We leverage advanced statistical models to analyze storm data because they allow precise predictions and effective risk management. By utilizing historical weather data and real-time atmospheric conditions, these models help us predict storm patterns and assess risks accurately. Machine learning algorithms process vast datasets efficiently, enhancing our forecasts dynamically. Rigorous data validation guarantees accuracy, while simulations of multiple storm scenarios provide insights into potential impacts. This approach helps us minimize storm damage, safeguard communities, and allocate resources effectively. Continue to explore with us to uncover the intricacies of storm data analysis and how it transforms into actionable insights.
Key Points
- Statistical models enable accurate predictions by analyzing vast historical and real-time atmospheric data.
- They enhance data accuracy through robust validation techniques and precise instrument calibration.
- Models help identify regions most susceptible to storm damage by evaluating risk factors and historical data.
- Machine learning algorithms within statistical models process data efficiently, improving forecast precision.
Predicting Storm Patterns
Utilizing advanced statistical models, we can accurately predict storm patterns by analyzing historical weather data and real-time atmospheric conditions. By leveraging machine learning algorithms, we process vast datasets to identify trends and anomalies that traditional methods might miss. These algorithms, trained on extensive historical data, can adapt to new information, enhancing our predictive capabilities.
Data visualization plays a pivotal role in this process. By transforming complex datasets into intuitive visual formats, we can quickly interpret the results of our models. This visual clarity allows us to make informed decisions and communicate potential storm impacts more effectively. For instance, heat maps and trajectory plots provide immediate insights into storm paths and intensities, empowering communities to prepare proactively.
Our approach combines the computational power of machine learning with the clarity of data visualization, ensuring our predictions are both accurate and actionable. We prioritize transparency and accessibility in our data presentation, fostering a sense of autonomy and preparedness. This methodology not only enhances our understanding of storm behavior but also supports communities in making informed, timely decisions that safeguard lives and property.
Enhancing Data Accuracy
To enhance data accuracy, we focus on refining data collection methods and employing robust validation techniques. By improving the precision of our instruments and methodologies, we markedly reduce errors in our storm data. Accurate data is paramount because even minor discrepancies can lead to flawed analyses and misguided decisions.
We rigorously apply data validation processes to ensure the integrity of our datasets. Data validation includes cross-referencing multiple data sources, employing statistical checks, and using machine learning algorithms to identify anomalies. This multi-faceted approach helps in pinpointing and rectifying inconsistencies, leading to more reliable datasets.
Error reduction is another critical aspect of enhancing data accuracy. We continually calibrate our sensors and update our software to minimize systematic errors. Additionally, implementing redundancy in data collection allows us to cross-verify data points, which notably lowers the chances of unnoticed errors.
Risk Assessment Strategies
Effective risk assessment strategies are crucial for accurately predicting the potential impact of storms and formulating appropriate response measures. By employing robust statistical models, we can evaluate various risk factors and enhance our emergency response capabilities. These models allow us to quantify the probability of different storm events and their potential consequences on infrastructure and populations.
Our vulnerability assessment involves analyzing historical storm data, socioeconomic factors, and geographical information. By doing so, we can identify the regions most susceptible to storm damage and allocate resources more efficiently. For instance, we can pinpoint areas where critical infrastructure, like hospitals and power plants, are at higher risk, enabling us to fortify these locations proactively.
Moreover, statistical models help us simulate multiple storm scenarios, providing insights into potential outcomes. This enables emergency response teams to develop targeted strategies and contingency plans tailored to specific risks. By integrating data on wind speeds, precipitation levels, and storm surges, we can create essential risk profiles that guide decision-making processes.
Ultimately, these risk assessment strategies empower us to minimize storm impact, safeguard communities, and optimize resource allocation. Through data-driven analyses, we can achieve a higher degree of preparedness and resilience, ensuring that we maintain our freedom and safety even in the face of severe weather events.
Real-Time Data Processing
Real-time data processing allows us to swiftly analyze incoming storm data, enabling timely and informed decision-making during severe weather events. By utilizing advanced data visualization techniques, we can present complex datasets in an intuitive manner, aiding in rapid comprehension and response. This is essential when we're faced with volatile and fast-changing storm conditions, where every second counts.
Incorporating machine learning algorithms into our analysis pipeline enhances our ability to detect patterns and anomalies in the data. These algorithms help with trend analysis by continuously learning from new data inputs, thereby improving the accuracy of our predictions over time. Predictive analytics, supported by these machine learning models, allows us to forecast storm trajectories and intensities with increasing precision, providing crucial lead time for emergency responses.
