We decipher meteorological data trends in storm events by utilizing historical data and analyzing key indicators like atmospheric pressure, wind speed, and humidity. We're observing that climate change affects storm frequency and intensity, with warmer ocean temperatures fueling more severe weather. Remote sensing and machine learning enhance our data collection, while advanced algorithms and visualization tools highlight important trends and anomalies. Predictive analytics and refined weather models, integrating real-time and historical data, propel our accurate storm forecasts. These methodologies guarantee more reliable predictions and adaptive strategies. For more insights on enhancing community resilience and disaster response, keep exploring with us.
Key Points
- Patterns and anomalies in storm frequency and intensity reveal climate change impacts.
- Rapid drops in atmospheric pressure indicate storm development.
- High humidity and warmer ocean temperatures contribute to severe weather.
- Machine learning enhances the accuracy of storm data analysis and forecasting.
Analyzing Storm Patterns
By examining historical data, we can identify recurring patterns and anomalies in storm events. This analysis enables us to understand the climate change impact on storm frequency and intensity. Utilizing detailed storm tracking, we observe how warmer ocean temperatures and shifting atmospheric conditions contribute to more severe and unpredictable weather events. Our focus on data reveals that these changes necessitate updated disaster response strategies to enhance community resilience.
Emergency preparedness hinges on our ability to anticipate storm behavior accurately. By analyzing past storm patterns, we gain insights into potential future events. Precision in storm tracking allows us to predict paths and intensities with greater accuracy, crucial for timely evacuations and resource allocation. Historical data doesn't just inform us—it empowers us to act.
Enhanced emergency preparedness involves not just reactive measures but proactive strategies. Our analysis of storm data guides the development of infrastructure designed to withstand extreme weather conditions. It informs public policies aimed at reducing vulnerabilities and improving rapid response capabilities. By leveraging this information, we ensure that our strategies evolve in tandem with the shifting climate landscape, safeguarding our freedom to live without constant fear of natural disasters.
Key Meteorological Indicators
To accurately anticipate storm behavior and enhance our preparedness, we must focus on key meteorological indicators such as atmospheric pressure, wind speed, and humidity levels. These indicators provide vital insights into the formation and potential impact of storm events.
For instance, a rapid drop in atmospheric pressure often signifies the development of a storm system. By monitoring these pressure changes, we can predict the intensity and trajectory of approaching storms.
Wind speed is another pivotal indicator. Increased wind speed can indicate the storm's strength and potential for damage. High-resolution wind speed data allows us to gauge the storm's evolution and implement timely safety measures.
Humidity levels play a significant role in storm development. High humidity fosters the formation of storm clouds and precipitation. By analyzing humidity trends, we gain insights into the storm's moisture content and precipitation potential.
Temperature fluctuations are equally important. Sudden changes in temperature can destabilize the atmosphere, leading to severe weather conditions. Tracking these fluctuations helps us understand the storm's dynamics and potential severity.
Data Collection Techniques
Effective data gathering methods are vital for accurately monitoring and analyzing meteorological indicators during storm events. We employ a variety of approaches, with remote sensing being a cornerstone. Satellites equipped with advanced sensors capture real-time data on atmospheric conditions, sea surface temperatures, and cloud formations. This allows us to gather extensive datasets that are essential for understanding storm dynamics.
Once collected, this raw data undergoes thorough data processing. Advanced algorithms filter out noise and correct any anomalies, ensuring the integrity of our datasets. Here, machine learning comes into action. By applying machine learning models, we can automate data processing tasks and identify patterns that might be overlooked by traditional methods. This not only improves accuracy but also speeds up the entire process.
Data visualization is the final step in our technique arsenal. Through sophisticated data visualization tools, we transform complex datasets into intuitive graphical representations. This makes it easier to communicate findings and supports informed decision-making. These visualizations can highlight trends and anomalies in storm behavior, offering valuable insights for both scientists and policymakers.
Interpreting Weather Models
Understanding weather models necessitates a thorough analysis of multiple variables to accurately predict storm events. By examining atmospheric pressure, temperature, humidity, and wind patterns, we can identify potential storm developments and trajectories. These models, constructed on historical data and current observations, offer a structured approach to weather forecasting, providing significant insights into storm behaviors.
To effectively interpret these models, we must consider:
- Data Integration: Combining diverse data sources (satellite imagery, ground sensors) enhances model accuracy.
- Temporal Resolution: High-frequency data captures rapid changes, essential for short-term forecasts.
- Spatial Resolution: Detailed geographic data improves local forecast precision, essential for storm tracking.
- Model Validation: Comparing model outputs with actual events ensures reliability and fine-tunes forecasting algorithms.
