We employ historical storm data to analyze recurring behaviors and anomalies, essential for accurate storm path forecasting. By integrating data from satellites, weather stations, ocean buoys, and reconnaissance aircraft, we construct robust models. These models use predictive algorithms, linear regression, and neural networks to simulate potential storm trajectories. Accuracy comes from rigorous evaluation methods like MAE and RMSE, ensuring our forecasts adapt to changing environmental factors. Combining high-resolution historical data with precise surveying techniques helps us refine our predictive frameworks. If you're looking to enhance storm prediction capabilities through these methods, advanced insights await.
Key Points
- Historical data constructs robust forecasting models for accurate storm path predictions.
- Satellites, weather stations, and ocean buoys provide critical real-time data for model accuracy.
- Feature selection and algorithms like linear regression improve predictive model performance.
- Comparing predicted storm paths with historical outcomes enhances forecasting reliability.
Understanding Storm Patterns
To accurately forecast storm paths, we must first analyze historical storm patterns to identify recurring behaviors and anomalies. By examining past data, we can discern how storm movement typically unfolds and recognize any deviations caused by broader environmental factors. This analysis is essential for predicting future events with greater accuracy.
When we track storm patterns, we notice that certain behaviors are often repeated. For example, storms might tend to follow similar paths based on geographic and atmospheric conditions. However, climate change has introduced additional variables, altering these patterns and increasing storm frequency. As a result, what was once predictable has become more intricate.
By studying historical storm data, we gain insights into the mechanics of storm movement. We can map out typical trajectories and understand how factors like ocean temperatures and wind currents influence their paths. This helps us build more dependable forecasting models.
Furthermore, recognizing the interplay between climate change and storm frequency is vital. The rising global temperatures lead to more frequent and intense storms. As a result, our tracking patterns must account for these changes to provide accurate forecasts. By refining our methods, we can better prepare and respond to future storm events, ensuring greater safety and freedom for all.
Importance of Historical Data
Historical data serves as the cornerstone for constructing robust and accurate storm forecasting models. By analyzing past storm paths, we can identify patterns and trends that help predict future behavior. Data accuracy is paramount in this process; any errors can lead to significant deviations in our models. Consequently, we must make sure that our historical data is meticulously verified and validated.
Trend analysis allows us to discern recurring behaviors in storm movements. For instance, we can examine how specific atmospheric conditions, ocean temperatures, and wind patterns have influenced storm trajectories over the years. These insights are invaluable, enabling us to develop predictive algorithms that can anticipate storm paths with a higher degree of confidence.
Moreover, historical data provides the statistical foundation needed to simulate various storm scenarios. By inputting different variables, we can test how storms might react under varying conditions. This not only helps in improving our forecasting models but also in understanding the inherent uncertainties in storm predictions.
Data Collection Methods
Accurate storm forecasting hinges on the meticulous collection of diverse data sets from satellites, weather stations, ocean buoys, and reconnaissance aircraft. We can't emphasize enough the importance of employing advanced surveying techniques to gather this data efficiently.
Satellites, for instance, provide us with real-time satellite imagery that captures the storm's evolution from space, offering invaluable insights into its formation and trajectory.
Weather stations on the ground play a pivotal role by recording atmospheric conditions such as temperature, humidity, and wind speeds. These stations are strategically placed to ensure thorough geographic coverage, maximizing the accuracy of our forecasts.
Ocean buoys, equipped with sensors, relay critical data about sea surface temperatures and wave heights, which are essential for understanding storm surges and potential flooding.
Reconnaissance aircraft, often referred to as 'hurricane hunters,' fly directly into the storm system to measure wind speeds, pressure, and other atmospheric parameters. This direct approach provides high-resolution data that supplements the broader-scale information gathered from satellites and ground stations.
Combining these diverse data sources through precise surveying techniques enables us to create a comprehensive model of storm behavior. The key lies in the seamless integration of data, allowing us to predict storm paths more accurately and offer timely warnings to those in harm's way.
Analyzing Past Storms
By meticulously analyzing past storm patterns and behaviors, we can refine our predictive models and improve future forecasting accuracy. To achieve this, we must examine historical data on storm intensity, tracking how it fluctuated over time and its correlation with specific atmospheric conditions. This analysis allows us to identify recurring trends and anomalies that can inform our understanding of storm dynamics.
We must also consider the geographical impact of past storms. By mapping the paths of previous storms and overlaying them with data on topography, population density, and infrastructure, we can discern patterns in how different regions are affected. This step is pivotal for pinpointing areas that are particularly vulnerable and for developing targeted mitigation strategies.
