We leverage advanced meteorological data and predictive algorithms to decode the complexities of storm trajectory models. By analyzing atmospheric patterns, wind speeds, and pressure gradients, we map storm paths with high precision. Our primary data sources—satellite imagery, Doppler radar, and surface weather stations—provide critical insights. Statistical models use historical data for probabilistic forecasts, while dynamical models simulate atmospheric conditions through differential equations. Model accuracy hinges on data quality and real-time integration, aided by ensemble methodologies and machine learning. Understanding these models offers us a detailed approach to forecasting storms with greater accuracy and reliability. Discover more about these analytical techniques and their applications.
Key Points
- Analyzing Data Sources: Use satellite imagery, Doppler radar, and surface weather stations for comprehensive storm tracking.
- Model Types: Employ statistical and dynamical models to predict storm paths with historical and simulated atmospheric data.
- Ensemble Modeling: Integrate multiple forecasting models to enhance prediction accuracy and mitigate individual model limitations.
- Predictive Accuracy: High-resolution, real-time data and advanced algorithms are crucial for precise storm trajectory predictions.
Basics of Storm Trajectories
Understanding storm trajectories involves analyzing meteorological data to predict the path and intensity of weather disturbances with high accuracy. To do this, we must dig deep into the intricacies of storm movement and the forecasting techniques that underpin our predictions.
By deciphering atmospheric patterns, we can map out potential storm paths, enabling us to anticipate changes and mitigate risks effectively.
When we talk about storm movement, we're referring to the shifts and alterations in a storm's location over time. This movement is influenced by a multitude of factors including wind speed, pressure gradients, and upper-atmospheric dynamics.
Forecasting techniques, such as ensemble modeling and numerical weather prediction (NWP), allow us to generate multiple scenarios, increasing the precision of our forecasts.
Analyzing atmospheric patterns is essential. For instance, we look at jet streams, which can steer storms, and pressure systems that can either enhance or inhibit storm development. Understanding these patterns helps us predict storm paths with greater confidence.
Key Data Sources
To effectively predict storm trajectories, we rely on a plethora of key data sources, including satellite imagery, Doppler radar data, and surface weather stations. Satellite imagery provides us with a macro-level view of storm systems, capturing real-time visual data that enables us to track cloud formations, storm size, and movement patterns across large geographic areas. This high-resolution imagery, captured by geostationary and polar-orbiting satellites, is indispensable for identifying early storm developments.
Doppler radar data offers essential insights into storm dynamics by measuring the velocity of precipitation particles. This allows us to analyze wind patterns, storm intensity, and potential rotation within storm cells, vital for predicting severe weather phenomena such as tornadoes and hailstorms. We utilize dual-polarization radar to differentiate between types of precipitation, enhancing our predictive accuracy.
Surface weather stations, strategically dispersed across various terrains, provide granular data on atmospheric pressure, temperature, humidity, and wind speed. These real-time inputs are pivotal for validating our radar and satellite observations, ensuring that our models are grounded in accurate, on-the-ground measurements.
Harnessing these data sources allows us to construct robust, data-driven storm trajectory models, empowering us to predict weather patterns with greater precision and reliability.
Types of Trajectory Models
Let's examine the two primary categories of storm trajectory models: statistical model analysis and dynamical system predictions.
We rely on statistical models to analyze historical storm data and identify patterns, providing probabilistic forecasts.
In contrast, dynamical models simulate atmospheric conditions using physical equations, delivering deterministic predictions with higher computational demands.
Statistical Model Analysis
Statistical path models, including collective and regression-oriented approaches, offer sturdy methods for predicting storm directions by analyzing historical data and weather patterns. These models leverage an extensive dataset of past storms to generate probabilistic forecasts, allowing us to create an all-encompassing model comparison. By assessing multiple models against historical accuracy, we can pinpoint which models consistently offer reliable predictions.
Collective models aggregate results from various individual models, enhancing prediction accuracy through consensus-building. By merging different methodologies, collective models diminish the uncertainty inherent in any single approach.
