storms in a changing climate.
Moreover, the continuous development of computational power allows us to run more complex simulations. These advancements enable us to identify and respond to potential threats more swiftly, giving communities more freedom to prepare and mitigate risks.
Global Forecast System
Let's focus on how the Global Forecast System (GFS) enhances storm prediction through advanced data assimilation techniques, high-resolution models, and ensemble forecasting.
By integrating vast amounts of observational data, GFS enhances forecast accuracy.
Additionally, the system's high model resolution and ensemble approach provide more detailed and probabilistic predictions.
Data Assimilation Techniques
Incorporating data assimilation techniques within the Global Forecast System (GFS) allows us to enhance the accuracy of storm formation predictions in a significant manner. Data assimilation challenges, such as integrating disparate observational data sources and ensuring timely updates, are notable hurdles. Yet, overcoming these obstacles can greatly improve predictive accuracy.
The impact of observational data on model initialization can't be overstated. High-quality, real-time data from satellites, weather stations, and buoys feed into the GFS, giving it a strong starting point. This initialization step is vital for capturing the current state of the atmosphere accurately, which in turn, refines subsequent forecasts. By assimilating such diverse data, we guarantee the model has the most precise representation of atmospheric conditions.
As we process this observational data, we employ sophisticated algorithms to minimize errors and discrepancies. These algorithms, designed to balance data quality and computational efficiency, are crucial for making real-time predictions feasible. Consequently, our ability to accurately predict storm formations hinges on our mastery of these data assimilation techniques. By continuously refining these methods, we can push the boundaries of what's achievable in meteorological forecasting, offering more precise and timely storm warnings.
Model Resolution Impact
When we analyze the impact of model resolution within the Global Forecast System (GFS), we observe significant variations in the accuracy of storm formation predictions. High-resolution models, with finer grid spacing, offer greater detail and capture smaller-scale meteorological phenomena more precisely. This increased model accuracy is critical for predicting the initiation and development of storms. However, there's a resolution trade-off: higher resolution demands more computational power and longer processing times.
The impact of grid spacing on predictions becomes evident when comparing different GFS configurations. For instance, a grid spacing of 13 kilometers can identify mesoscale features that might be entirely missed with coarser resolutions like 25 kilometers. These finer grids enhance the model's ability to forecast localized severe weather events, providing more accurate and timely warnings.
On the flip side, the resolution trade-off can't be ignored. Higher resolution models require substantial computational resources, which can limit their operational feasibility, especially for long-range forecasts. Balancing this trade-off is key. By optimizing grid spacing, the GFS aims to improve storm prediction accuracy while managing computational constraints.
Ultimately, our goal is to achieve the highest possible model accuracy without compromising efficiency and timeliness.
Ensemble Forecasting Benefits
Ensemble forecasting in the Global Forecast System allows us to quantify uncertainty and improve the reliability of storm predictions by running multiple model simulations with slight variations in initial conditions. This approach enhances ensemble forecasting accuracy by providing a range of possible outcomes rather than a single deterministic forecast. When we assess these varying scenarios, we can better understand forecast uncertainty and make more informed decisions.
Probabilistic predictions derived from ensemble models offer a clearer picture of potential storm paths and intensities. By analyzing the spread and consensus among the different simulations, we can identify the most likely scenarios while also accounting for less likely, but still feasible, outcomes. This gives us a more thorough view of potential storm developments and allows us to prepare more effectively.
Ensemble forecasting reliability is vital for both short-term and long-term planning. For instance, emergency management teams can use probabilistic predictions to allocate resources more efficiently, while policymakers can develop strategies that address a range of possible future conditions. Ultimately, integrating ensemble forecasting into our predictive toolkit not only enhances our ability to anticipate storms but also empowers us to navigate the inherent uncertainties with greater confidence and precision.