As we explore these models, it's crucial to recognize the influence of climate change on storm patterns. Rising global temperatures and shifting climate norms alter atmospheric dynamics, leading to more intense and unpredictable storm activity. By integrating climate change variables into our models, we can adapt our forecasting techniques to better predict these evolving threats.
In our pursuit of accurate weather forecasting, we embrace the freedom that comes with knowledge, empowering us to make informed decisions and safeguard communities against the increasing impact of storms.
Predictive Analytics in Storms
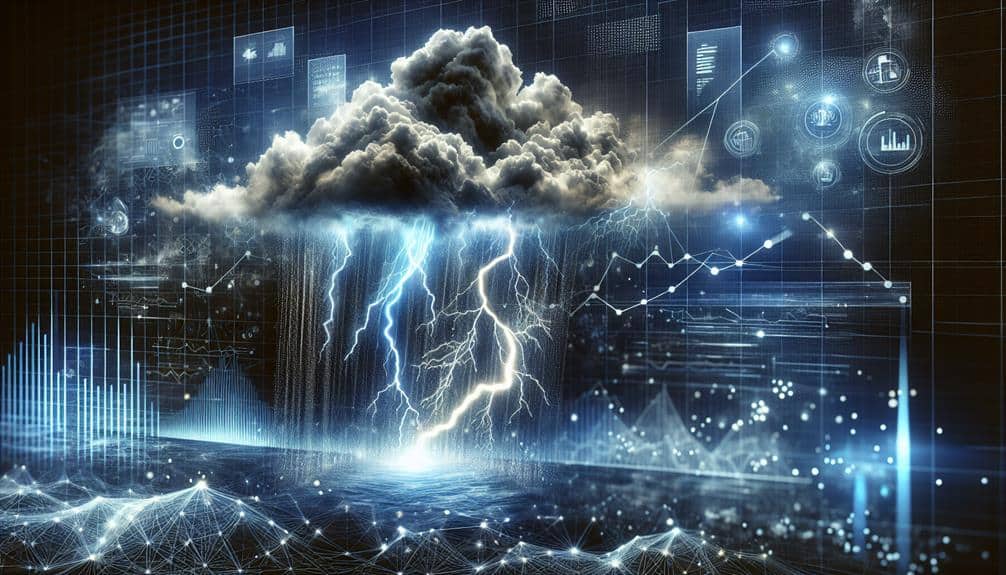
Leveraging predictive analytics, we can enhance our ability to forecast storm events by analyzing vast datasets and identifying patterns that traditional models might miss. By harnessing the power of machine learning, we can process immense amounts of meteorological data to detect subtle trends and correlations. These advanced techniques enable us to refine our forecasting accuracy, providing more reliable predictions and timely warnings.
Incorporating machine learning algorithms allows us to sift through historical storm data, satellite imagery, and real-time weather observations. This inclusive approach helps us identify precursor signals that often precede severe weather events. The result? Improved predictive models that can anticipate storm paths, intensities, and potential impacts with greater precision.
Moreover, we can continuously update our models as new data becomes available, ensuring our forecasts remain current and relevant. This dynamic capability not only enhances our readiness but also empowers communities to take proactive measures, thereby mitigating potential damage and safeguarding lives.
Frequently Asked Questions
How Do Storm Events Impact Local Wildlife Populations?
Storm events disrupt habitats, impacting local wildlife populations. We see shifts in population dynamics and forced adaptation strategies. Wildlife conservation efforts must address these challenges to mitigate habitat disruption and guarantee species survival amidst changing conditions.
What Are the Economic Consequences of Frequent Storm Events?
Just as the butterfly effect suggests small changes can lead to significant outcomes, frequent storm events lead to severe economic impacts. We see infrastructure damage, rising insurance costs, and extensive recovery efforts straining local economies.
How Can Communities Better Prepare for Severe Storms?
To enhance community readiness for severe storms, we should allocate emergency resources efficiently. By investing in robust infrastructure, early warning systems, and public education, we can mitigate risks and guarantee our communities stay resilient and free.
What Role Do Climate Change and Global Warming Play in Storm Frequency?
We see climate change and global warming increasing storm frequency. The data shows a clear uptrend in storm events, suggesting these factors have a notable impact on our weather patterns. Understanding this helps us advocate for freedom through better preparedness.
What Are the Psychological Effects of Repeated Storm Events on Affected Populations?
When it rains, it pours. Repeated storm events have a profound impact on mental health, leading to trauma. However, communities develop resilience and coping strategies, demonstrating remarkable adaptability. Data shows increased anxiety but also a growth in communal support systems.