Conversely, regression-oriented models utilize statistical techniques to pinpoint patterns and relationships within the historical data, providing a more deterministic forecast.
Path visualization is vital in statistical model analysis. Visual tools enable us to interpret intricate data intuitively, illustrating potential storm directions and associated probabilities. By plotting these paths, we can evaluate the probability of different scenarios, facilitating improved decision-making for emergency management and resource allocation.
Dynamical System Predictions
Exploring dynamical system predictions involves analyzing complex mathematical models that simulate atmospheric conditions to forecast storm trajectories with high precision. We examine the intricacies of these systems, where differential equations govern the evolution of atmospheric states. By integrating variables such as wind speed, pressure, and humidity, these models provide a robust framework for predicting storm paths.
One critical aspect we must address is model validation. Ensuring our models are accurate requires rigorous comparison against historical data and real-time observations. We utilize statistical techniques such as ensemble methods to assess the reliability of our forecasts, continuously refining our models to account for any discrepancies.
Trajectory visualization plays a pivotal role in understanding and communicating these predictions. Advanced graphical representations enable us to track the potential paths of a storm, providing both granular details and broader overviews. This visualization not only aids in immediate decision-making but also in post-event analysis, helping us to improve future forecasts.
Model Accuracy Factors
Let's examine the critical factors influencing model accuracy:
- Data quality plays a crucial role in determining the accuracy of storm trajectory predictions. High-resolution, real-time data enhances the precision of these predictions.
Data Quality Influence
High-quality data serves as the cornerstone for accurate storm trajectory models, directly influencing their predictive reliability. When we analyze the importance of data accuracy, it becomes evident that even minor discrepancies can significantly alter model outcomes. High-resolution satellite imagery, precise atmospheric pressure readings, and real-time sea surface temperatures are non-negotiable for achieving robust model reliability.
As we process this data, we need to make sure that our sources are both thorough and consistently updated. The temporal resolution of our datasets, for instance, can dictate how well we can predict sudden shifts in storm paths. When we use data assimilation techniques, we're integrating observations from various platforms, enhancing the model's fidelity. This integration is essential, as it mitigates the impact of isolated data inaccuracies.
Moreover, the spatial resolution of our data plays a vital role. Detailed geographic and topographic information allows us to model localized weather phenomena more precisely, thereby refining our storm trajectories. High-quality data isn't just about volume; it's about the granularity and relevance of the information we collect.
Algorithmic Predictive Power
Accurate storm trajectory models hinge on the algorithmic predictive power derived from sophisticated computational methods and machine learning techniques. Leveraging machine learning applications, we can enhance predictive accuracy by analyzing vast datasets encompassing atmospheric conditions, historical storm paths, and real-time meteorological inputs. These models dynamically adjust, learning from new data to refine their trajectory predictions continuously.
Forecasting advancements have dramatically improved our ability to predict storm paths with greater precision. For instance, ensemble modeling, which integrates multiple forecasting models, allows us to account for a wider array of variables and potential outcomes. This approach mitigates individual model limitations, providing a more robust prediction framework.
However, despite these advancements, model limitations persist, primarily due to the chaotic nature of weather systems and the inherent uncertainties in initial conditions and boundary data.