Weather Research and Forecasting
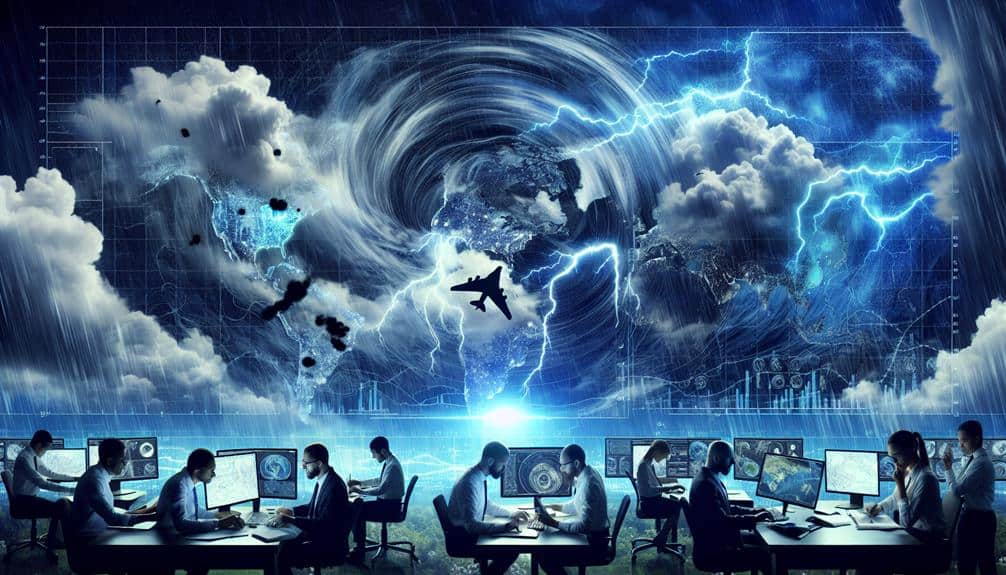
Let's analyze the Weather Research and Forecasting model's strengths.
It excels with high-resolution simulations, capturing fine-scale atmospheric details essential for storm prediction.
Additionally, its real-time data integration enhances accuracy, providing timely and reliable forecasts.
High-Resolution Simulations
High-detail simulations, like those generated by the Weather Research and Forecasting (WRF) model, are vital for accurately predicting storm formations due to their capacity to capture fine-scale atmospheric processes. By improving storm tracking capabilities, we can pinpoint the development and trajectory of storms with greater accuracy.
The WRF model excels at simulating atmospheric dynamics, covering intricate elements like wind patterns, temperature variations, and moisture distribution. These factors are essential in understanding and predicting storm genesis and evolution.
High-detail models allow us to explore deeper into the microphysical processes that govern storm behavior. For example, the WRF can simulate convection and precipitation at a detailed level, offering insights that coarser models might overlook. This level of detail proves essential when predicting localized phenomena, such as tornadoes or flash floods, which can have significant impacts on communities.
Additionally, the data-driven approach of high-detail simulations supports a more thorough analysis of storm systems. By integrating diverse datasets and running numerous simulations, we can evaluate different scenarios and improve our predictive accuracy. This method enhances our ability to make informed decisions, ultimately granting us more flexibility to prepare for and respond to severe weather events.
Real-Time Data Integration
Integrating real-time data into the Weather Research and Forecasting (WRF) model greatly enhances the accuracy of storm predictions by continuously updating simulations with the latest atmospheric observations. This integration allows for more precise predictive analytics, leveraging data visualization techniques to provide an immediate understanding of evolving storm patterns.
By incorporating real-time monitoring, we can respond dynamically to changes, ensuring our predictions remain relevant and accurate.
Machine learning applications play a vital role in this process. Algorithms can sift through vast amounts of incoming data, identifying patterns and anomalies that might signal storm formations. These insights are then fed back into the WRF model, refining its predictive capabilities.
The synergy between real-time data and machine learning enables us to adapt quickly, reducing the lag between observation and prediction.
Furthermore, the use of advanced data visualization techniques makes complex data sets more accessible and actionable. Visual tools allow meteorologists to interpret real-time data efficiently, making informed decisions quicker.
This level of responsiveness is essential for communities seeking autonomy in preparing for and mitigating the impacts of storms. By harnessing real-time data integration, the WRF model becomes an indispensable tool for proactive storm prediction and management.
High-Resolution Rapid Refresh
The High-Resolution Rapid Refresh (HRRR) model provides hourly updated, high-fidelity forecasts that are instrumental in predicting storm formations with greater accuracy. It stands out due to its ability to assimilate high-frequency data from various sources, enhancing storm tracking capabilities and offering a more precise depiction of weather phenomena.
Using advanced weather modeling techniques, the HRRR effectively captures small-scale atmospheric processes, which are essential for accurate storm prediction. By leveraging data from Doppler radars, satellites, and surface observations, the HRRR generates detailed forecasts up to 18 hours ahead. This granularity is necessary for identifying rapid changes in storm intensity and movement, thereby aiding in timely decision-making.
The model operates at a high spatial resolution of 3 kilometers, allowing us to discern localized weather patterns that broader models might miss. This precision is particularly valuable in regions prone to severe weather events, where early warnings can mitigate damage and save lives.
Additionally, the HRRR's continuous hourly updates assure that we've the most current information, reducing the lag between observation and prediction.