Comparing storms of varying intensities and their respective impacts on different geographical areas enables us to create a more nuanced predictive framework. For instance, a storm with high intensity may cause devastating effects in coastal regions but may weaken significantly upon moving inland. By analyzing these distinctions, we can tailor our forecasting models to account for both intensity and geographical factors.
Ultimately, this rigorous analysis empowers us to make more informed predictions, enhancing our ability to safeguard communities and optimize resource allocation.
Predictive Modeling Techniques
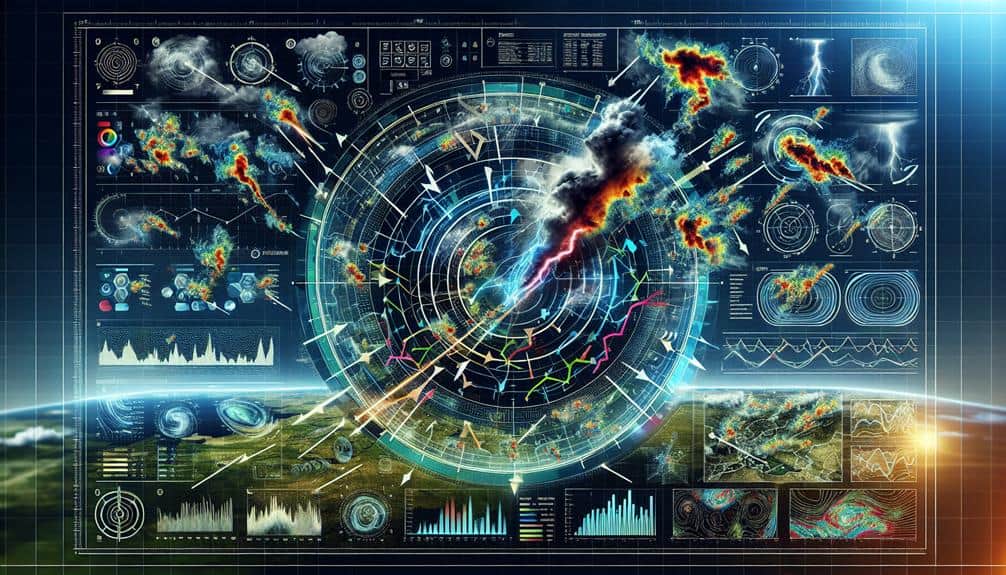
Building on our analysis of past storms, we can now explore predictive modeling techniques that leverage this historical data to forecast future storm paths with greater precision. By employing various models, we can identify patterns and relationships within the data that weren't immediately apparent.
The first step in this process is feature selection, where we determine which variables—such as wind speed, pressure, and humidity—are most predictive of a storm's trajectory.
Once we've selected our features, we can use algorithms like linear regression, decision trees, and neural networks to build our predictive models. Each technique has its strengths and trade-offs, but our goal is to maximize accuracy while maintaining computational efficiency.
After constructing our models, we must rigorously evaluate their performance. Model evaluation involves metrics such as mean absolute error (MAE), root mean squared error (RMSE), and R-squared values. These metrics help us understand how well our model predicts storm paths and where it might fall short.
Tools for Storm Forecasting
To enhance our storm path forecasts, we rely on advanced meteorological software and extensive historical weather databases. These tools enable us to analyze past storm data and generate predictive models with high accuracy.
Advanced Meteorological Software
Meteorologists depend on advanced software like the Weather Research and Forecasting (WRF) model to predict storm paths with high precision. These sophisticated meteorological tools allow us to simulate atmospheric conditions in high detail, greatly improving our storm tracking and weather prediction capabilities.
The WRF model, for example, integrates various data inputs such as temperature, humidity, wind speed, and pressure to produce accurate forecasts.
To significantly enhance accuracy, we use both deterministic and probabilistic approaches. Deterministic models provide a single, specific forecast, while probabilistic models offer a range of potential outcomes, helping us evaluate the variability in storm paths.
Tools such as the Global Forecast System (GFS) and the European Centre for Medium-Range Weather Forecasts (ECMWF) are also crucial. They employ complex algorithms that consider the Earth's atmospheric dynamics, providing dependable predictions across various time spans.
Historical Weather Databases
Our ability to predict storm paths greatly improves through the use of historical weather databases, which provide a wealth of past atmospheric data for analysis. These databases compile extensive records of meteorological parameters such as temperature, humidity, wind speed, and atmospheric pressure. By leveraging this historical data, we can identify patterns and trends that are essential for accurate storm tracking.
When we analyze past weather events, we gain insights into how storms have historically developed and moved. This allows us to recognize recurring weather trends, which serve as valuable predictors for future storm behavior. For instance, historical data can reveal the typical paths that storms tend to follow in certain regions during specific times of the year. This knowledge empowers us to make more informed predictions about where a current storm might head next.