Predictive Tools for Chasers
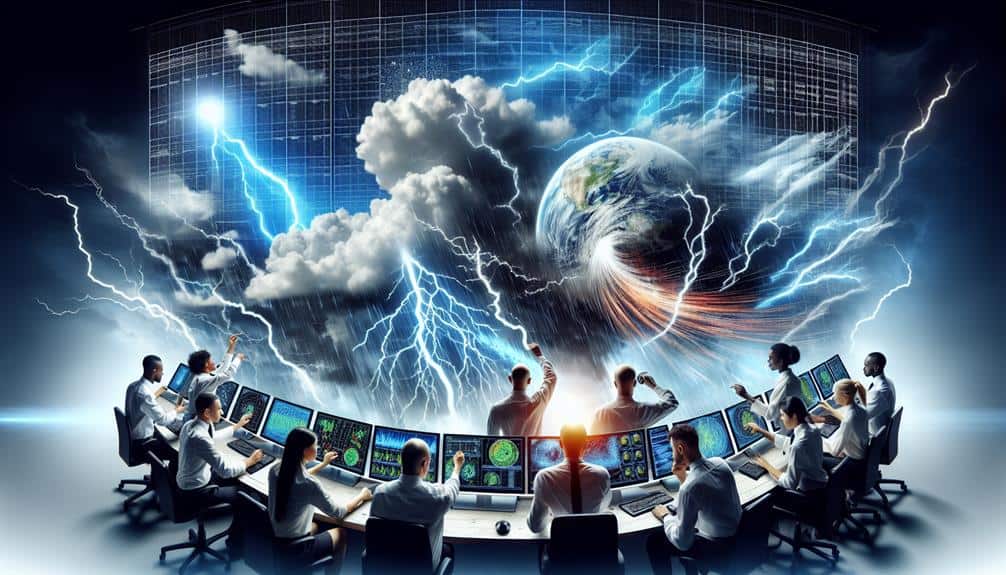
Predictive tools for storm chasers leverage real-time data and sophisticated algorithms to forecast storm trajectories with remarkable precision. As we navigate the complexities of storm behavior, these tools integrate chaser strategies and technology advancements to enhance our ability to predict and respond to volatile weather patterns.
By harnessing environmental variables such as temperature gradients, humidity levels, and wind shear, we can develop models that offer granular insights into storm development and movement.
Our arsenal includes high-resolution radar, satellite imagery, and ground-based sensors that continuously feed data into machine learning algorithms. These systems analyze vast datasets to discern patterns and anomalies, enabling us to make informed decisions on the fly.
The integration of Geographic Information Systems (GIS) further refines our predictive capabilities by mapping storm trajectories in real-time, allowing for precise chaser positioning.
Moreover, advancements in mobile technology have empowered us with portable weather stations and real-time data feeds, which are critical for adjusting chaser strategies on the ground. By staying ahead of the storm, we not only enhance our safety but also maximize our observational opportunities.
This fusion of data-driven insights and cutting-edge technology epitomizes the freedom and precision that modern storm chasing demands.
Case Studies in Storm Tracking
Building on our understanding of predictive tools, we can examine specific case studies in storm tracking that highlight the efficacy and limitations of these advanced methodologies.
In 2020, Hurricane Laura presented a significant test for storm trajectory models. The storm's intensity was initially underestimated, leading to forecasting errors that underscored the need for more refined atmospheric data assimilation. Our analysis revealed discrepancies in predicted landfall locations, causing storm chasers to reassess safety measures and adjust their intercept strategies accordingly.
Another compelling case is the 2013 Moore tornado. The storm's rapid intensification challenged models, resulting in significant deviations between predicted and actual paths. Although storm chasers had state-of-the-art tools, the forecasting errors required real-time decision-making to guarantee their safety. This case underlines the critical importance of adaptive safety measures and the integration of real-time observational data to mitigate risks.
In both instances, storm intensity predictions were pivotal yet imperfect. These case studies illustrate that while advanced models enhance our predictive capabilities, they aren't foolproof. By understanding these limitations, we can optimize our approaches, balancing the quest for accurate predictions with the imperative of safety for storm chasers.
