We're utilizing data analytics to transform atmospheric research by meticulously analyzing measurements like temperature, humidity, and wind speed from remote sensing technologies and UAVs. Statistical modeling, machine learning algorithms, and time-series analysis allow us to uncover patterns and enhance predictive accuracy, particularly for storm patterns and climate trends. These techniques enable us to adapt models in real-time, improving weather forecasts and proactive decision-making. Tools such as neural networks and support vector machines continuously refine predictions, aiding in precise environmental responses. For those interested, we'll reveal how these advancements enable sophisticated analysis and timely interventions in atmospheric phenomena.
Key Points
- Machine learning algorithms enhance predictive accuracy and computational efficiency for atmospheric research.
- Remote sensing technologies provide comprehensive atmospheric data from space for large-scale analysis.
- Statistical modeling identifies patterns and trends in atmospheric data for climate prediction.
- Real-time data applications improve the responsiveness and accuracy of weather forecasts.
Understanding Atmospheric Data
To effectively analyze atmospheric data, we must first understand the different types and sources of data available. Atmospheric data encompasses a range of measurements, including temperature, humidity, wind speed, and chemical composition. This data is essential for climate modeling and evaluating air quality. By dissecting these datasets, we can predict future climate trends and identify pollution sources, providing us with the freedom to make informed decisions.
Climate modeling relies heavily on accurate atmospheric data. We use historical and real-time data to simulate future climate scenarios. These models help us understand potential changes in weather patterns, sea levels, and global temperatures. The precision of our models depends on the quality and thoroughness of the data we collect.
Air quality data, on the other hand, involves monitoring pollutants like nitrogen dioxide, sulfur dioxide, and particulate matter. This data is vital for public health and regulatory compliance. By analyzing air quality data, we can pinpoint pollution hotspots and track the effectiveness of emission reduction strategies.
Tools for Data Collection
A variety of advanced instruments and technologies enable us to collect accurate and detailed atmospheric data. Remote sensing technologies, such as satellites equipped with advanced sensors, provide essential data on atmospheric conditions from space. These satellites measure variables such as temperature, humidity, and wind speed over vast areas, offering a comprehensive view that ground-based measurements can't achieve.
On the ground, sensor networks form the backbone of localized atmospheric monitoring. These networks consist of numerous interconnected sensors strategically placed to gather high-resolution data. Instruments like anemometers, barometers, and hygrometers continuously measure wind speed, atmospheric pressure, and moisture levels, respectively. By leveraging these sensor networks, we achieve detailed insights into microclimatic conditions, important for localized weather prediction and climate studies.
Unmanned Aerial Vehicles (UAVs) also play an increasingly important role in data collection. Equipped with specialized sensors, UAVs can traverse hard-to-reach areas, capturing real-time atmospheric data at various altitudes. This flexibility allows us to study phenomena such as localized storm systems and pollution dispersion with exceptional precision.
Data Analysis Techniques
Effective data analysis techniques enable us to transform raw atmospheric data into actionable insights, driving advancements in weather prediction and climate research. We rely on statistical modeling to identify patterns and trends within large datasets. By applying regression analysis, time-series analysis, and other statistical methods, we can uncover relationships between various atmospheric variables, like temperature, humidity, and wind speed. These insights are pivotal for developing robust predictive models.
Next, we incorporate machine learning algorithms to enhance the predictive accuracy and computational efficiency of our models. Techniques such as neural networks, decision trees, and support vector machines allow us to process vast amounts of data quickly, identifying complex, non-linear relationships that traditional statistical methods might miss. These algorithms can adapt over time, learning from new data to improve their predictions continually.
Predicting Storm Patterns
Leveraging these advanced techniques, we can significantly enhance our capacity to predict storm patterns with greater accuracy and timeliness. By integrating storm tracking and climate modeling, we create sophisticated systems that analyze vast datasets. Our approach involves using machine learning algorithms to identify patterns within historical weather data. This enables us to predict the trajectory and intensity of storms with exceptional precision.
Weather forecasting benefits greatly from these techniques. We can now simulate various atmospheric conditions and their potential outcomes more effectively. By employing high-resolution data visualization tools, we can present complex climate models in a more easily understandable manner. This not only aids meteorologists in making informed decisions but also empowers individuals and communities to prepare for severe weather events.
Moreover, our predictive models continuously improve as they ingest more data. As a result, storm tracking becomes increasingly reliable, contributing to more precise early warning systems. These advancements in climate modeling and weather forecasting offer an invaluable resource for mitigating the impacts of storms.