European Centre Model
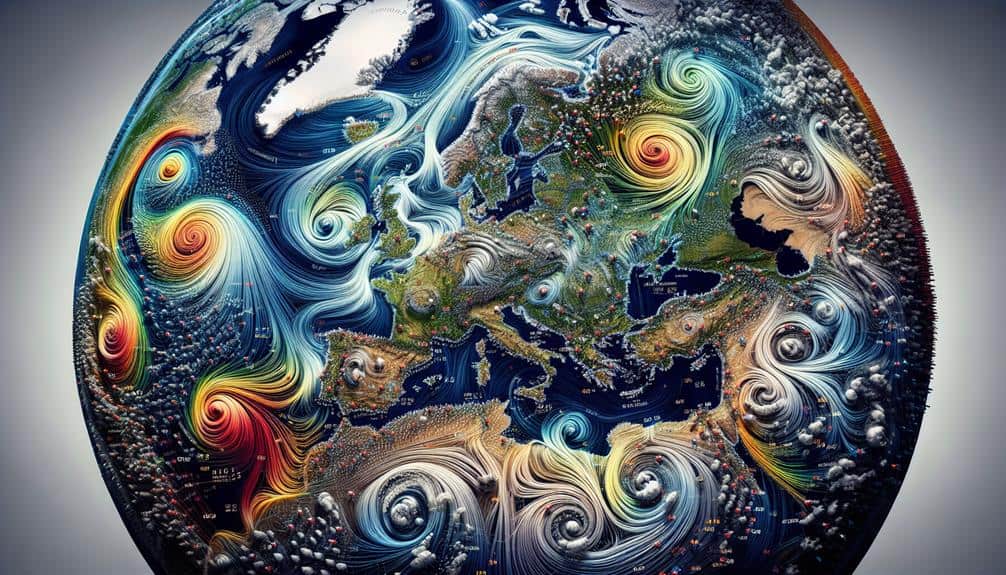
Leveraging sophisticated numerical weather prediction techniques, the European Centre Model (ECMWF) provides some of the most accurate medium-range weather forecasts available.
By focusing on model comparison and accuracy assessment, we can see that ECMWF consistently outperforms other models in predicting storm formations. This is largely due to its advanced parameter tuning and sensitivity analysis, which optimize the model's performance.
We should highlight the ECMWF's strengths through a few key points:
- Global Data Assimilation: ECMWF ingests a massive amount of global data, ensuring that the initial conditions for its forecasts are as precise as possible.
- High Spatial Resolution: The model operates at a high spatial resolution, allowing it to capture fine-scale atmospheric processes that are essential for storm prediction.
Machine Learning Approaches
In recent years, we've observed machine learning algorithms significantly improve the accuracy of storm prediction models by leveraging extensive datasets for pattern recognition and predictive analytics. By utilizing deep learning applications, we can now identify intricate patterns within meteorological data that were previously unnoticeable. These advanced techniques have transformed storm tracking, giving us more precise and timely forecasts.
A crucial aspect of these machine learning models is feature selection. By carefully selecting relevant variables, such as atmospheric pressure, sea surface temperature, and humidity levels, we ensure that our weather prediction models are both robust and efficient. This focused approach reduces noise and enhances the model's ability to predict storm formations accurately.
Furthermore, deep learning applications utilize neural networks to process large amounts of data, allowing for real-time storm tracking and prediction updates. These models continually learn and adapt, improving their predictive capabilities over time. The combination of sophisticated algorithms and extensive datasets has resulted in significant advancements in our ability to predict storms, ultimately assisting in disaster preparedness and risk management.
In essence, machine learning approaches empower us with the tools to make more informed decisions, providing a crucial advantage in weather prediction and storm tracking.
Frequently Asked Questions
How Do Storm Prediction Models Account for Climate Change?
We analyze how storm prediction models integrate the impact of greenhouse gases and weather pattern changes. By updating algorithms with real-time data, we guarantee accurate reflections of our evolving climate, enabling better preparedness and freedom from unexpected events.
What Role Do Satellites Play in Storm Prediction?
Satellites are our eyes in the sky, enhancing storm prediction by providing precise, real-time data. Satellite technology boosts data accuracy, helping us make informed decisions and feel more secure in our ability to anticipate severe weather events.
How Can Local Weather Stations Improve Storm Forecasting Accuracy?
We can improve storm forecasting accuracy by enhancing data collection and leveraging technology advancements. By integrating real-time data from local weather stations, we'll achieve more precise predictions, empowering communities with timely, reliable information to make informed decisions.
What Are the Limitations of Current Storm Prediction Models?
Current models have limitations like data gaps, resolution constraints, and computational limits. We can pursue improvements by enhancing data quality, integrating AI, and increasing computational power to deliver more precise, timely storm predictions, supporting individual freedom and safety.
How Does Storm Prediction Impact Emergency Preparedness and Response?
Isn't it ironic that storm prediction, often ignored, drastically enhances emergency response and community preparedness? Accurate forecasts save lives, reducing chaos and enabling timely evacuations. Data-driven models inform strategies, ensuring we're always ready for nature's surprises.