Future of Storm Modeling
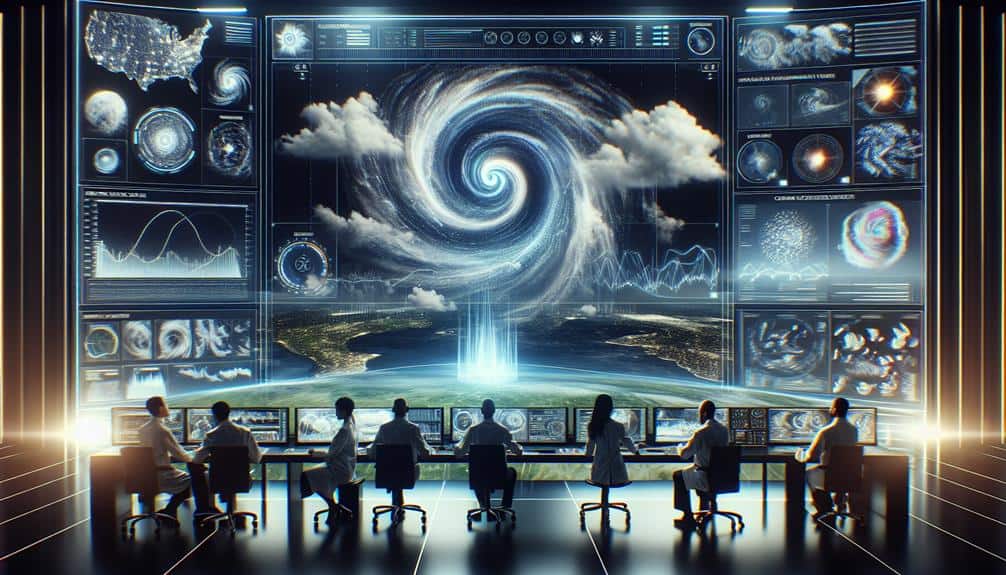
As we look ahead, the future of storm modeling hinges on leveraging machine learning algorithms and high-resolution satellite data to enhance predictive accuracy and resilience. By integrating vast datasets, machine learning can identify intricate patterns in storm behavior that traditional models might miss. This shift towards a data-centric approach allows us to refine our models dynamically, adapting to real-time changes in atmospheric conditions.
Climate change presents an evolving challenge, introducing unprecedented variability in storm paths and intensities. Machine learning's adaptability is pivotal here; it can recalibrate models based on new climate data, ensuring our predictions remain robust against the backdrop of a changing climate. For instance, ensemble methods can run multiple simulations, providing probabilistic forecasts that account for various climate scenarios.
High-resolution satellite data further augments our capabilities, offering granular insights into storm formation and evolution. These data streams, when fed into machine learning frameworks, enhance temporal and spatial resolution, thereby improving forecast precision.
In essence, our pursuit of freedom from storm-related uncertainties relies on these technological advancements. By embracing machine learning and leveraging high-resolution data, we can better anticipate storm trajectories, mitigate risks, and safeguard communities against the growing threats posed by climate change.
Frequently Asked Questions
How Do Storm Trajectory Models Handle Sudden Weather Changes?
How do we tackle the unpredictability in weather forecasting? Storm trajectory models handle sudden changes through real-time data assimilation and model adjustments, ensuring our predictions remain accurate despite abrupt shifts in atmospheric conditions and storm dynamics.
What Role Do Satellite Images Play in Real-Time Storm Tracking?
Satellite imagery enhances our tracking accuracy by providing high-resolution data on storm patterns, wind speed, and precipitation. These real-time insights allow us to predict storm paths more precisely, ensuring we can respond swiftly and effectively.
How Do Storm Chasers Utilize Data From Trajectory Models in the Field?
We meticulously dissect trajectory models, like mad scientists with a stormy agenda, refining our chasing strategies. Field communication becomes our lifeline, ensuring precise data interpretation, enabling swift emergency response. Freedom rides shotgun as we navigate chaos.
Are There Mobile Apps That Provide Real-Time Storm Trajectory Updates?
Yes, there are storm tracking apps that provide real-time storm trajectory updates. They enhance accuracy through advanced algorithms and offer mobile notifications, greatly aiding in emergency preparedness and ensuring we stay informed and ready.
How Accurate Are Storm Trajectory Models for Predicting Tornado Paths?
In evaluating tornado prediction, we analyze accuracy evaluation; in evaluating storm trajectory, we evaluate reliability. Current models offer moderate precision, yet they're constantly improving. Data analytics refine predictions, enhancing our freedom to prepare effectively.