Ultimately, our goal is to provide timely, actionable insights that enhance public safety and resilience. By harnessing data analytics, we're transforming how we interpret and predict atmospheric phenomena.
Real-Time Data Application
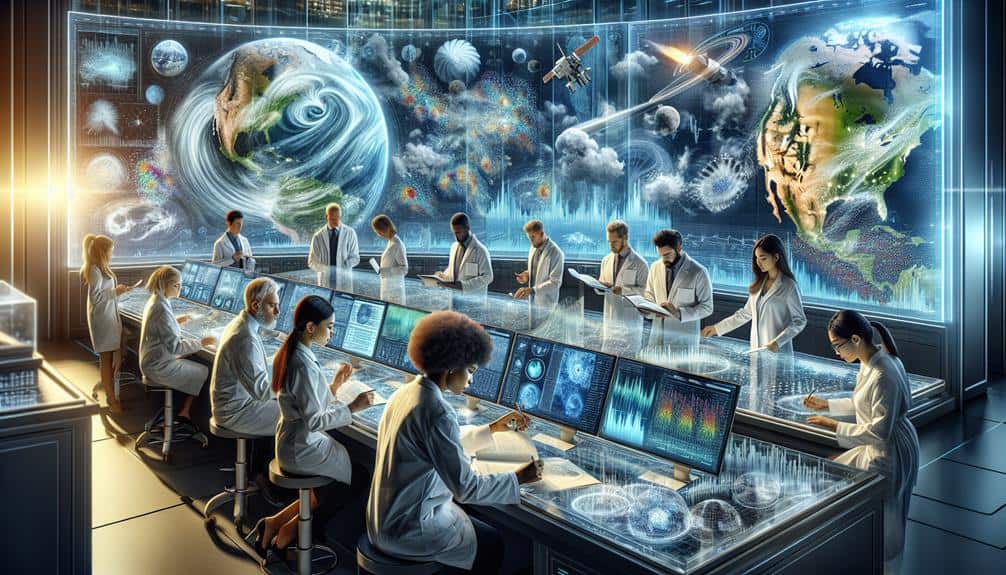
Incorporating real-time data into atmospheric research allows us to make immediate, informed decisions that improve our understanding and response to dynamic weather patterns. By integrating real-time data, we can enhance weather monitoring and climate modeling, ultimately refining our predictive capabilities.
Here's how real-time data application transforms atmospheric research:
- Enhanced Accuracy: Real-time data ensures our models are continuously updated with the latest observations, reducing discrepancies and enhancing the precision of weather forecasts. This constant stream of information allows for more accurate predictions and timely responses to weather events.
- Dynamic Adaptation: With real-time data, we can adapt our climate models on-the-fly, allowing us to account for sudden changes in atmospheric conditions. This flexibility is important for understanding and mitigating the impacts of extreme weather events, such as hurricanes or heatwaves.
- Proactive Decision-Making: Access to real-time data empowers us to make proactive decisions, rather than reactive ones. For instance, emergency services can be better prepared for imminent natural disasters, and agricultural sectors can adjust practices based on current weather trends.
The integration of real-time data into our atmospheric research not only improves our analytical capabilities but also aligns with our desire for freedom by providing the tools to anticipate and respond to environmental changes more effectively.
Frequently Asked Questions
How Can Data Analytics Improve Climate Change Mitigation Efforts?
We can leverage data analytics to enhance climate change mitigation by providing precise insights for policy implications and driving technology advancements. This approach empowers us to implement effective strategies while ensuring our freedoms remain intact.
What Are the Ethical Considerations in Atmospheric Data Analytics?
Sailing ethical considerations in atmospheric data analytics is like steering a ship through stormy seas. We must address privacy concerns and guarantee bias detection, balancing innovation with respect for individual freedoms and data integrity.
How Do You Ensure the Accuracy of Atmospheric Data Models?
We confirm the accuracy of atmospheric data models through rigorous model validation and data verification processes. By cross-referencing multiple data sources and employing statistical techniques, we maintain high standards and give users the freedom to trust our models.
What Are the Career Opportunities in Atmospheric Data Analytics?
We see strong job prospects in atmospheric data analytics. Skill development in machine learning, statistical analysis, and programming opens doors to roles in weather forecasting, climate modeling, and environmental consulting. Freedom to innovate is key.
How Can Data Analytics Be Used for Disaster Preparedness and Response?
We can use data analytics for disaster forecasting and real-time monitoring to predict extreme weather events, enabling timely evacuations and resource deployment. This empowers communities to act swiftly, minimizing impact and maximizing freedom of movement and safety.