Furthermore, real-time data processing empowers us to update our models dynamically as new information becomes available. This adaptability guarantees that our models remain relevant and effective under evolving circumstances, granting us the freedom to make data-driven decisions swiftly and confidently.
Ultimately, the integration of real-time data processing with statistical models is a game-changer in storm data analysis, enhancing our readiness and resilience against severe weather phenomena.
Improving Forecast Models
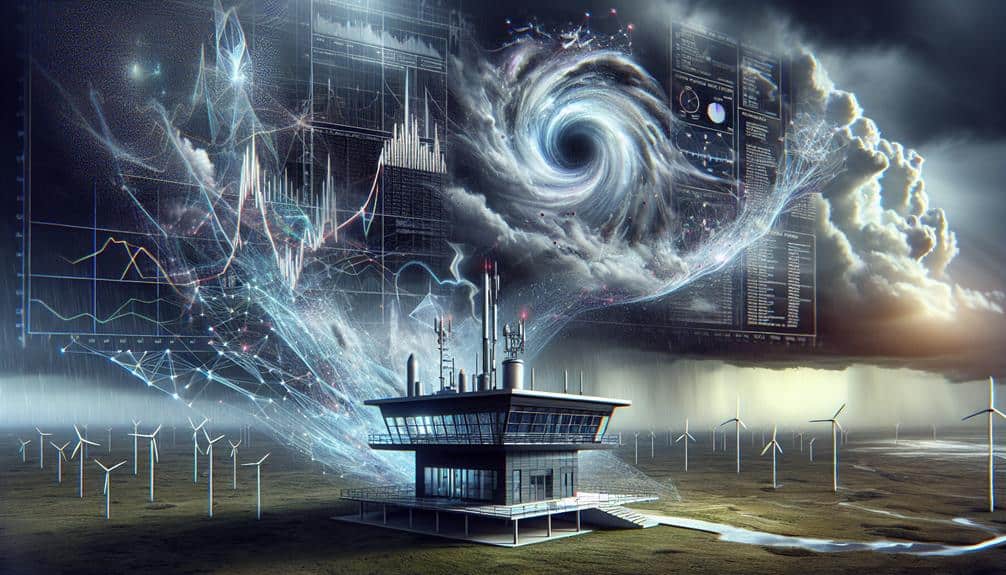
Enhancing our forecast models depends on integrating high-resolution data and advanced computational techniques to achieve greater predictive precision. By leveraging high-resolution satellite imagery and radar data, we can capture the complexities of storm development, enabling more accurate predictions.
Advanced computational techniques, such as machine learning algorithms, allow us to process this vast amount of data efficiently, identifying patterns that traditional methods might overlook.
Model validation is essential in this process. We need to rigorously test our models against historical storm data to make sure they accurately predict future events. This includes cross-referencing forecasts with actual outcomes and refining algorithms based on discrepancies. A validated model increases our confidence in its predictive power, allowing for more informed decision-making.
Data visualization plays a pivotal role in improving forecast models. By transforming raw data into visual formats, we can quickly identify trends and anomalies. Effective visualizations, such as heat maps and predictive trajectory plots, make complex data understandable at a glance, facilitating rapid analysis and response.
Frequently Asked Questions
What Types of Data Are Used in Storm Analysis?
We use various data sources and data types in storm analysis, including satellite imagery, radar data, and historical storm records. Data collection involves real-time monitoring, and data analysis helps us understand patterns and predict future storm behavior.
How Do Statistical Models Handle Missing or Incomplete Storm Data?
How do we handle missing storm data? Statistical models use data imputation to estimate missing values, guaranteeing thorough analysis. By validating models, we ensure accuracy and reliability, empowering us to make informed decisions despite incomplete datasets.
What Role Do Historical Storm Data Play in Model Development?
We leverage historical storm data for data visualization and trend analysis, enhancing machine learning algorithms. This improves forecasting accuracy, empowering us to make informed decisions and maintain our freedom to proactively manage storm-related challenges.
Can Statistical Models Predict Economic Impacts of Storms?
Like a lighthouse guiding ships, statistical models help us navigate economic forecasting and risk assessment. They analyze storm data to predict economic impacts, providing valuable insights for preparing and mitigating financial risks associated with severe weather events.
How Do Statistical Models Integrate With Other Meteorological Tools?
By integrating statistical models with other meteorological tools, we enhance data integration and improve forecast accuracy. This synergy allows us to provide precise, actionable insights, empowering communities to make informed decisions and maintain their autonomy during storm events.