Moreover, historical weather databases are instrumental in refining our forecasting models. By comparing predicted storm paths with actual historical outcomes, we can continually improve the accuracy of our models. This iterative process ensures that our storm tracking methods evolve and adapt, providing greater assurance and enhancing our preparedness for adverse weather conditions.
Through precise analysis of historical data, we guarantee the potential to forecast storms with greater accuracy and confidence.
Improving Forecast Accuracy
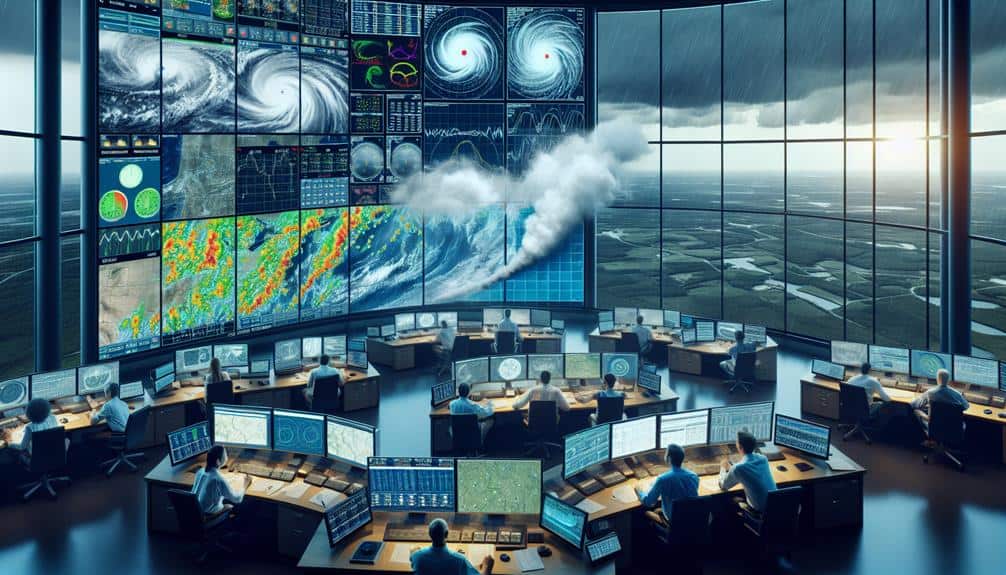
Enhancing forecast accuracy hinges on leveraging high-resolution historical data to refine predictive models. By meticulously analyzing past storm tracking information, we can identify patterns and anomalies that improve our predictions.
Our process involves three important steps that guarantee our models are both robust and dependable.
- Data Integration: We incorporate diverse datasets, such as satellite imagery, radar data, and historical storm paths, to create a thorough foundation. This integration allows us to capture the complexities of storm behavior.
- Model Calibration: Using historical data, we calibrate our predictive models to enhance their accuracy. By adjusting parameters based on past storm tracking and behavior, we fine-tune the models to better reflect real-world conditions.
- Forecast Validation: Continuous validation of our forecasts against actual outcomes is essential. We compare predicted storm paths with historical data to assess accuracy and make necessary adjustments. This iterative process ensures our models remain precise and dependable.
Frequently Asked Questions
How Do Storm Paths Impact Local Wildlife and Ecosystems?
When it rains, it pours. Storm paths cause habitat destruction, leading to species displacement and significant biodiversity impacts. However, ecosystem resilience can help recovery, although some local wildlife may never fully return to pre-storm conditions.
What Are the Ethical Considerations in Storm Forecasting?
We must consider data privacy and community impact in storm forecasting. Ensuring individuals' information remains secure while providing accurate predictions can save lives and minimize disruption, empowering communities to take proactive measures and maintain their autonomy.
How Are Storm Forecasts Communicated to the Public?
We softly sound the alarm through emergency alerts and extensive media coverage. This guarantees everyone's informed and prepared. We use precise, technical language to give clear guidance, respecting your freedom to act on accurate, timely information.
What Careers Are Available in Storm Forecasting?
In storm forecasting, we can pursue meteorology careers, including research opportunities in atmospheric sciences, operational meteorology, and climate modeling. These roles enable us to analyze weather patterns, develop predictive models, and disseminate crucial information to the public.
How Can Communities Prepare for Predicted Storm Paths?
To prepare for predicted storm paths, we should strengthen our emergency response systems and develop efficient evacuation plans. Ensuring timely information and resources allows communities to act swiftly, minimizing risks and preserving our freedom during crises.